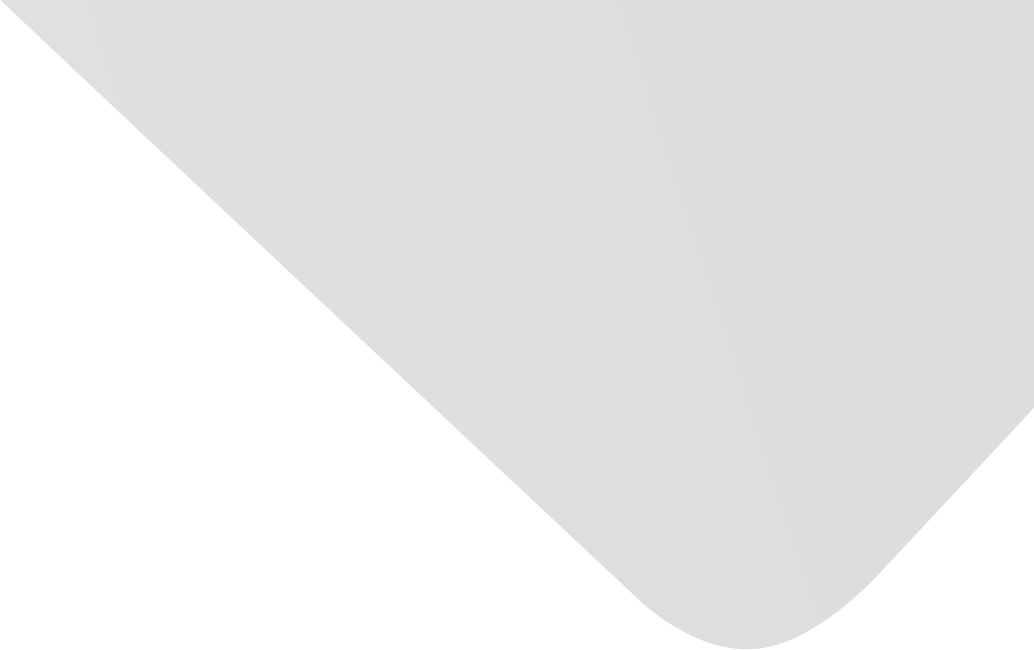
A Global-Relationship Dissimilarity Measure for the k-Modes Clustering Algorithm
Joint Authors
Zhou, Hongfang
Zhang, Yihui
Liu, Yibin
Source
Computational Intelligence and Neuroscience
Issue
Vol. 2017, Issue 2017 (31 Dec. 2017), pp.1-7, 7 p.
Publisher
Hindawi Publishing Corporation
Publication Date
2017-03-28
Country of Publication
Egypt
No. of Pages
7
Main Subjects
Abstract EN
The k-modes clustering algorithm has been widely used to cluster categorical data.
In this paper, we firstly analyzed the k-modes algorithm and its dissimilarity measure.
Based on this, we then proposed a novel dissimilarity measure, which is named as GRD.
GRD considers not only the relationships between the object and all cluster modes but also the differences of different attributes.
Finally the experiments were made on four real data sets from UCI.
And the corresponding results show that GRD achieves better performance than two existing dissimilarity measures used in k-modes and Cao’s algorithms.
American Psychological Association (APA)
Zhou, Hongfang& Zhang, Yihui& Liu, Yibin. 2017. A Global-Relationship Dissimilarity Measure for the k-Modes Clustering Algorithm. Computational Intelligence and Neuroscience،Vol. 2017, no. 2017, pp.1-7.
https://search.emarefa.net/detail/BIM-1140914
Modern Language Association (MLA)
Zhou, Hongfang…[et al.]. A Global-Relationship Dissimilarity Measure for the k-Modes Clustering Algorithm. Computational Intelligence and Neuroscience No. 2017 (2017), pp.1-7.
https://search.emarefa.net/detail/BIM-1140914
American Medical Association (AMA)
Zhou, Hongfang& Zhang, Yihui& Liu, Yibin. A Global-Relationship Dissimilarity Measure for the k-Modes Clustering Algorithm. Computational Intelligence and Neuroscience. 2017. Vol. 2017, no. 2017, pp.1-7.
https://search.emarefa.net/detail/BIM-1140914
Data Type
Journal Articles
Language
English
Notes
Includes bibliographical references
Record ID
BIM-1140914