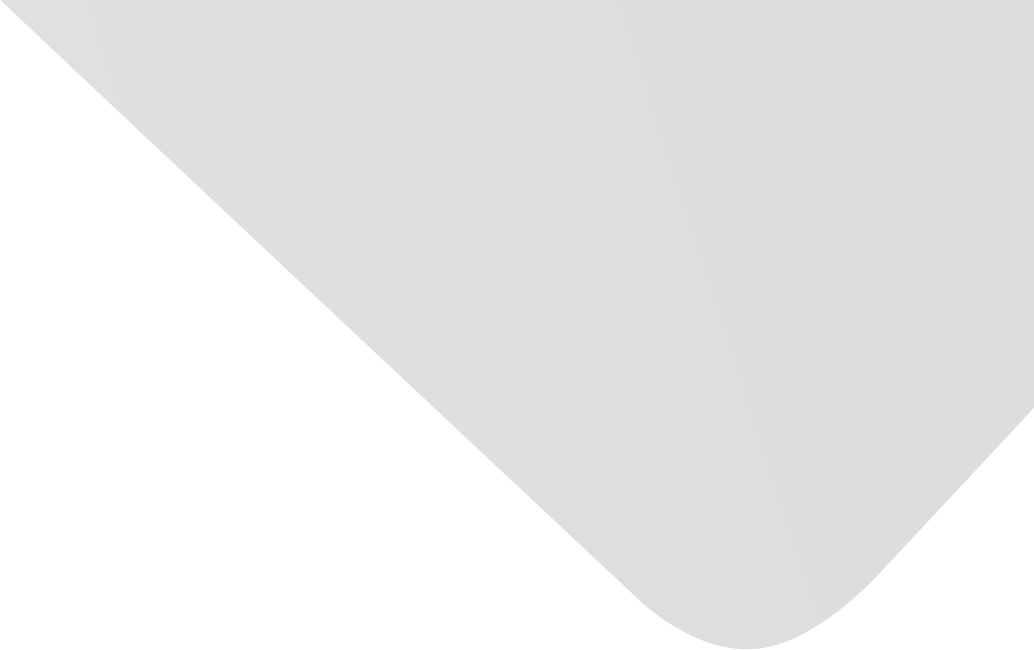
Ranking Support Vector Machine with Kernel Approximation
Joint Authors
Dou, Yong
Lv, Qi
Chen, Kai
Li, Rongchun
Liang, Zhengfa
Source
Computational Intelligence and Neuroscience
Issue
Vol. 2017, Issue 2017 (31 Dec. 2017), pp.1-9, 9 p.
Publisher
Hindawi Publishing Corporation
Publication Date
2017-02-13
Country of Publication
Egypt
No. of Pages
9
Main Subjects
Abstract EN
Learning to rank algorithm has become important in recent years due to its successful application in information retrieval, recommender system, and computational biology, and so forth.
Ranking support vector machine (RankSVM) is one of the state-of-art ranking models and has been favorably used.
Nonlinear RankSVM (RankSVM with nonlinear kernels) can give higher accuracy than linear RankSVM (RankSVM with a linear kernel) for complex nonlinear ranking problem.
However, the learning methods for nonlinear RankSVM are still time-consuming because of the calculation of kernel matrix.
In this paper, we propose a fast ranking algorithm based on kernel approximation to avoid computing the kernel matrix.
We explore two types of kernel approximation methods, namely, the Nyström method and random Fourier features.
Primal truncated Newton method is used to optimize the pairwise L2-loss (squared Hinge-loss) objective function of the ranking model after the nonlinear kernel approximation.
Experimental results demonstrate that our proposed method gets a much faster training speed than kernel RankSVM and achieves comparable or better performance over state-of-the-art ranking algorithms.
American Psychological Association (APA)
Chen, Kai& Li, Rongchun& Dou, Yong& Liang, Zhengfa& Lv, Qi. 2017. Ranking Support Vector Machine with Kernel Approximation. Computational Intelligence and Neuroscience،Vol. 2017, no. 2017, pp.1-9.
https://search.emarefa.net/detail/BIM-1140970
Modern Language Association (MLA)
Chen, Kai…[et al.]. Ranking Support Vector Machine with Kernel Approximation. Computational Intelligence and Neuroscience No. 2017 (2017), pp.1-9.
https://search.emarefa.net/detail/BIM-1140970
American Medical Association (AMA)
Chen, Kai& Li, Rongchun& Dou, Yong& Liang, Zhengfa& Lv, Qi. Ranking Support Vector Machine with Kernel Approximation. Computational Intelligence and Neuroscience. 2017. Vol. 2017, no. 2017, pp.1-9.
https://search.emarefa.net/detail/BIM-1140970
Data Type
Journal Articles
Language
English
Notes
Includes bibliographical references
Record ID
BIM-1140970