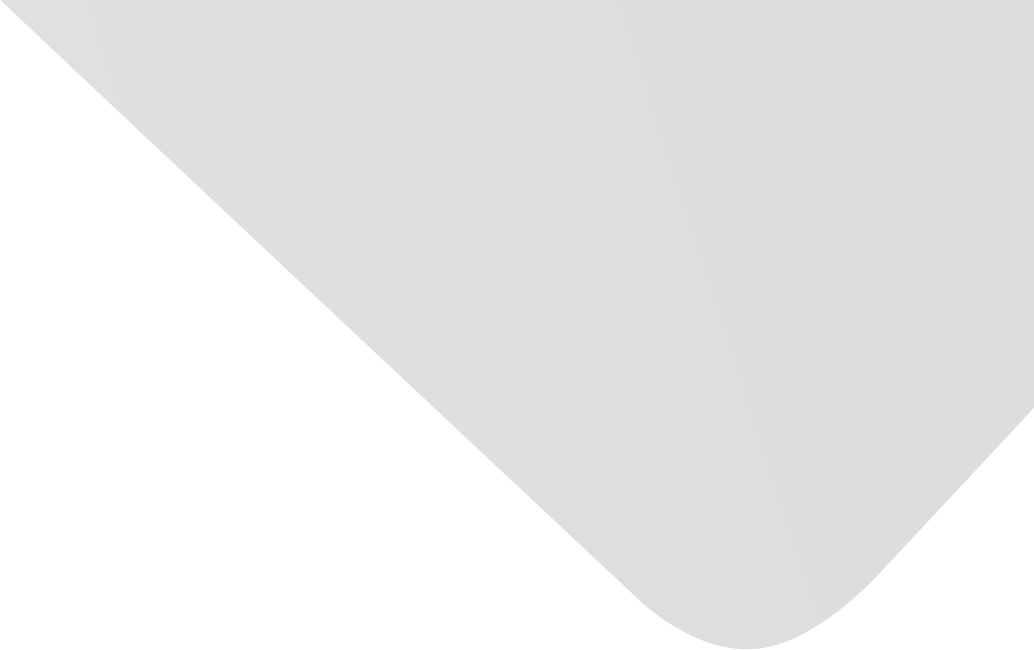
A Sparse Underdetermined Blind Source Separation Method and Its Application in Fault Diagnosis of Rotating Machinery
Joint Authors
Source
Issue
Vol. 2020, Issue 2020 (31 Dec. 2020), pp.1-17, 17 p.
Publisher
Hindawi Publishing Corporation
Publication Date
2020-07-23
Country of Publication
Egypt
No. of Pages
17
Main Subjects
Abstract EN
Rolling element bearing is one of the most commonly used supporting parts in rotating machinery, and it is also one of the most easily failing rotating parts.
It is of great safety and economic significance to study the effective fault diagnosis method of rolling element bearing.
The fault characteristic signal of rolling bearing is often affected by other interference signals in practical engineering, and the situation is much more serious when the rolling bearing fault occurs in gearbox.
Besides, only a limited number of measuring points are used in the process of rolling bearing fault signal acquisition due to the limitation of sensors installation condition.
In some sense, the above two factors often cause the result that the fault diagnosis of rolling bearing is the problem of underdetermined blind source separation.
The independence and non-Gaussian characteristic of the observed signals are the prerequisite of most of existent blind source separation methods.
Unlike traditional blind source separation methods, SCA originating from sparse representation is an effective method to solve the problem of underdetermined blind source separation, because it does not require the independence or non-Gaussian characteristics of the observed signals, and it only makes full use of the sparse characteristics of the observed signals to extract the source signal from the observed signals.
Based on these, a sparse component analysis (SCA) method based on linear clustering (LC) named LC-SCA is proposed for the purpose of underdetermined blind source separation of vibration signals of rolling element bearing, and the LC is introduced into SCA to improve the computation efficiency of SCA.
The effectiveness of the proposed method is verified by simulation and experiment.
In addition, the superiority of the method is verified by comparison with the other related methods such as constrained independent component analysis (cICA) and SCA.
American Psychological Association (APA)
Wang, HongChao& Du, WenLiao. 2020. A Sparse Underdetermined Blind Source Separation Method and Its Application in Fault Diagnosis of Rotating Machinery. Complexity،Vol. 2020, no. 2020, pp.1-17.
https://search.emarefa.net/detail/BIM-1140992
Modern Language Association (MLA)
Wang, HongChao& Du, WenLiao. A Sparse Underdetermined Blind Source Separation Method and Its Application in Fault Diagnosis of Rotating Machinery. Complexity No. 2020 (2020), pp.1-17.
https://search.emarefa.net/detail/BIM-1140992
American Medical Association (AMA)
Wang, HongChao& Du, WenLiao. A Sparse Underdetermined Blind Source Separation Method and Its Application in Fault Diagnosis of Rotating Machinery. Complexity. 2020. Vol. 2020, no. 2020, pp.1-17.
https://search.emarefa.net/detail/BIM-1140992
Data Type
Journal Articles
Language
English
Notes
Includes bibliographical references
Record ID
BIM-1140992