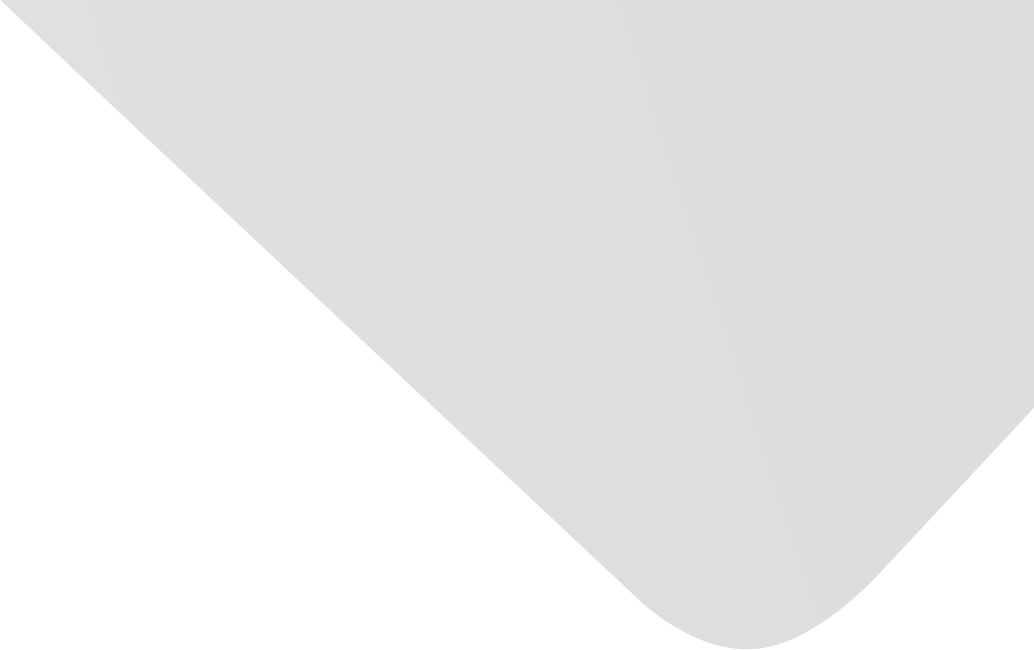
Displacement Prediction of a Complex Landslide in the Three Gorges Reservoir Area (China) Using a Hybrid Computational Intelligence Approach
Joint Authors
Tang, Huiming
Zhang, Junrong
Wang, Yankun
Ma, Junwei
Niu, Xiaoxu
Wen, Tao
Source
Issue
Vol. 2020, Issue 2020 (31 Dec. 2020), pp.1-15, 15 p.
Publisher
Hindawi Publishing Corporation
Publication Date
2020-01-28
Country of Publication
Egypt
No. of Pages
15
Main Subjects
Abstract EN
Displacement prediction of reservoir landslide remains inherently uncertain since a complete understanding of the complex nonlinear, dynamic landslide system is still lacking.
An appropriate quantification of predictive uncertainties is a key underpinning of displacement prediction and mitigation of reservoir landslide.
A density prediction, offering a full estimation of the probability density for future outputs, is promising for quantification of the uncertainty of landslide displacement.
In the present study, a hybrid computational intelligence approach is proposed to build a density prediction model of landslide displacement and quantify the associated predictive uncertainties.
The hybrid computational intelligence approach consists of two steps: first, the input variables are selected through copula analysis; second, kernel-based support vector machine quantile regression (KSVMQR) is employed to perform density prediction.
The copula-KSVMQR approach is demonstrated through a complex landslide in the Three Gorges Reservoir Area (TGRA), China.
The experimental study suggests that the copula-KSVMQR approach is capable of construction density prediction by providing full probability density distributions of the prediction with perfect performance.
In addition, different types of predictions, including interval prediction and point prediction, can be derived from the obtained density predictions with excellent performance.
The results show that the mean prediction interval widths of the proposed approach at ZG287 and ZG289 are 27.30 and 33.04, respectively, which are approximately 60 percent lower than that obtained using the traditional bootstrap-extreme learning machine-artificial neural network (Bootstrap-ELM-ANN).
Moreover, the obtained point predictions show great consistency with the observations, with correlation coefficients of 0.9998.
Given the satisfactory performance, the presented copula-KSVMQR approach shows a great ability to predict landslide displacement.
American Psychological Association (APA)
Ma, Junwei& Niu, Xiaoxu& Tang, Huiming& Wang, Yankun& Wen, Tao& Zhang, Junrong. 2020. Displacement Prediction of a Complex Landslide in the Three Gorges Reservoir Area (China) Using a Hybrid Computational Intelligence Approach. Complexity،Vol. 2020, no. 2020, pp.1-15.
https://search.emarefa.net/detail/BIM-1141116
Modern Language Association (MLA)
Ma, Junwei…[et al.]. Displacement Prediction of a Complex Landslide in the Three Gorges Reservoir Area (China) Using a Hybrid Computational Intelligence Approach. Complexity No. 2020 (2020), pp.1-15.
https://search.emarefa.net/detail/BIM-1141116
American Medical Association (AMA)
Ma, Junwei& Niu, Xiaoxu& Tang, Huiming& Wang, Yankun& Wen, Tao& Zhang, Junrong. Displacement Prediction of a Complex Landslide in the Three Gorges Reservoir Area (China) Using a Hybrid Computational Intelligence Approach. Complexity. 2020. Vol. 2020, no. 2020, pp.1-15.
https://search.emarefa.net/detail/BIM-1141116
Data Type
Journal Articles
Language
English
Notes
Includes bibliographical references
Record ID
BIM-1141116