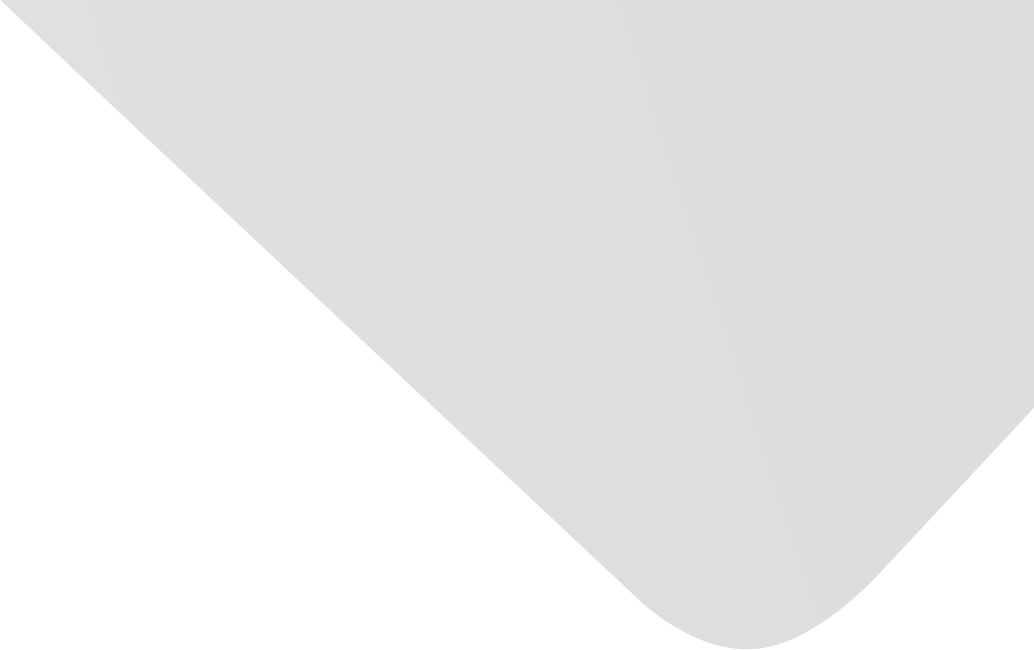
Temperature Forecasting via Convolutional Recurrent Neural Networks Based on Time-Series Data
Joint Authors
Source
Issue
Vol. 2020, Issue 2020 (31 Dec. 2020), pp.1-8, 8 p.
Publisher
Hindawi Publishing Corporation
Publication Date
2020-03-20
Country of Publication
Egypt
No. of Pages
8
Main Subjects
Abstract EN
Today, artificial intelligence and deep neural networks have been successfully used in many applications that have fundamentally changed people’s lives in many areas.
However, very limited research has been done in the meteorology area, where meteorological forecasts still rely on simulations via extensive computing resources.
In this paper, we propose an approach to using the neural network to forecast the future temperature according to the past temperature values.
Specifically, we design a convolutional recurrent neural network (CRNN) model that is composed of convolution neural network (CNN) portion and recurrent neural network (RNN) portion.
The model can learn the time correlation and space correlation of temperature changes from historical data through neural networks.
To evaluate the proposed CRNN model, we use the daily temperature data of mainland China from 1952 to 2018 as training data.
The results show that our model can predict future temperature with an error around 0.907°C.
American Psychological Association (APA)
Zhang, Zao& Dong, Yuan. 2020. Temperature Forecasting via Convolutional Recurrent Neural Networks Based on Time-Series Data. Complexity،Vol. 2020, no. 2020, pp.1-8.
https://search.emarefa.net/detail/BIM-1141490
Modern Language Association (MLA)
Zhang, Zao& Dong, Yuan. Temperature Forecasting via Convolutional Recurrent Neural Networks Based on Time-Series Data. Complexity No. 2020 (2020), pp.1-8.
https://search.emarefa.net/detail/BIM-1141490
American Medical Association (AMA)
Zhang, Zao& Dong, Yuan. Temperature Forecasting via Convolutional Recurrent Neural Networks Based on Time-Series Data. Complexity. 2020. Vol. 2020, no. 2020, pp.1-8.
https://search.emarefa.net/detail/BIM-1141490
Data Type
Journal Articles
Language
English
Notes
Includes bibliographical references
Record ID
BIM-1141490