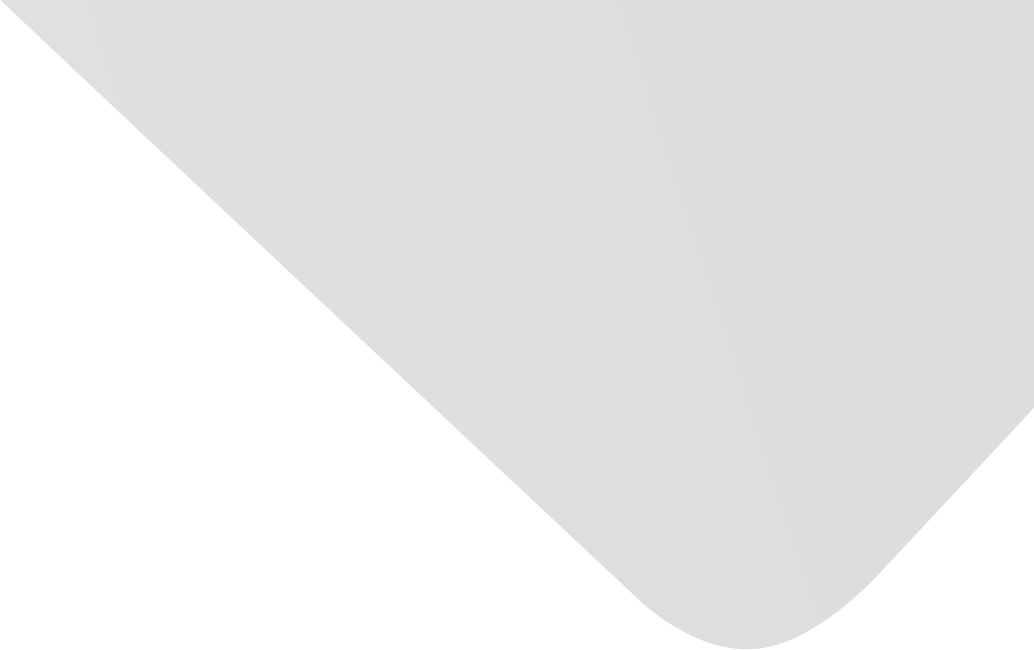
Joint Nonnegative Matrix Factorization Based on Sparse and Graph Laplacian Regularization for Clustering and Co-Differential Expression Genes Analysis
Joint Authors
Dai, Ling-Yun
Zhu, Rong
Wang, Juan
Source
Issue
Vol. 2020, Issue 2020 (31 Dec. 2020), pp.1-10, 10 p.
Publisher
Hindawi Publishing Corporation
Publication Date
2020-11-16
Country of Publication
Egypt
No. of Pages
10
Main Subjects
Abstract EN
The explosion of multiomics data poses new challenges to existing data mining methods.
Joint analysis of multiomics data can make the best of the complementary information that is provided by different types of data.
Therefore, they can more accurately explore the biological mechanism of diseases.
In this article, two forms of joint nonnegative matrix factorization based on the sparse and graph Laplacian regularization (SG-jNMF) method are proposed.
In the method, the graph regularization constraint can preserve the local geometric structure of data.
L2,1-norm regularization can enhance the sparsity among the rows and remove redundant features in the data.
First, SG-jNMF1 projects multiomics data into a common subspace and applies the multiomics fusion characteristic matrix to mine the important information closely related to diseases.
Second, multiomics data of the same disease are mapped into the common sample space by SG-jNMF2, and the cluster structures are detected clearly.
Experimental results show that SG-jNMF can achieve significant improvement in sample clustering compared with existing joint analysis frameworks.
SG-jNMF also effectively integrates multiomics data to identify co-differentially expressed genes (Co-DEGs).
SG-jNMF provides an efficient integrative analysis method for mining the biological information hidden in heterogeneous multiomics data.
American Psychological Association (APA)
Dai, Ling-Yun& Zhu, Rong& Wang, Juan. 2020. Joint Nonnegative Matrix Factorization Based on Sparse and Graph Laplacian Regularization for Clustering and Co-Differential Expression Genes Analysis. Complexity،Vol. 2020, no. 2020, pp.1-10.
https://search.emarefa.net/detail/BIM-1141744
Modern Language Association (MLA)
Dai, Ling-Yun…[et al.]. Joint Nonnegative Matrix Factorization Based on Sparse and Graph Laplacian Regularization for Clustering and Co-Differential Expression Genes Analysis. Complexity No. 2020 (2020), pp.1-10.
https://search.emarefa.net/detail/BIM-1141744
American Medical Association (AMA)
Dai, Ling-Yun& Zhu, Rong& Wang, Juan. Joint Nonnegative Matrix Factorization Based on Sparse and Graph Laplacian Regularization for Clustering and Co-Differential Expression Genes Analysis. Complexity. 2020. Vol. 2020, no. 2020, pp.1-10.
https://search.emarefa.net/detail/BIM-1141744
Data Type
Journal Articles
Language
English
Notes
Includes bibliographical references
Record ID
BIM-1141744