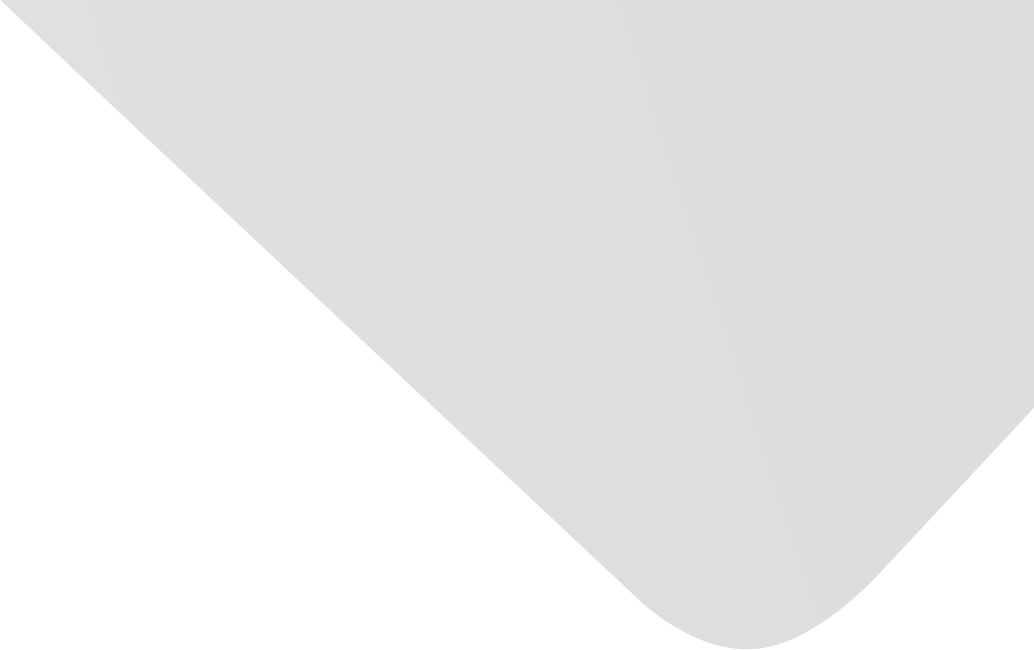
Fault Diagnosis of Rolling-Element Bearing Using Multiscale Pattern Gradient Spectrum Entropy Coupled with Laplacian Score
Joint Authors
Yan, Xiaoan
Liu, Ying
Ding, Peng
Jia, Minping
Source
Issue
Vol. 2020, Issue 2020 (31 Dec. 2020), pp.1-29, 29 p.
Publisher
Hindawi Publishing Corporation
Publication Date
2020-02-17
Country of Publication
Egypt
No. of Pages
29
Main Subjects
Abstract EN
Feature extraction is recognized as a critical stage in bearing fault diagnosis.
Pattern spectrum (PS) and pattern spectrum entropy (PSE) in recent years have been smoothly applied in feature extraction, whereas they easily ignore the partial impulse signatures hidden in bearing vibration data.
In this paper, the pattern gradient spectrum (PGS) and pattern gradient spectrum entropy (PGSE) are firstly presented to improve the performance of fault feature extraction of two approaches (PS and PSE).
Nonetheless, PSE and PGSE are only able to evaluate dynamic behavior of the time series on a single scale, which implies there is no consideration of feature information at other scales.
To address this problem, a novel approach entitled multiscale pattern gradient spectrum entropy (MPGSE) is further implemented to extract fault features across multiple scales, where its key parameters are determined adaptively by grey wolf optimization (GWO).
Meanwhile, a Laplacian score- (LS-) based feature selection strategy is employed to choose the sensitive features and establish a new feature set.
Finally, the selected new feature set is imported into extreme learning machine (ELM) to identify different health conditions of rolling bearing.
Performance of our designed algorithm is tested on two experimental cases.
Results confirm the availability of our proposed algorithm in feature extraction and show that our method can recognize effectively different bearing fault categories and severities.
More importantly, the designed approach can achieve higher recognition accuracies and provide better stability by comparing with other entropy-based methods involved in this paper.
American Psychological Association (APA)
Yan, Xiaoan& Liu, Ying& Ding, Peng& Jia, Minping. 2020. Fault Diagnosis of Rolling-Element Bearing Using Multiscale Pattern Gradient Spectrum Entropy Coupled with Laplacian Score. Complexity،Vol. 2020, no. 2020, pp.1-29.
https://search.emarefa.net/detail/BIM-1141761
Modern Language Association (MLA)
Yan, Xiaoan…[et al.]. Fault Diagnosis of Rolling-Element Bearing Using Multiscale Pattern Gradient Spectrum Entropy Coupled with Laplacian Score. Complexity No. 2020 (2020), pp.1-29.
https://search.emarefa.net/detail/BIM-1141761
American Medical Association (AMA)
Yan, Xiaoan& Liu, Ying& Ding, Peng& Jia, Minping. Fault Diagnosis of Rolling-Element Bearing Using Multiscale Pattern Gradient Spectrum Entropy Coupled with Laplacian Score. Complexity. 2020. Vol. 2020, no. 2020, pp.1-29.
https://search.emarefa.net/detail/BIM-1141761
Data Type
Journal Articles
Language
English
Notes
Includes bibliographical references
Record ID
BIM-1141761