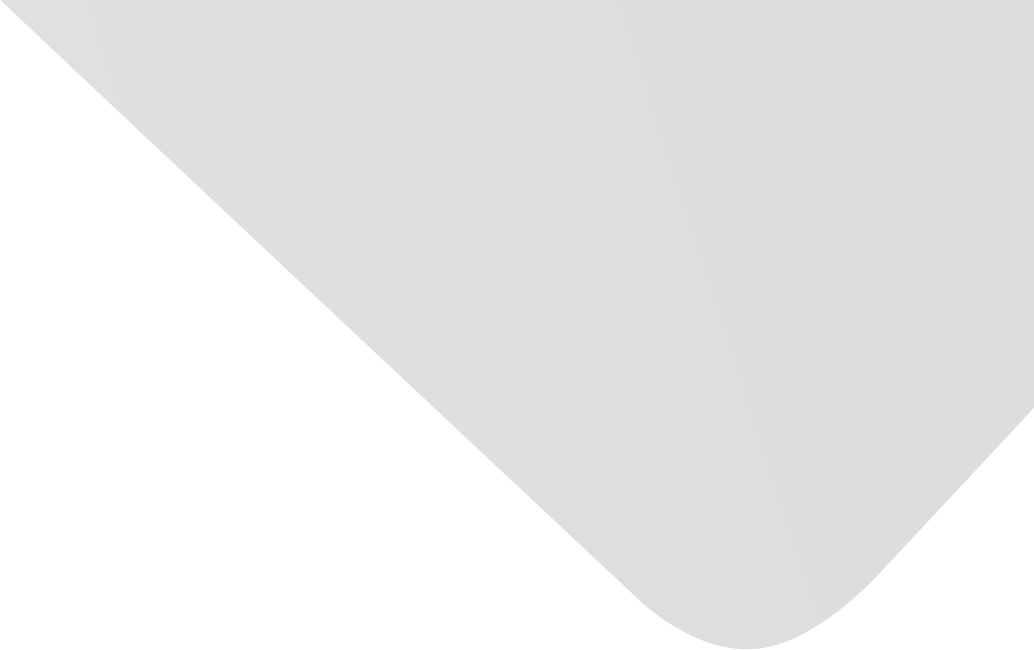
Articulatory-to-Acoustic Conversion Using BiLSTM-CNN Word-Attention-Based Method
Joint Authors
Ren, Guofeng
Shao, Guicheng
Fu, Jianmei
Source
Issue
Vol. 2020, Issue 2020 (31 Dec. 2020), pp.1-10, 10 p.
Publisher
Hindawi Publishing Corporation
Publication Date
2020-09-26
Country of Publication
Egypt
No. of Pages
10
Main Subjects
Abstract EN
In the recent years, along with the development of artificial intelligence (AI) and man-machine interaction technology, speech recognition and production have been asked to adapt to the rapid development of AI and man-machine technology, which need to improve recognition accuracy through adding novel features, fusing the feature, and improving recognition methods.
Aiming at developing novel recognition feature and application to speech recognition, this paper presents a new method for articulatory-to-acoustic conversion.
In the study, we have converted articulatory features (i.e., velocities of tongue and motion of lips) into acoustic features (i.e., the second formant and Mel-Cepstra).
By considering the graphical representation of the articulators’ motion, this study combined Bidirectional Long Short-Term Memory (BiLSTM) with convolution neural network (CNN) and adopted the idea of word attention in Mandarin to extract semantic features.
In this paper, we used the electromagnetic articulography (EMA) database designed by Taiyuan University of Technology, which contains ten speakers’ 299 disyllables and sentences of Mandarin, and extracted 8-dimensional articulatory features and 1-dimensional semantic feature relying on the word-attention layer; we then trained 200 samples and tested 99 samples for the articulatory-to-acoustic conversion.
Finally, Root Mean Square Error (RMSE), Mean Mel-Cepstral Distortion (MMCD), and correlation coefficient have been used to evaluate the conversion effect and for comparison with Gaussian Mixture Model (GMM) and BiLSTM of recurrent neural network (BiLSTM-RNN).
The results illustrated that the MMCD of Mel-Frequency Cepstrum Coefficient (MFCC) was 1.467 dB, and the RMSE of F2 was 22.10 Hz.
The research results of this study can be used in the features fusion and speech recognition to improve the accuracy of recognition.
American Psychological Association (APA)
Ren, Guofeng& Shao, Guicheng& Fu, Jianmei. 2020. Articulatory-to-Acoustic Conversion Using BiLSTM-CNN Word-Attention-Based Method. Complexity،Vol. 2020, no. 2020, pp.1-10.
https://search.emarefa.net/detail/BIM-1141898
Modern Language Association (MLA)
Ren, Guofeng…[et al.]. Articulatory-to-Acoustic Conversion Using BiLSTM-CNN Word-Attention-Based Method. Complexity No. 2020 (2020), pp.1-10.
https://search.emarefa.net/detail/BIM-1141898
American Medical Association (AMA)
Ren, Guofeng& Shao, Guicheng& Fu, Jianmei. Articulatory-to-Acoustic Conversion Using BiLSTM-CNN Word-Attention-Based Method. Complexity. 2020. Vol. 2020, no. 2020, pp.1-10.
https://search.emarefa.net/detail/BIM-1141898
Data Type
Journal Articles
Language
English
Notes
Includes bibliographical references
Record ID
BIM-1141898