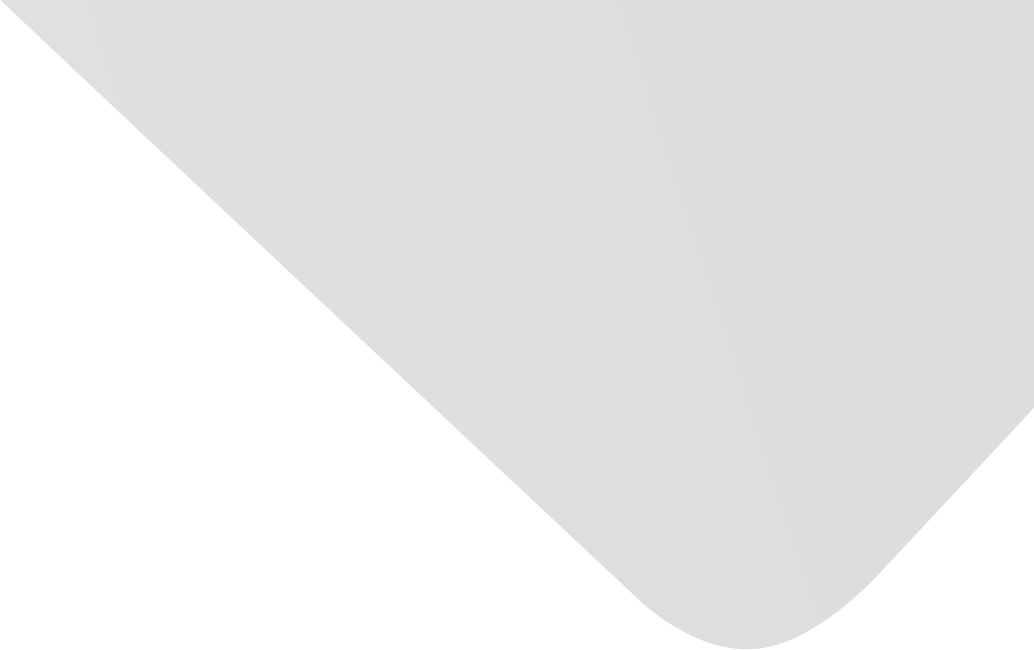
Vibration Tendency Prediction Approach for Hydropower Generator Fused with Multiscale Dominant Ingredient Chaotic Analysis, Adaptive Mutation Grey Wolf Optimizer, and KELM
Joint Authors
Fu, Wenlong
Tan, Jiawen
Wang, Kai
Shao, Kaixuan
Source
Issue
Vol. 2020, Issue 2020 (31 Dec. 2020), pp.1-20, 20 p.
Publisher
Hindawi Publishing Corporation
Publication Date
2020-02-12
Country of Publication
Egypt
No. of Pages
20
Main Subjects
Abstract EN
Accurate vibrational tendency forecasting of hydropower generator unit (HGU) is of great significance to guarantee the safe and economic operation of hydropower station.
For this purpose, a novel hybrid approach combined with multiscale dominant ingredient chaotic analysis, kernel extreme learning machine (KELM), and adaptive mutation grey wolf optimizer (AMGWO) is proposed.
Among the methods, variational mode decomposition (VMD), phase space reconstruction (PSR), and singular spectrum analysis (SSA) are suitably integrated into the proposed analysis strategy.
First of all, VMD is employed to decompose the monitored vibrational signal into several subseries with various frequency scales.
Then, SSA is applied to divide each decomposed subseries into dominant and residuary ingredients, after which an additional forecasting component is calculated by integrating the residual of VMD with all the residuary ingredients orderly.
Subsequently, the proposed AMGWO is implemented to simultaneously adapt the intrinsic parameters in PSR and KELM for all the forecasting components.
Ultimately, the prediction results of the raw vibration signal are obtained by assembling the results of all the predicted prediction components.
Furthermore, six relevant contrastive models are adopted to verify the feasibility and availability of the modified strategies employed in the proposed model.
The experimental results illustrate that (1) VMD plays a positive role for the prediction accuracy promotion; (2) the proposed dominant ingredient chaotic analysis based on the realization of time-frequency decomposition can further enhance the capability of the forecasting model; and (3) the appropriate parameters for each forecasting component can be optimized by the proposed AMGWO effectively, which can contribute to elevating the forecasting performance distinctly.
American Psychological Association (APA)
Fu, Wenlong& Wang, Kai& Tan, Jiawen& Shao, Kaixuan. 2020. Vibration Tendency Prediction Approach for Hydropower Generator Fused with Multiscale Dominant Ingredient Chaotic Analysis, Adaptive Mutation Grey Wolf Optimizer, and KELM. Complexity،Vol. 2020, no. 2020, pp.1-20.
https://search.emarefa.net/detail/BIM-1141926
Modern Language Association (MLA)
Fu, Wenlong…[et al.]. Vibration Tendency Prediction Approach for Hydropower Generator Fused with Multiscale Dominant Ingredient Chaotic Analysis, Adaptive Mutation Grey Wolf Optimizer, and KELM. Complexity No. 2020 (2020), pp.1-20.
https://search.emarefa.net/detail/BIM-1141926
American Medical Association (AMA)
Fu, Wenlong& Wang, Kai& Tan, Jiawen& Shao, Kaixuan. Vibration Tendency Prediction Approach for Hydropower Generator Fused with Multiscale Dominant Ingredient Chaotic Analysis, Adaptive Mutation Grey Wolf Optimizer, and KELM. Complexity. 2020. Vol. 2020, no. 2020, pp.1-20.
https://search.emarefa.net/detail/BIM-1141926
Data Type
Journal Articles
Language
English
Notes
Includes bibliographical references
Record ID
BIM-1141926