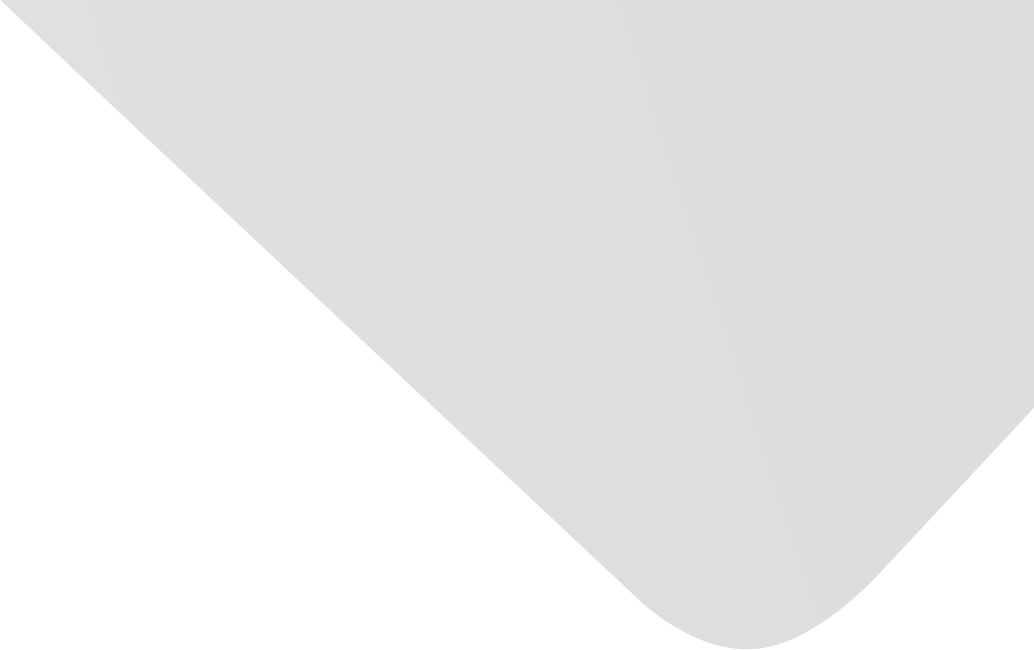
Data-Driven Bearing Fault Diagnosis of Microgrid Network Power Device Based on a Stacked Denoising Autoencoder in Deep Learning and Clustering by Fast Search without Data Labels
Joint Authors
Shu, Xin
Xu, Fan
Zhang, Xiaodi
Li, Xin
Source
Issue
Vol. 2020, Issue 2020 (31 Dec. 2020), pp.1-29, 29 p.
Publisher
Hindawi Publishing Corporation
Publication Date
2020-11-02
Country of Publication
Egypt
No. of Pages
29
Main Subjects
Abstract EN
The traditional health indicator (HI) construction method of electric equipment devices in microgrid networks, such as bearings that require different time-frequency domain indicators, needs several models to combine.
Therefore, it is necessary to manually select appropriate and sensitive models, such as time-frequency domain indicators and multimodel fusion, to build HIs in multiple steps, which is more complicated because sensitivity characteristics and suitable models are more representatives of bearing degradation trends.
In this paper, we use the stacked denoising autoencoder (SDAE) model in deep learning to construct HI directly from the microgrid power equipment of raw signals in bearings.
With this model, the HI can be constructed without multiple model combinations or the need for manual experience in selecting the sensitive indicators.
The SDAE can extract the representative degradation information adaptively from the original data through several nonlinear hidden layers automatically and approximate complicated nonlinear functions with a small reconstruction error.
After the SDAE extracts the preliminary HI, a model is needed to divide the wear state of the HI constructed by the SDAE.
A cluster model is commonly used for this, and unlike most clustering methods such as k-means, k-medoids, and fuzzy c-means (FCM), in which the clustering center point must be preset, cluster by fast search (CFS) can automatically find available cluster center points automatically according to the distance and local density between each point and its clustering center point.
Thus, the selected cluster center points are used to divide the wear state of the bearing.
The root mean square (RMS), kurtosis, Shannon entropy (SHE), approximate entropy (AE), permutation entropy (PE), and principal component analysis (PCA) are also used to construct the HI.
Finally, the results show that the performance of the method (SDAE-CFS) presented is superior to other combination HI models, such as EEMD-SVD-FCM/k-means/k-medoids, stacked autoencoder-CFS (SAE-CFS), RMS, kurtosis, SHE, AE, PE, and PCA.
American Psychological Association (APA)
Xu, Fan& Shu, Xin& Li, Xin& Zhang, Xiaodi. 2020. Data-Driven Bearing Fault Diagnosis of Microgrid Network Power Device Based on a Stacked Denoising Autoencoder in Deep Learning and Clustering by Fast Search without Data Labels. Complexity،Vol. 2020, no. 2020, pp.1-29.
https://search.emarefa.net/detail/BIM-1142193
Modern Language Association (MLA)
Xu, Fan…[et al.]. Data-Driven Bearing Fault Diagnosis of Microgrid Network Power Device Based on a Stacked Denoising Autoencoder in Deep Learning and Clustering by Fast Search without Data Labels. Complexity No. 2020 (2020), pp.1-29.
https://search.emarefa.net/detail/BIM-1142193
American Medical Association (AMA)
Xu, Fan& Shu, Xin& Li, Xin& Zhang, Xiaodi. Data-Driven Bearing Fault Diagnosis of Microgrid Network Power Device Based on a Stacked Denoising Autoencoder in Deep Learning and Clustering by Fast Search without Data Labels. Complexity. 2020. Vol. 2020, no. 2020, pp.1-29.
https://search.emarefa.net/detail/BIM-1142193
Data Type
Journal Articles
Language
English
Notes
Includes bibliographical references
Record ID
BIM-1142193