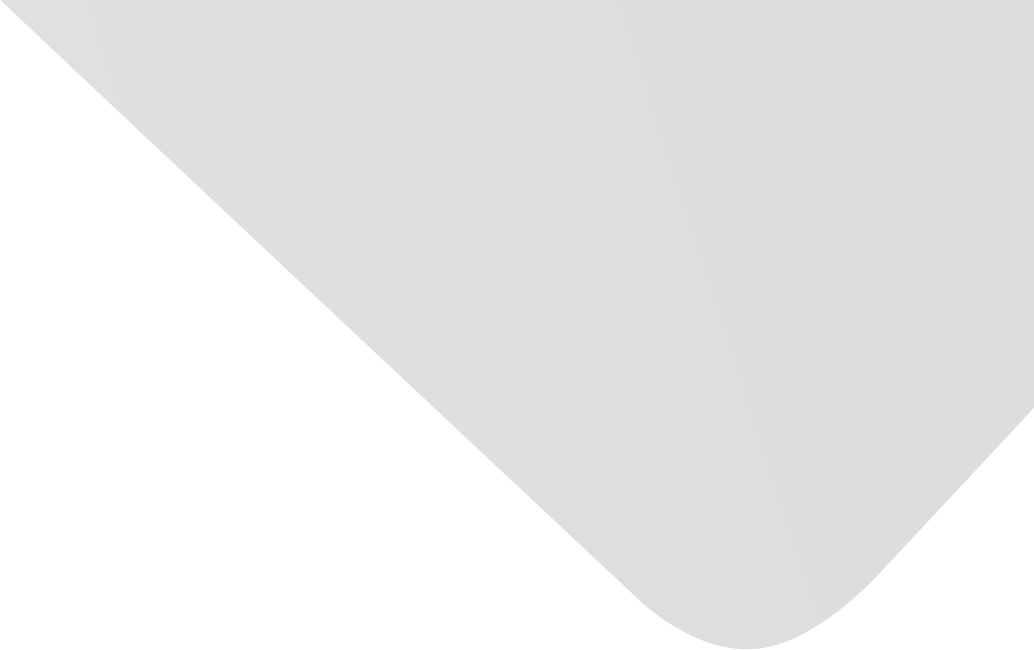
Pathway-Based Kernel Boosting for the Analysis of Genome-Wide Association Studies
Joint Authors
Wichmann, H. E.
Hofner, Benjamin
Friedrichs, Stefanie
Manitz, Juliane
Burger, Patricia
Amos, Christopher I.
Risch, Angela
Kneib, Thomas
Bickeböller, Heike
Chang-Claude, Jenny
Source
Computational and Mathematical Methods in Medicine
Issue
Vol. 2017, Issue 2017 (31 Dec. 2017), pp.1-17, 17 p.
Publisher
Hindawi Publishing Corporation
Publication Date
2017-07-13
Country of Publication
Egypt
No. of Pages
17
Main Subjects
Abstract EN
The analysis of genome-wide association studies (GWAS) benefits from the investigation of biologically meaningful gene sets, such as gene-interaction networks (pathways).
We propose an extension to a successful kernel-based pathway analysis approach by integrating kernel functions into a powerful algorithmic framework for variable selection, to enable investigation of multiple pathways simultaneously.
We employ genetic similarity kernels from the logistic kernel machine test (LKMT) as base-learners in a boosting algorithm.
A model to explain case-control status is created iteratively by selecting pathways that improve its prediction ability.
We evaluated our method in simulation studies adopting 50 pathways for different sample sizes and genetic effect strengths.
Additionally, we included an exemplary application of kernel boosting to a rheumatoid arthritis and a lung cancer dataset.
Simulations indicate that kernel boosting outperforms the LKMT in certain genetic scenarios.
Applications to GWAS data on rheumatoid arthritis and lung cancer resulted in sparse models which were based on pathways interpretable in a clinical sense.
Kernel boosting is highly flexible in terms of considered variables and overcomes the problem of multiple testing.
Additionally, it enables the prediction of clinical outcomes.
Thus, kernel boosting constitutes a new, powerful tool in the analysis of GWAS data and towards the understanding of biological processes involved in disease susceptibility.
American Psychological Association (APA)
Friedrichs, Stefanie& Manitz, Juliane& Burger, Patricia& Amos, Christopher I.& Risch, Angela& Chang-Claude, Jenny…[et al.]. 2017. Pathway-Based Kernel Boosting for the Analysis of Genome-Wide Association Studies. Computational and Mathematical Methods in Medicine،Vol. 2017, no. 2017, pp.1-17.
https://search.emarefa.net/detail/BIM-1142257
Modern Language Association (MLA)
Friedrichs, Stefanie…[et al.]. Pathway-Based Kernel Boosting for the Analysis of Genome-Wide Association Studies. Computational and Mathematical Methods in Medicine No. 2017 (2017), pp.1-17.
https://search.emarefa.net/detail/BIM-1142257
American Medical Association (AMA)
Friedrichs, Stefanie& Manitz, Juliane& Burger, Patricia& Amos, Christopher I.& Risch, Angela& Chang-Claude, Jenny…[et al.]. Pathway-Based Kernel Boosting for the Analysis of Genome-Wide Association Studies. Computational and Mathematical Methods in Medicine. 2017. Vol. 2017, no. 2017, pp.1-17.
https://search.emarefa.net/detail/BIM-1142257
Data Type
Journal Articles
Language
English
Notes
Includes bibliographical references
Record ID
BIM-1142257