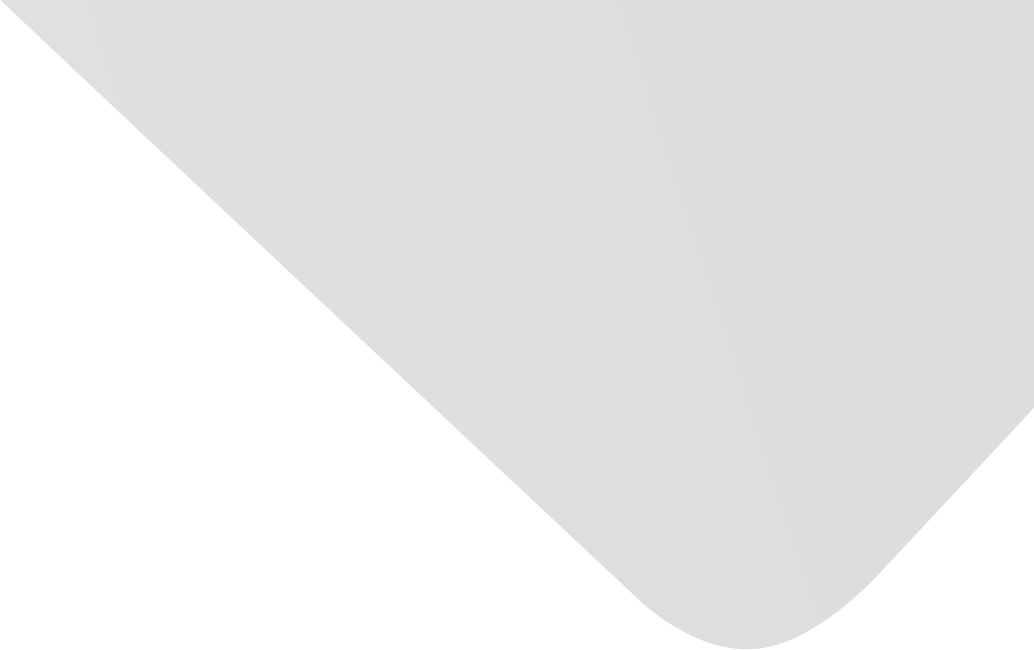
Correcting Classifiers for Sample Selection Bias in Two-Phase Case-Control Studies
Joint Authors
Krautenbacher, Norbert
Fuchs, Christiane
Theis, Fabian J.
Source
Computational and Mathematical Methods in Medicine
Issue
Vol. 2017, Issue 2017 (31 Dec. 2017), pp.1-18, 18 p.
Publisher
Hindawi Publishing Corporation
Publication Date
2017-09-24
Country of Publication
Egypt
No. of Pages
18
Main Subjects
Abstract EN
Epidemiological studies often utilize stratified data in which rare outcomes or exposures are artificially enriched.
This design can increase precision in association tests but distorts predictions when applying classifiers on nonstratified data.
Several methods correct for this so-called sample selection bias, but their performance remains unclear especially for machine learning classifiers.
With an emphasis on two-phase case-control studies, we aim to assess which corrections to perform in which setting and to obtain methods suitable for machine learning techniques, especially the random forest.
We propose two new resampling-based methods to resemble the original data and covariance structure: stochastic inverse-probability oversampling and parametric inverse-probability bagging.
We compare all techniques for the random forest and other classifiers, both theoretically and on simulated and real data.
Empirical results show that the random forest profits from only the parametric inverse-probability bagging proposed by us.
For other classifiers, correction is mostly advantageous, and methods perform uniformly.
We discuss consequences of inappropriate distribution assumptions and reason for different behaviors between the random forest and other classifiers.
In conclusion, we provide guidance for choosing correction methods when training classifiers on biased samples.
For random forests, our method outperforms state-of-the-art procedures if distribution assumptions are roughly fulfilled.
We provide our implementation in the R package sambia.
American Psychological Association (APA)
Krautenbacher, Norbert& Theis, Fabian J.& Fuchs, Christiane. 2017. Correcting Classifiers for Sample Selection Bias in Two-Phase Case-Control Studies. Computational and Mathematical Methods in Medicine،Vol. 2017, no. 2017, pp.1-18.
https://search.emarefa.net/detail/BIM-1142323
Modern Language Association (MLA)
Krautenbacher, Norbert…[et al.]. Correcting Classifiers for Sample Selection Bias in Two-Phase Case-Control Studies. Computational and Mathematical Methods in Medicine No. 2017 (2017), pp.1-18.
https://search.emarefa.net/detail/BIM-1142323
American Medical Association (AMA)
Krautenbacher, Norbert& Theis, Fabian J.& Fuchs, Christiane. Correcting Classifiers for Sample Selection Bias in Two-Phase Case-Control Studies. Computational and Mathematical Methods in Medicine. 2017. Vol. 2017, no. 2017, pp.1-18.
https://search.emarefa.net/detail/BIM-1142323
Data Type
Journal Articles
Language
English
Notes
Includes bibliographical references
Record ID
BIM-1142323