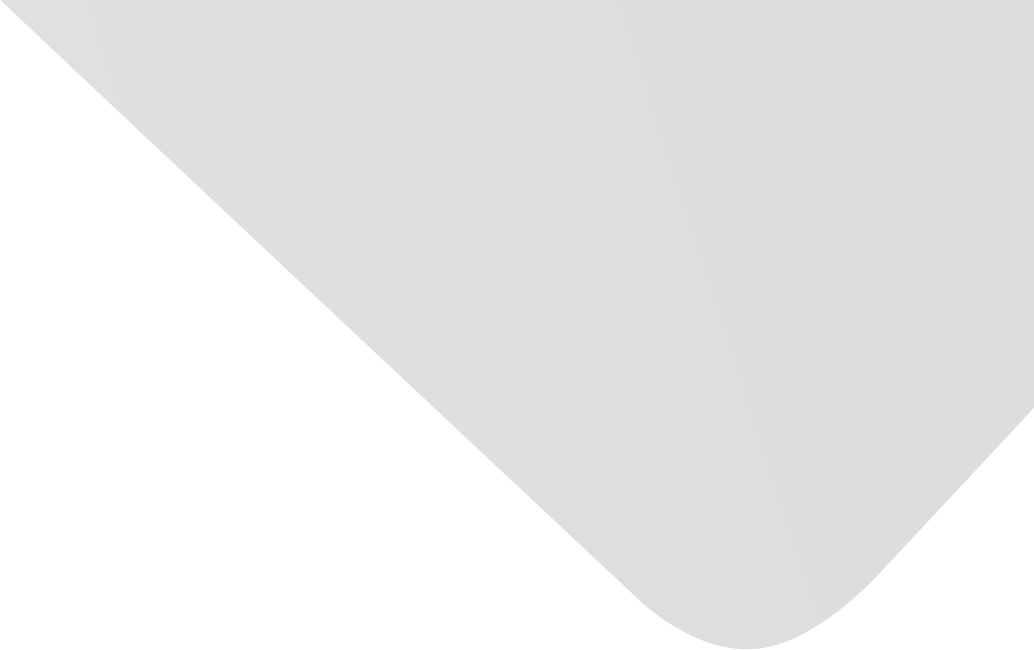
A Multi-Index Generative Adversarial Network for Tool Wear Detection with Imbalanced Data
Joint Authors
Zhang, Guokai
Xiao, Haoping
Jiang, Jingwen
Liu, Qinyuan
Liu, Yimo
Wang, Liying
Source
Issue
Vol. 2020, Issue 2020 (31 Dec. 2020), pp.1-10, 10 p.
Publisher
Hindawi Publishing Corporation
Publication Date
2020-12-07
Country of Publication
Egypt
No. of Pages
10
Main Subjects
Abstract EN
The scarcity of abnormal data leads to imbalanced data in the field of monitoring tool wear conditions.
In this paper, a novel multi-index generative adversarial network (MI-GAN) is proposed to detect the tool wear conditions subject to imbalanced signal data.
First, the generator in the MI-GAN is trained to produce fake normal signals, and the discriminator computes scores of testing signals and generated signals.
Next, the generator detects abnormal signals based on the performance of imitating testing signals, and the discriminator will compute the scores of testing signals and generated signals.
Subsequently, two indexes, i.e., L2-norm and temporal correlation coefficient (CORT), are put forward to measure the similarity between generated signals and testing signals.
Finally, our decision-making function further combines L2-norm and CORT with two discriminator scores to determine the tool conditions.
Experimental results show that our method obtains 97% accuracy in tool wear detection based on imbalanced data without manual feature extraction, which outperforms traditional machine learning methods.
American Psychological Association (APA)
Zhang, Guokai& Xiao, Haoping& Jiang, Jingwen& Liu, Qinyuan& Liu, Yimo& Wang, Liying. 2020. A Multi-Index Generative Adversarial Network for Tool Wear Detection with Imbalanced Data. Complexity،Vol. 2020, no. 2020, pp.1-10.
https://search.emarefa.net/detail/BIM-1142570
Modern Language Association (MLA)
Zhang, Guokai…[et al.]. A Multi-Index Generative Adversarial Network for Tool Wear Detection with Imbalanced Data. Complexity No. 2020 (2020), pp.1-10.
https://search.emarefa.net/detail/BIM-1142570
American Medical Association (AMA)
Zhang, Guokai& Xiao, Haoping& Jiang, Jingwen& Liu, Qinyuan& Liu, Yimo& Wang, Liying. A Multi-Index Generative Adversarial Network for Tool Wear Detection with Imbalanced Data. Complexity. 2020. Vol. 2020, no. 2020, pp.1-10.
https://search.emarefa.net/detail/BIM-1142570
Data Type
Journal Articles
Language
English
Notes
Includes bibliographical references
Record ID
BIM-1142570