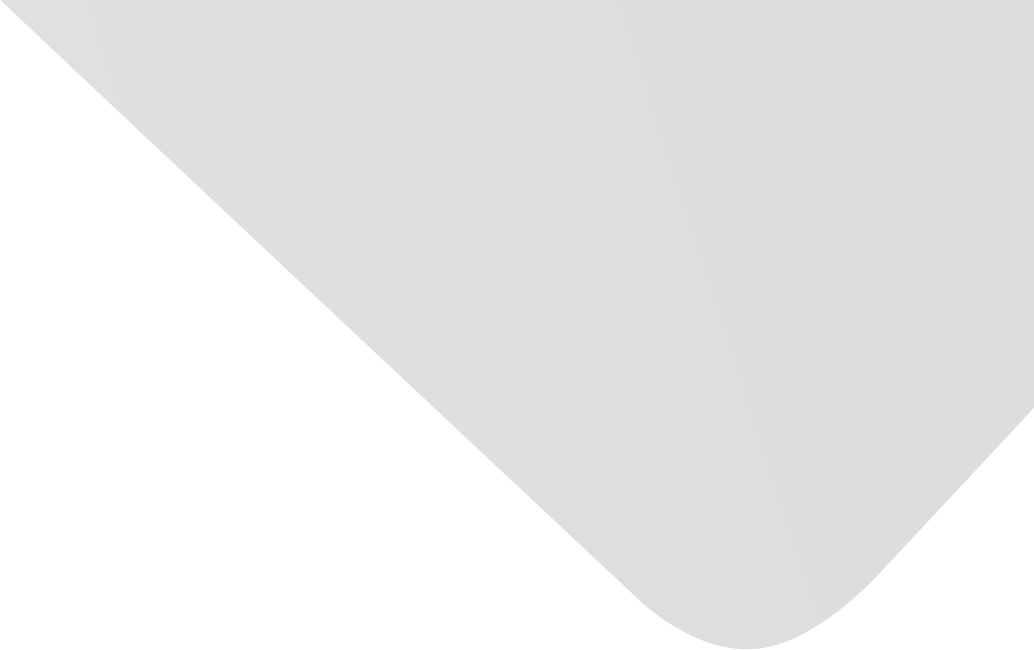
DLI: A Deep Learning-Based Granger Causality Inference
Author
Source
Issue
Vol. 2020, Issue 2020 (31 Dec. 2020), pp.1-6, 6 p.
Publisher
Hindawi Publishing Corporation
Publication Date
2020-06-09
Country of Publication
Egypt
No. of Pages
6
Main Subjects
Abstract EN
Integrating autoencoder (AE), long short-term memory (LSTM), and convolutional neural network (CNN), we propose an interpretable deep learning architecture for Granger causality inference, named deep learning-based Granger causality inference (DLI).
Two contributions of the proposed DLI are to reveal the Granger causality between the bitcoin price and S&P index and to forecast the bitcoin price and S&P index with a higher accuracy.
Experimental results demonstrate that there is a bidirectional but asymmetric Granger causality between the bitcoin price and S&P index.
And the DLI performs a superior prediction accuracy by integrating variables that have causalities with the target variable into the prediction process.
American Psychological Association (APA)
Peng, Wei. 2020. DLI: A Deep Learning-Based Granger Causality Inference. Complexity،Vol. 2020, no. 2020, pp.1-6.
https://search.emarefa.net/detail/BIM-1142641
Modern Language Association (MLA)
Peng, Wei. DLI: A Deep Learning-Based Granger Causality Inference. Complexity No. 2020 (2020), pp.1-6.
https://search.emarefa.net/detail/BIM-1142641
American Medical Association (AMA)
Peng, Wei. DLI: A Deep Learning-Based Granger Causality Inference. Complexity. 2020. Vol. 2020, no. 2020, pp.1-6.
https://search.emarefa.net/detail/BIM-1142641
Data Type
Journal Articles
Language
English
Notes
Includes bibliographical references
Record ID
BIM-1142641