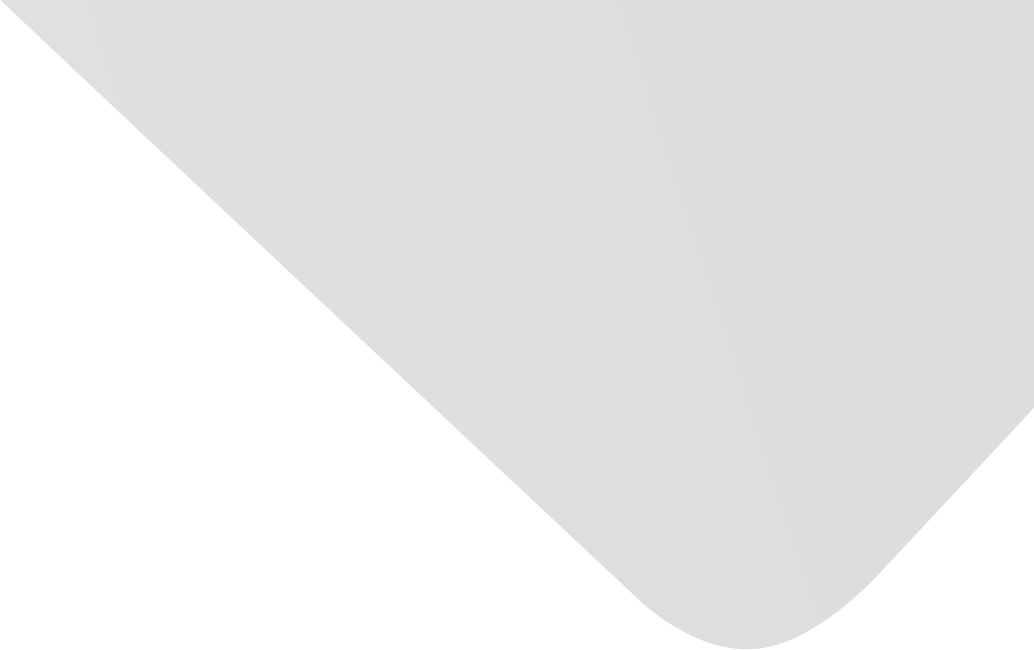
Attention with Long-Term Interval-Based Deep Sequential Learning for Recommendation
Joint Authors
Li, Zhao
Zhang, Long
Lei, Chenyi
Chen, Xia
Gao, Jianliang
Gao, Jun
Source
Issue
Vol. 2020, Issue 2020 (31 Dec. 2020), pp.1-13, 13 p.
Publisher
Hindawi Publishing Corporation
Publication Date
2020-07-13
Country of Publication
Egypt
No. of Pages
13
Main Subjects
Abstract EN
Modeling user behaviors as sequential learning provides key advantages in predicting future user actions, such as predicting the next product to purchase or the next song to listen to, for the purpose of personalized search and recommendation.
Traditional methods for modeling sequential user behaviors usually depend on the premise of Markov processes, while recently recurrent neural networks (RNNs) have been adopted to leverage their power in modeling sequences.
In this paper, we propose integrating attention mechanism into RNNs for better modeling sequential user behaviors.
Specifically, we design a network featuring Attention with Long-term Interval-based Gated Recurrent Units (ALI-GRU) to model temporal sequences of user actions.
Compared to previous works, our network can exploit the information of temporal dimension extracted by time interval-based GRU in addition to normal GRU to encoding user actions and has a specially designed matrix-form attention function to characterize both long-term preferences and short-term intents of users, while the attention-weighted features are finally decoded to predict the next user action.
We have performed experiments on two well-known public datasets as well as a huge dataset built from real-world data of one of the largest online shopping websites.
Experimental results show that the proposed ALI-GRU achieves significant improvement compared to state-of-the-art RNN-based methods.
ALI-GRU is also adopted in a real-world application and results of the online A/B test further demonstrate its practical value.
American Psychological Association (APA)
Li, Zhao& Zhang, Long& Lei, Chenyi& Chen, Xia& Gao, Jianliang& Gao, Jun. 2020. Attention with Long-Term Interval-Based Deep Sequential Learning for Recommendation. Complexity،Vol. 2020, no. 2020, pp.1-13.
https://search.emarefa.net/detail/BIM-1142740
Modern Language Association (MLA)
Li, Zhao…[et al.]. Attention with Long-Term Interval-Based Deep Sequential Learning for Recommendation. Complexity No. 2020 (2020), pp.1-13.
https://search.emarefa.net/detail/BIM-1142740
American Medical Association (AMA)
Li, Zhao& Zhang, Long& Lei, Chenyi& Chen, Xia& Gao, Jianliang& Gao, Jun. Attention with Long-Term Interval-Based Deep Sequential Learning for Recommendation. Complexity. 2020. Vol. 2020, no. 2020, pp.1-13.
https://search.emarefa.net/detail/BIM-1142740
Data Type
Journal Articles
Language
English
Notes
Includes bibliographical references
Record ID
BIM-1142740