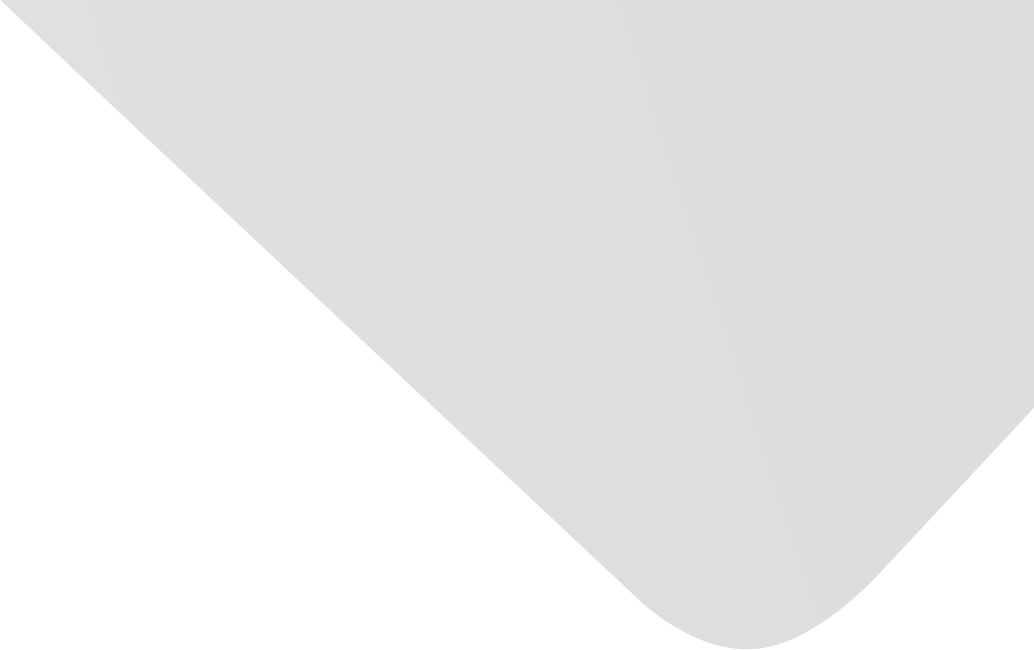
Robust Nonnegative Matrix Factorization via Joint Graph Laplacian and Discriminative Information for Identifying Differentially Expressed Genes
Joint Authors
Zheng, Chun-Hou
Liu, Jin-Xing
Hou, Mi-Xiao
Dai, Ling-Yun
Feng, Chun-Mei
Yu, Jiguo
Source
Issue
Vol. 2017, Issue 2017 (31 Dec. 2017), pp.1-11, 11 p.
Publisher
Hindawi Publishing Corporation
Publication Date
2017-04-06
Country of Publication
Egypt
No. of Pages
11
Main Subjects
Abstract EN
Differential expression plays an important role in cancer diagnosis and classification.
In recent years, many methods have been used to identify differentially expressed genes.
However, the recognition rate and reliability of gene selection still need to be improved.
In this paper, a novel constrained method named robust nonnegative matrix factorization via joint graph Laplacian and discriminative information (GLD-RNMF) is proposed for identifying differentially expressed genes, in which manifold learning and the discriminative label information are incorporated into the traditional nonnegative matrix factorization model to train the objective matrix.
Specifically, L2,1-norm minimization is enforced on both the error function and the regularization term which is robust to outliers and noise in gene data.
Furthermore, the multiplicative update rules and the details of convergence proof are shown for the new model.
The experimental results on two publicly available cancer datasets demonstrate that GLD-RNMF is an effective method for identifying differentially expressed genes.
American Psychological Association (APA)
Dai, Ling-Yun& Feng, Chun-Mei& Liu, Jin-Xing& Zheng, Chun-Hou& Yu, Jiguo& Hou, Mi-Xiao. 2017. Robust Nonnegative Matrix Factorization via Joint Graph Laplacian and Discriminative Information for Identifying Differentially Expressed Genes. Complexity،Vol. 2017, no. 2017, pp.1-11.
https://search.emarefa.net/detail/BIM-1142845
Modern Language Association (MLA)
Dai, Ling-Yun…[et al.]. Robust Nonnegative Matrix Factorization via Joint Graph Laplacian and Discriminative Information for Identifying Differentially Expressed Genes. Complexity No. 2017 (2017), pp.1-11.
https://search.emarefa.net/detail/BIM-1142845
American Medical Association (AMA)
Dai, Ling-Yun& Feng, Chun-Mei& Liu, Jin-Xing& Zheng, Chun-Hou& Yu, Jiguo& Hou, Mi-Xiao. Robust Nonnegative Matrix Factorization via Joint Graph Laplacian and Discriminative Information for Identifying Differentially Expressed Genes. Complexity. 2017. Vol. 2017, no. 2017, pp.1-11.
https://search.emarefa.net/detail/BIM-1142845
Data Type
Journal Articles
Language
English
Notes
Includes bibliographical references
Record ID
BIM-1142845