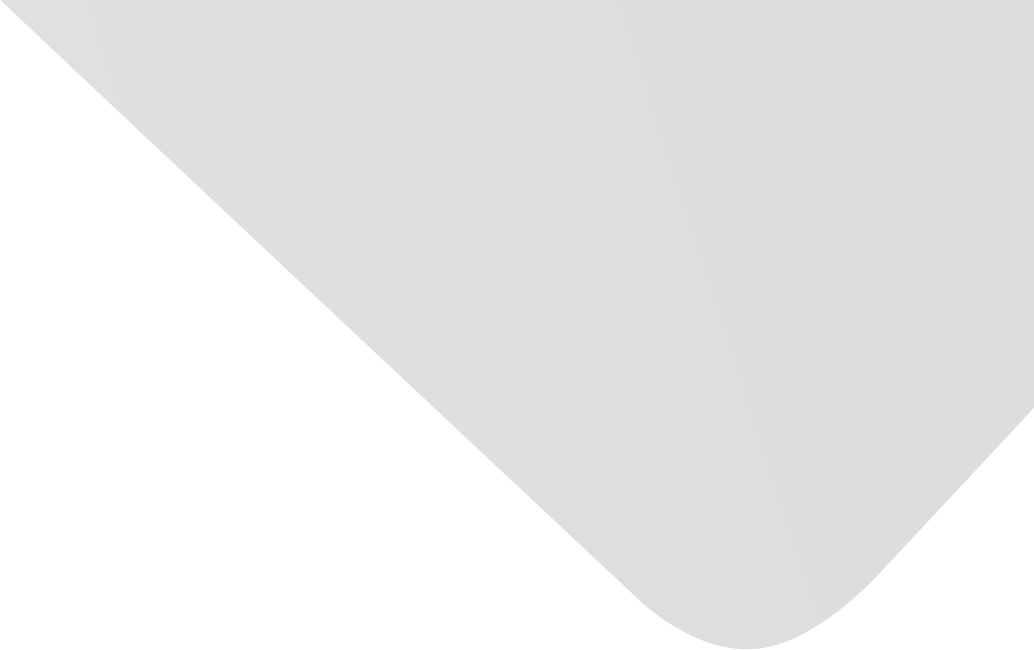
Intelligent Image Recognition System for Marine Fouling Using Softmax Transfer Learning and Deep Convolutional Neural Networks
Joint Authors
Chin, C. S.
Si, JianTing
Clare, A. S.
Ma, Maode
Source
Issue
Vol. 2017, Issue 2017 (31 Dec. 2017), pp.1-9, 9 p.
Publisher
Hindawi Publishing Corporation
Publication Date
2017-10-15
Country of Publication
Egypt
No. of Pages
9
Main Subjects
Abstract EN
The control of biofouling on marine vessels is challenging and costly.
Early detection before hull performance is significantly affected is desirable, especially if “grooming” is an option.
Here, a system is described to detect marine fouling at an early stage of development.
In this study, an image of fouling can be transferred wirelessly via a mobile network for analysis.
The proposed system utilizes transfer learning and deep convolutional neural network (CNN) to perform image recognition on the fouling image by classifying the detected fouling species and the density of fouling on the surface.
Transfer learning using Google’s Inception V3 model with Softmax at last layer was carried out on a fouling database of 10 categories and 1825 images.
Experimental results gave acceptable accuracies for fouling detection and recognition.
American Psychological Association (APA)
Chin, C. S.& Si, JianTing& Clare, A. S.& Ma, Maode. 2017. Intelligent Image Recognition System for Marine Fouling Using Softmax Transfer Learning and Deep Convolutional Neural Networks. Complexity،Vol. 2017, no. 2017, pp.1-9.
https://search.emarefa.net/detail/BIM-1143111
Modern Language Association (MLA)
Chin, C. S.…[et al.]. Intelligent Image Recognition System for Marine Fouling Using Softmax Transfer Learning and Deep Convolutional Neural Networks. Complexity No. 2017 (2017), pp.1-9.
https://search.emarefa.net/detail/BIM-1143111
American Medical Association (AMA)
Chin, C. S.& Si, JianTing& Clare, A. S.& Ma, Maode. Intelligent Image Recognition System for Marine Fouling Using Softmax Transfer Learning and Deep Convolutional Neural Networks. Complexity. 2017. Vol. 2017, no. 2017, pp.1-9.
https://search.emarefa.net/detail/BIM-1143111
Data Type
Journal Articles
Language
English
Notes
Includes bibliographical references
Record ID
BIM-1143111