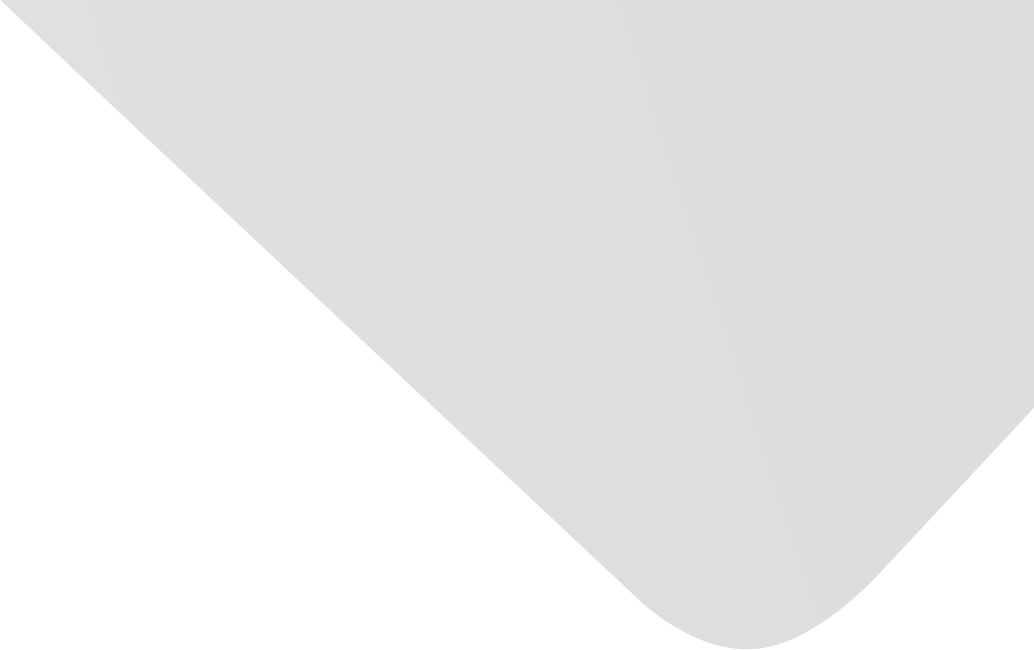
Adaptive Attention with Consumer Sentinel for Movie Box Office Prediction
Joint Authors
Source
Issue
Vol. 2020, Issue 2020 (31 Dec. 2020), pp.1-9, 9 p.
Publisher
Hindawi Publishing Corporation
Publication Date
2020-12-07
Country of Publication
Egypt
No. of Pages
9
Main Subjects
Abstract EN
To improve the movie box office prediction accuracy, this paper proposes an adaptive attention with consumer sentinel (LSTM-AACS) for movie box office prediction.
First, the influencing factors of the movie box office are analyzed.
Tackling the problem of ignoring consumer groups in existing prediction models, we add consumer features and then quantitatively analyze and normalize the box office influence factors.
Second, we establish an LSTM (Long Short-Term Memory) box office prediction model and inject the attention mechanism to construct an adaptive attention with consumer sentinel for movie box office prediction.
Finally, 10,398 pieces of movie box office dataset are used in the Kaggle competition to compare the prediction results with the LSTM-AACS model, LSTM-Attention model, and LSTM model.
The results show that the relative error of LSTM-AACS prediction is 6.58%, which is lower than other models used in the experiment.
American Psychological Association (APA)
Feng, Kaicheng& Liu, Xiaobing. 2020. Adaptive Attention with Consumer Sentinel for Movie Box Office Prediction. Complexity،Vol. 2020, no. 2020, pp.1-9.
https://search.emarefa.net/detail/BIM-1143254
Modern Language Association (MLA)
Feng, Kaicheng& Liu, Xiaobing. Adaptive Attention with Consumer Sentinel for Movie Box Office Prediction. Complexity No. 2020 (2020), pp.1-9.
https://search.emarefa.net/detail/BIM-1143254
American Medical Association (AMA)
Feng, Kaicheng& Liu, Xiaobing. Adaptive Attention with Consumer Sentinel for Movie Box Office Prediction. Complexity. 2020. Vol. 2020, no. 2020, pp.1-9.
https://search.emarefa.net/detail/BIM-1143254
Data Type
Journal Articles
Language
English
Notes
Includes bibliographical references
Record ID
BIM-1143254