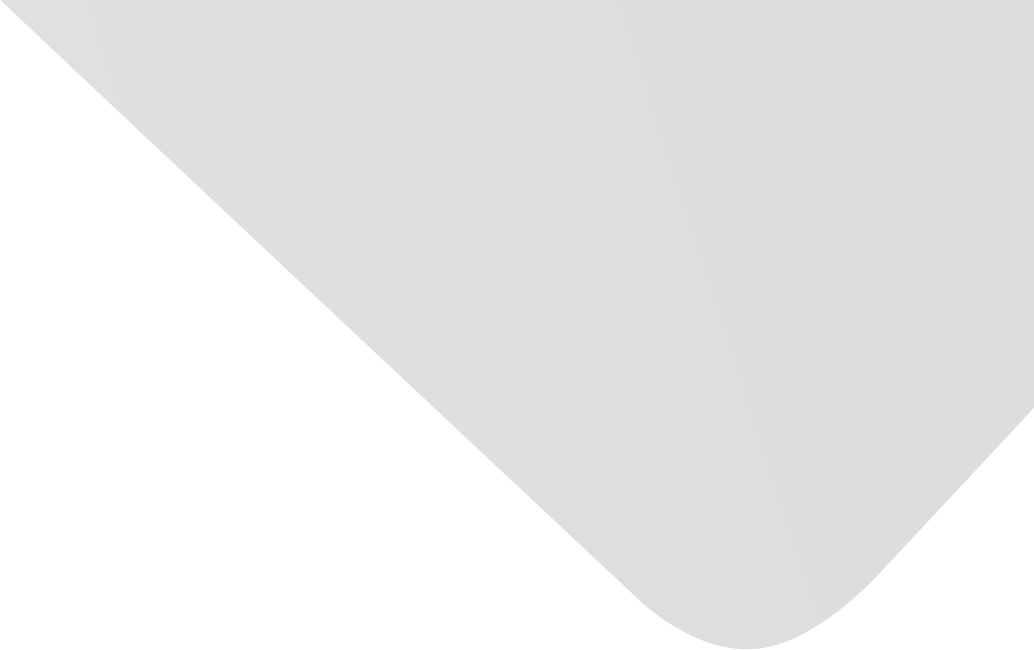
Decode Brain System: A Dynamic Adaptive Convolutional Quorum Voting Approach for Variable-Length EEG Data
Joint Authors
Xu, Tao
Zhou, Yun
Hou, Zekai
Zhang, Wenlan
Source
Issue
Vol. 2020, Issue 2020 (31 Dec. 2020), pp.1-9, 9 p.
Publisher
Hindawi Publishing Corporation
Publication Date
2020-03-28
Country of Publication
Egypt
No. of Pages
9
Main Subjects
Abstract EN
The brain is a complex and dynamic system, consisting of interacting sets and the temporal evolution of these sets.
Electroencephalogram (EEG) recordings of brain activity play a vital role to decode the cognitive process of human beings in learning research and application areas.
In the real world, people react to stimuli differently, and the duration of brain activities varies between individuals.
Therefore, the length of EEG recordings in trials gathered in the experiment is variable.
However, current approaches either fix the length of EEG recordings in each trial which would lose information hidden in the data or use the sliding window which would consume large computation on overlapped parts of slices.
In this paper, we propose TOO (Traverse Only Once), a new approach for processing variable-length EEG trial data.
TOO is a convolutional quorum voting approach that breaks the fixed structure of the model through convolutional implementation of sliding windows and the replacement of the fully connected layer by the 1 × 1 convolutional layer.
Each output cell generated from 1 × 1 convolutional layer corresponds to each slice created by a sliding time window, which reflects changes in cognitive states.
Then, TOO employs quorum voting on output cells and determines the cognitive state representing the entire single trial.
Our approach provides an adaptive model for trials of different lengths with traversing EEG data of each trial only once to recognize cognitive states.
We design and implement a cognitive experiment and obtain EEG data.
Using the data collecting from this experiment, we conducted an evaluation to compare TOO with a state-of-art sliding window end-to-end approach.
The results show that TOO yields a good accuracy (83.58%) at the trial level with a much lower computation (11.16%).
It also has the potential to be used in variable signal processing in other application areas.
American Psychological Association (APA)
Xu, Tao& Zhou, Yun& Hou, Zekai& Zhang, Wenlan. 2020. Decode Brain System: A Dynamic Adaptive Convolutional Quorum Voting Approach for Variable-Length EEG Data. Complexity،Vol. 2020, no. 2020, pp.1-9.
https://search.emarefa.net/detail/BIM-1143424
Modern Language Association (MLA)
Xu, Tao…[et al.]. Decode Brain System: A Dynamic Adaptive Convolutional Quorum Voting Approach for Variable-Length EEG Data. Complexity No. 2020 (2020), pp.1-9.
https://search.emarefa.net/detail/BIM-1143424
American Medical Association (AMA)
Xu, Tao& Zhou, Yun& Hou, Zekai& Zhang, Wenlan. Decode Brain System: A Dynamic Adaptive Convolutional Quorum Voting Approach for Variable-Length EEG Data. Complexity. 2020. Vol. 2020, no. 2020, pp.1-9.
https://search.emarefa.net/detail/BIM-1143424
Data Type
Journal Articles
Language
English
Notes
Includes bibliographical references
Record ID
BIM-1143424