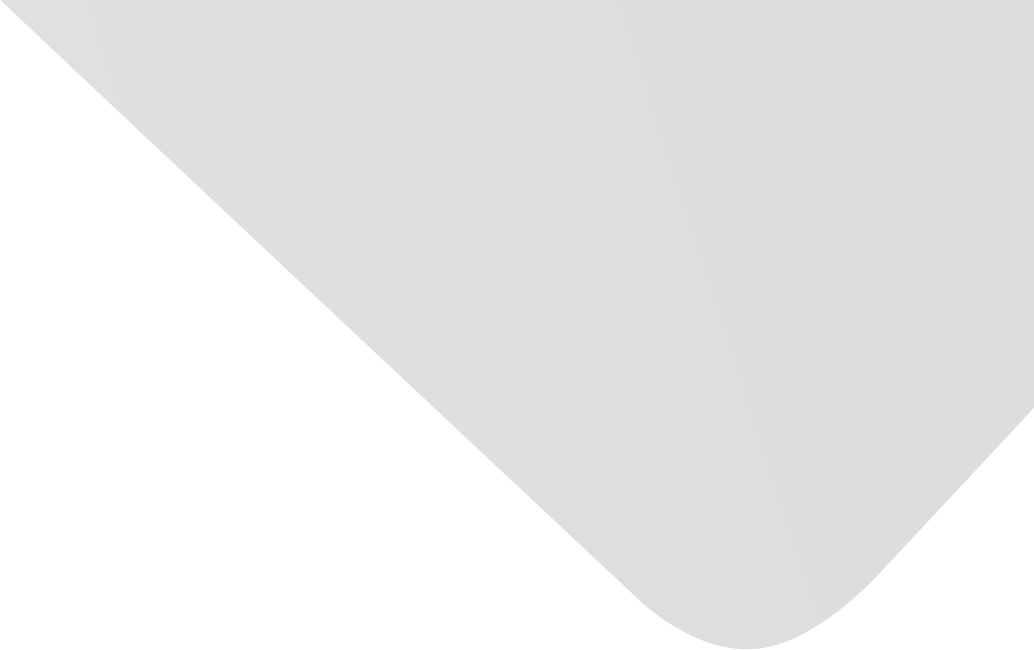
Nonlinear System Identification Using Quasi-ARX RBFN Models with a Parameter-Classified Scheme
Joint Authors
Wang, Lan
Hu, Jinglu
Dobaie, A.
Cheng, Yu
Liang, Jinling
Source
Issue
Vol. 2017, Issue 2017 (31 Dec. 2017), pp.1-12, 12 p.
Publisher
Hindawi Publishing Corporation
Publication Date
2017-12-11
Country of Publication
Egypt
No. of Pages
12
Main Subjects
Abstract EN
Quasi-linear autoregressive with exogenous inputs (Quasi-ARX) models have received considerable attention for their usefulness in nonlinear system identification and control.
In this paper, identification methods of quasi-ARX type models are reviewed and categorized in three main groups, and a two-step learning approach is proposed as an extension of the parameter-classified methods to identify the quasi-ARX radial basis function network (RBFN) model.
Firstly, a clustering method is utilized to provide statistical properties of the dataset for determining the parameters nonlinear to the model, which are interpreted meaningfully in the sense of interpolation parameters of a local linear model.
Secondly, support vector regression is used to estimate the parameters linear to the model; meanwhile, an explicit kernel mapping is given in terms of the nonlinear parameter identification procedure, in which the model is transformed from the nonlinear-in-nature to the linear-in-parameter.
Numerical and real cases are carried out finally to demonstrate the effectiveness and generalization ability of the proposed method.
American Psychological Association (APA)
Wang, Lan& Cheng, Yu& Hu, Jinglu& Liang, Jinling& Dobaie, A.. 2017. Nonlinear System Identification Using Quasi-ARX RBFN Models with a Parameter-Classified Scheme. Complexity،Vol. 2017, no. 2017, pp.1-12.
https://search.emarefa.net/detail/BIM-1143495
Modern Language Association (MLA)
Wang, Lan…[et al.]. Nonlinear System Identification Using Quasi-ARX RBFN Models with a Parameter-Classified Scheme. Complexity No. 2017 (2017), pp.1-12.
https://search.emarefa.net/detail/BIM-1143495
American Medical Association (AMA)
Wang, Lan& Cheng, Yu& Hu, Jinglu& Liang, Jinling& Dobaie, A.. Nonlinear System Identification Using Quasi-ARX RBFN Models with a Parameter-Classified Scheme. Complexity. 2017. Vol. 2017, no. 2017, pp.1-12.
https://search.emarefa.net/detail/BIM-1143495
Data Type
Journal Articles
Language
English
Notes
Includes bibliographical references
Record ID
BIM-1143495