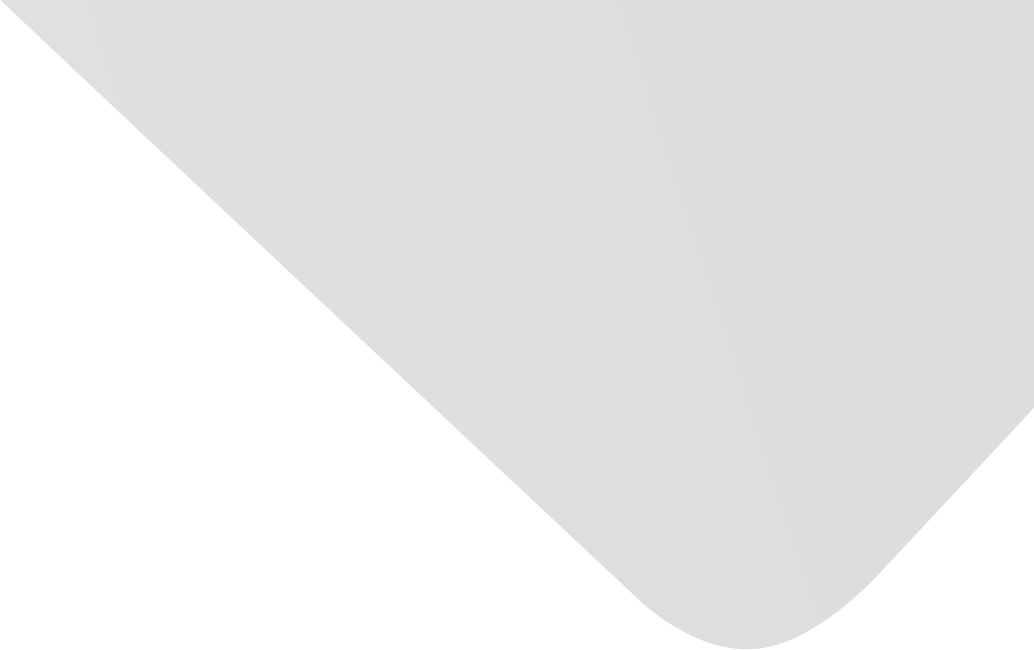
1D CNN-Based Intracranial Aneurysms Detection in 3D TOF-MRA
Joint Authors
Hou, Wenguang
Mei, Shaojie
Gui, Qiuling
Zou, Yingcheng
Wang, Yifan
Deng, Xianbo
Cheng, Qimin
Source
Issue
Vol. 2020, Issue 2020 (31 Dec. 2020), pp.1-13, 13 p.
Publisher
Hindawi Publishing Corporation
Publication Date
2020-11-12
Country of Publication
Egypt
No. of Pages
13
Main Subjects
Abstract EN
How to automatically detect intracranial aneurysms from Three-Dimension Time of Flight Magnetic Resonance Angiography (3D TOF MRA) images is a typical 3D image classification problem.
Currently, the commonly used method is the Maximum Intensity Projection- (MIP-) based way.
It transfers 3D classification into 2D case by projecting the 3D patch into 2D planes along different directions on the basis of voxel’s intensity.
After then, the 2D Convolutional Neural Network (CNN) is established to do classification.
It has been shown that the MIP-based method can reduce the demands for the samples and increase the computation efficiency.
Meanwhile, the accuracy is comparable with that of 3D image classification.
Inspired by the strategy of MIP, we want to further reduce the demands for samples and accelerate the training by transferring the 2D image classification into 1D case, i.e., we want to generate the 1D vectors from the MIP images and then establish a 1D CNN to do intracranial aneurysm detection and classification for 3D TOF MRA image.
Specifically, our method first extracts a series of patches as the Region of Interests (ROIs) along the blood vessels from the original 3D TOF MRA 3D image.
The corresponding MIP images of each ROI will be obtained through maximum intensity projecting.
Then, we generate a series of 1D vectors by accumulating each MIP image along different directions.
Meanwhile, a 1D CNN is established to detect aneurysms, in which, the input is the obtained 1D vectors and the output is the binary classification result denoting whether there are intracranial aneurysms in the considered patch.
Generally, compared with 2D- and 3D-CNN, the 1D CNN-based way greatly accelerates the training and shows stronger robustness in the case of fewer samples.
The efficiency of the proposed method outperforms the 2D CNN about 10 times in CPU training.
Yet, their accuracies are close.
American Psychological Association (APA)
Hou, Wenguang& Mei, Shaojie& Gui, Qiuling& Zou, Yingcheng& Wang, Yifan& Deng, Xianbo…[et al.]. 2020. 1D CNN-Based Intracranial Aneurysms Detection in 3D TOF-MRA. Complexity،Vol. 2020, no. 2020, pp.1-13.
https://search.emarefa.net/detail/BIM-1143504
Modern Language Association (MLA)
Hou, Wenguang…[et al.]. 1D CNN-Based Intracranial Aneurysms Detection in 3D TOF-MRA. Complexity No. 2020 (2020), pp.1-13.
https://search.emarefa.net/detail/BIM-1143504
American Medical Association (AMA)
Hou, Wenguang& Mei, Shaojie& Gui, Qiuling& Zou, Yingcheng& Wang, Yifan& Deng, Xianbo…[et al.]. 1D CNN-Based Intracranial Aneurysms Detection in 3D TOF-MRA. Complexity. 2020. Vol. 2020, no. 2020, pp.1-13.
https://search.emarefa.net/detail/BIM-1143504
Data Type
Journal Articles
Language
English
Notes
Includes bibliographical references
Record ID
BIM-1143504