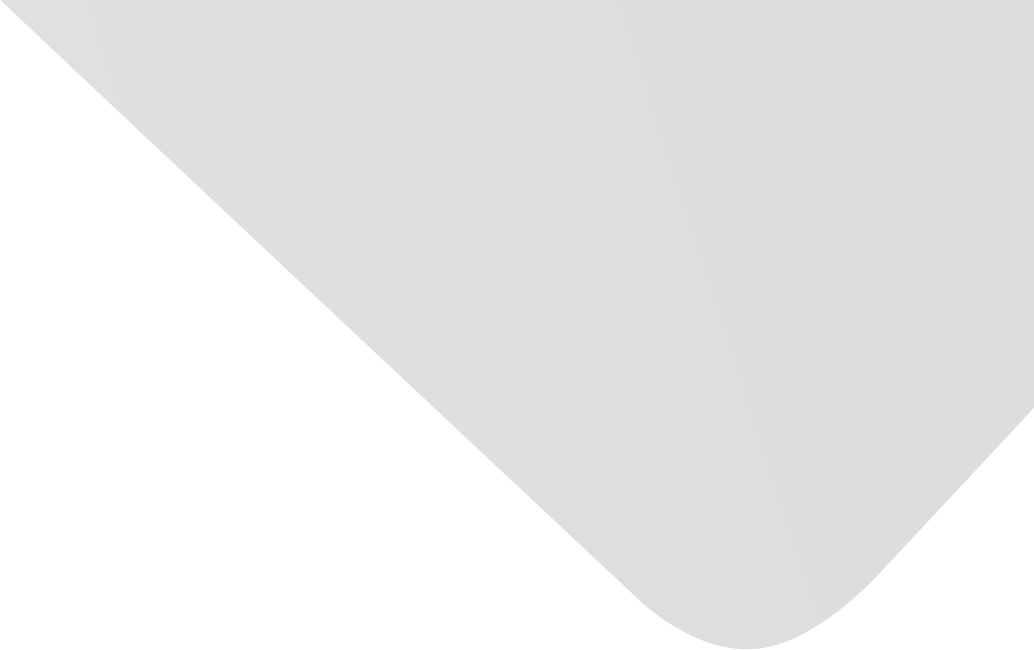
Nonlinear Structural Fusion for Multiplex Network
Joint Authors
Ning, Nianwen
Long, Feiyu
Wang, Chunchun
Zhang, Youjie
Yang, Yilin
Wu, Bin
Source
Issue
Vol. 2020, Issue 2020 (31 Dec. 2020), pp.1-17, 17 p.
Publisher
Hindawi Publishing Corporation
Publication Date
2020-07-14
Country of Publication
Egypt
No. of Pages
17
Main Subjects
Abstract EN
Many real-world complex systems have multiple types of relations between their components, and they are popularly modeled as multiplex networks with each type of relation as one layer.
Since the fusion analysis of multiplex networks can provide a comprehensive insight, the structural information fusion of multiplex networks has become a crucial issue.
However, most of these existing data fusion methods are inappropriate for researchers to apply to complex network analysis directly.
The feature-based fusion methods ignore the sharing and complementarity of interlayer structural information.
To tackle this problem, we propose a multiplex network structural fusion (MNSF) model, which can construct a network with comprehensive information.
It is composed of two modules: the network feature extraction (NFE) module and the network structural fusion (NSF) module.
(1) In NFE, MNSF first extracts a low-dimensional vector representation of a node from each layer.
Then, we construct a node similarity network based on embedding matrices and K-D tree algorithm.
(2) In NSF, we present a nonlinear enhanced iterative fusion (EIF) strategy.
EIF can strengthen high-weight edges presented in one (i.e., complementary information) or more (i.e., shared information) networks and weaken low-weight edges (i.e., redundant information).
The retention of low-weight edges shared by all layers depends on the tightness of connections of their K-order proximity.
The usage of higher-order proximity in EIF alleviates the dependence on the quality of node embedding.
Besides, the fused network can be easily exploited by traditional single-layer network analysis methods.
Experiments on real-world networks demonstrate that MNSF outperforms the state-of-the-art methods in tasks link prediction and shared community detection.
American Psychological Association (APA)
Ning, Nianwen& Long, Feiyu& Wang, Chunchun& Zhang, Youjie& Yang, Yilin& Wu, Bin. 2020. Nonlinear Structural Fusion for Multiplex Network. Complexity،Vol. 2020, no. 2020, pp.1-17.
https://search.emarefa.net/detail/BIM-1143531
Modern Language Association (MLA)
Ning, Nianwen…[et al.]. Nonlinear Structural Fusion for Multiplex Network. Complexity No. 2020 (2020), pp.1-17.
https://search.emarefa.net/detail/BIM-1143531
American Medical Association (AMA)
Ning, Nianwen& Long, Feiyu& Wang, Chunchun& Zhang, Youjie& Yang, Yilin& Wu, Bin. Nonlinear Structural Fusion for Multiplex Network. Complexity. 2020. Vol. 2020, no. 2020, pp.1-17.
https://search.emarefa.net/detail/BIM-1143531
Data Type
Journal Articles
Language
English
Notes
Includes bibliographical references
Record ID
BIM-1143531