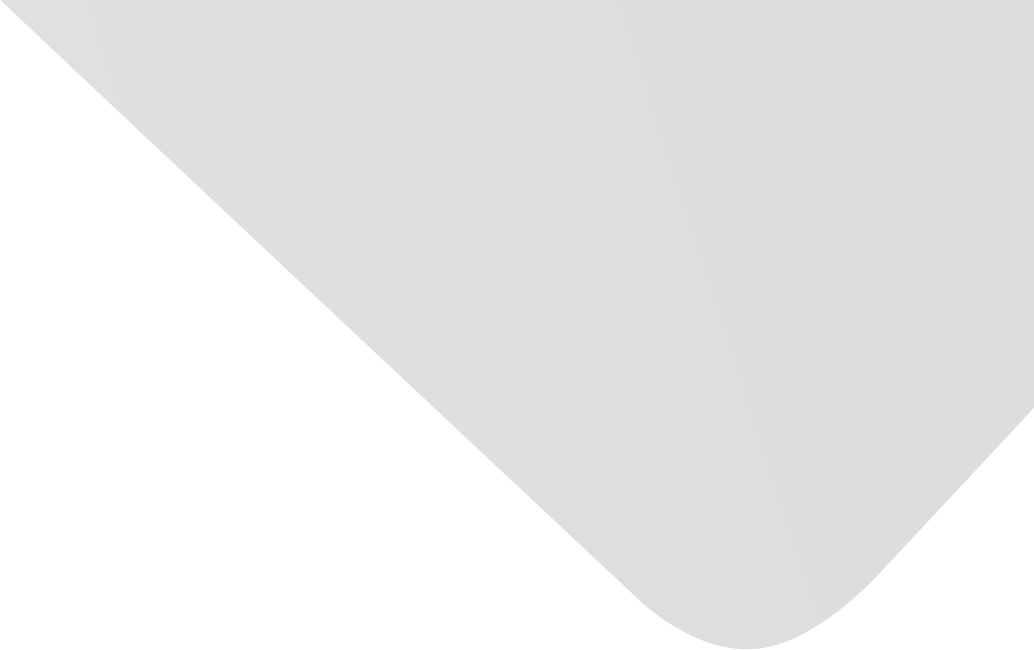
Reinforcement Learning-Based Routing Protocol to Minimize Channel Switching and Interference for Cognitive Radio Networks
Joint Authors
Safdar Malik, Tauqeer
Hasan, Mohd Hilmi
Source
Issue
Vol. 2020, Issue 2020 (31 Dec. 2020), pp.1-24, 24 p.
Publisher
Hindawi Publishing Corporation
Publication Date
2020-08-13
Country of Publication
Egypt
No. of Pages
24
Main Subjects
Abstract EN
In the existing network-layered architectural stack of Cognitive Radio Ad Hoc Network (CRAHN), channel selection is performed at the Medium Access Control (MAC) layer.
However, routing is done on the network layer.
Due to this limitation, the Secondary/Unlicensed Users (SUs) need to access the channel information from the MAC layer whenever the channel switching event occurred during the data transmission.
This issue delayed the channel selection process during the immediate routing decision for the channel switching event to continue the transmission.
In this paper, a protocol is proposed to implement the channel selection decisions at the network layer during the routing process.
The decision is based on past and expected future routing decisions of Primary Users (PUs).
A learning agent operating in the cross-layer mode of the network-layered architectural stack is implemented in the spectrum mobility manager to pass the channel information to the network layer.
This information is originated at the MAC layer.
The channel selection is performed on the basis of reinforcement learning algorithms such as No-External Regret Learning, Q-Learning, and Learning Automata.
This leads to minimizing the channel switching events and user interferences in the Reinforcement Learning- (RL-) based routing protocol.
Simulations are conducted using Cognitive Radio Cognitive Network simulator based on Network Simulator (NS-2).
The simulation results showed that the proposed routing protocol performed better than all the other comparative routing protocols in terms of number of channel switching events, average data rate, packet collision, packet loss, and end-to-end delay.
The proposed routing protocol implies the improved Quality of Service (QoS) of the delay sensitive and real-time networks such as Cellular and Tele Vision (TV) networks.
American Psychological Association (APA)
Safdar Malik, Tauqeer& Hasan, Mohd Hilmi. 2020. Reinforcement Learning-Based Routing Protocol to Minimize Channel Switching and Interference for Cognitive Radio Networks. Complexity،Vol. 2020, no. 2020, pp.1-24.
https://search.emarefa.net/detail/BIM-1144160
Modern Language Association (MLA)
Safdar Malik, Tauqeer& Hasan, Mohd Hilmi. Reinforcement Learning-Based Routing Protocol to Minimize Channel Switching and Interference for Cognitive Radio Networks. Complexity No. 2020 (2020), pp.1-24.
https://search.emarefa.net/detail/BIM-1144160
American Medical Association (AMA)
Safdar Malik, Tauqeer& Hasan, Mohd Hilmi. Reinforcement Learning-Based Routing Protocol to Minimize Channel Switching and Interference for Cognitive Radio Networks. Complexity. 2020. Vol. 2020, no. 2020, pp.1-24.
https://search.emarefa.net/detail/BIM-1144160
Data Type
Journal Articles
Language
English
Notes
Includes bibliographical references
Record ID
BIM-1144160