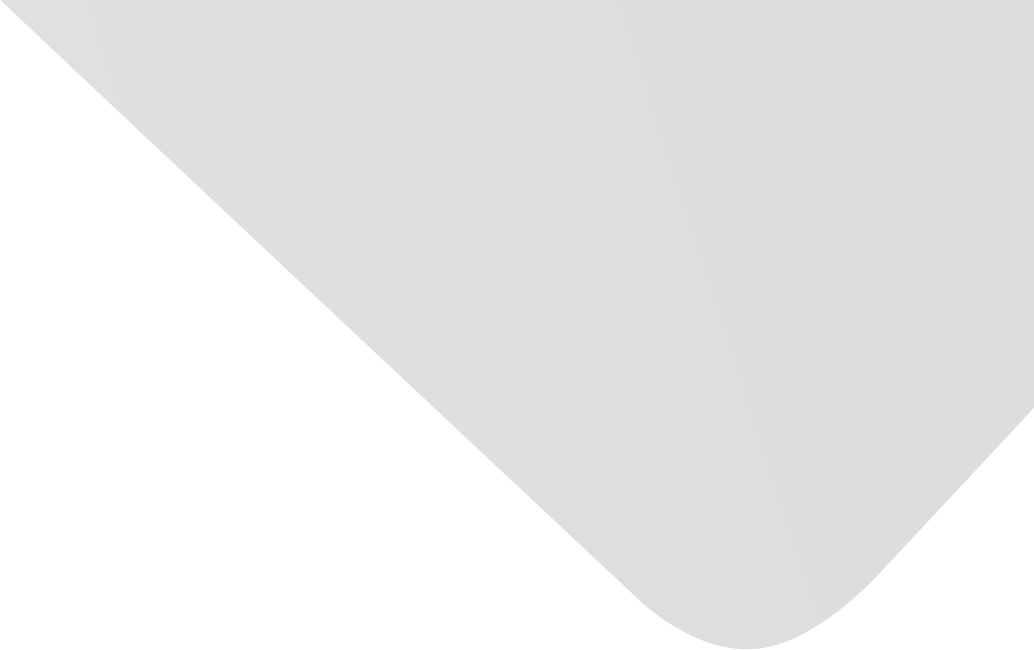
Resilience Analysis of Urban Road Networks Based on Adaptive Signal Controls: Day-to-Day Traffic Dynamics with Deep Reinforcement Learning
Joint Authors
Ochieng, Washington Y.
Shang, Wen-Long
Li, Xingang
Chen, Yanyan
Source
Issue
Vol. 2020, Issue 2020 (31 Dec. 2020), pp.1-19, 19 p.
Publisher
Hindawi Publishing Corporation
Publication Date
2020-11-21
Country of Publication
Egypt
No. of Pages
19
Main Subjects
Abstract EN
Improving the resilience of urban road networks suffering from various disruptions has been a central focus for urban emergence management.
However, to date the effective methods which may mitigate the negative impacts caused by the disruptions, such as road accidents and natural disasters, on urban road networks is highly insufficient.
This study proposes a novel adaptive signal control strategy based on a doubly dynamic learning framework, which consists of deep reinforcement learning and day-to-day traffic dynamic learning, to improve the network performance by adjusting red/green time split.
In this study, red time split is regarded as extra traffic flow to discourage drivers to use affected roads, so as to reduce congestion and improve the resilience when urban road networks are subject to different levels of disruptions.
In addition, we utilize the convolution neural network as Q-network to approximate Q values, link flow distribution and link capacity are regarded as the state space, and actions are denoted as red/green time split.
A small network is utilized as a numerical example, and a fixed time signal control and other two adaptive signal controls are employed for the comparisons with the proposed one.
The results show that the proposed adaptive signal control based on deep reinforcement learning can achieve better resilience in most of the cases, particularly in the scenarios of moderate and severe disruptions.
This study may shed light on the advantages of the proposed adaptive signal control dealing with major emergencies compared to others.
American Psychological Association (APA)
Shang, Wen-Long& Chen, Yanyan& Li, Xingang& Ochieng, Washington Y.. 2020. Resilience Analysis of Urban Road Networks Based on Adaptive Signal Controls: Day-to-Day Traffic Dynamics with Deep Reinforcement Learning. Complexity،Vol. 2020, no. 2020, pp.1-19.
https://search.emarefa.net/detail/BIM-1144805
Modern Language Association (MLA)
Shang, Wen-Long…[et al.]. Resilience Analysis of Urban Road Networks Based on Adaptive Signal Controls: Day-to-Day Traffic Dynamics with Deep Reinforcement Learning. Complexity No. 2020 (2020), pp.1-19.
https://search.emarefa.net/detail/BIM-1144805
American Medical Association (AMA)
Shang, Wen-Long& Chen, Yanyan& Li, Xingang& Ochieng, Washington Y.. Resilience Analysis of Urban Road Networks Based on Adaptive Signal Controls: Day-to-Day Traffic Dynamics with Deep Reinforcement Learning. Complexity. 2020. Vol. 2020, no. 2020, pp.1-19.
https://search.emarefa.net/detail/BIM-1144805
Data Type
Journal Articles
Language
English
Notes
Includes bibliographical references
Record ID
BIM-1144805