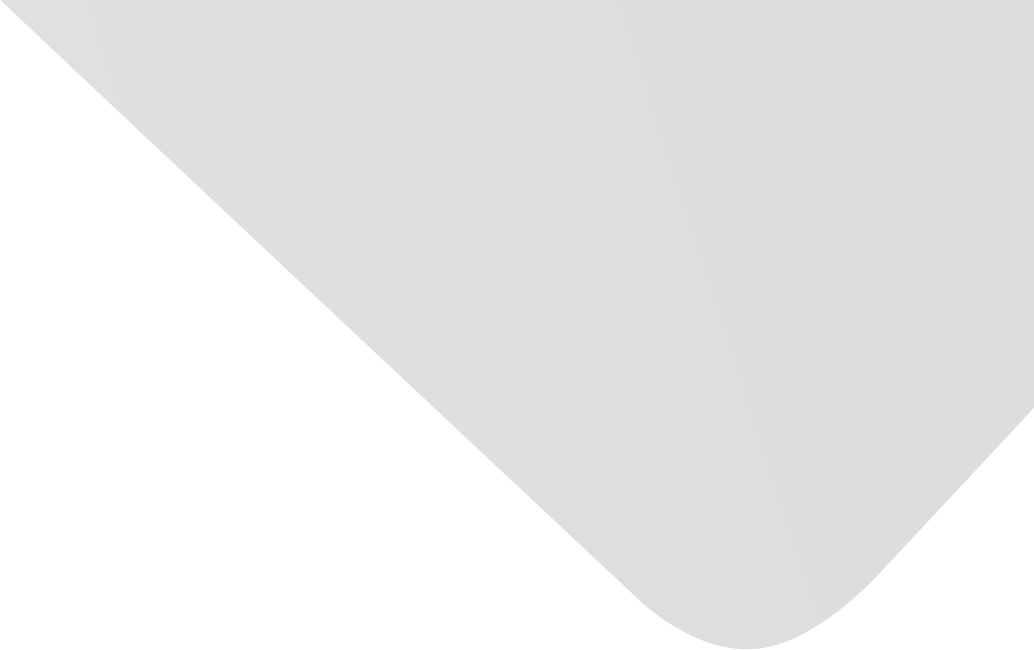
MTAD-TF: Multivariate Time Series Anomaly Detection Using the Combination of Temporal Pattern and Feature Pattern
Joint Authors
He, Q.
Zheng, Y. J.
Zhang, C.L.
Wang, H. Y.
Source
Issue
Vol. 2020, Issue 2020 (31 Dec. 2020), pp.1-9, 9 p.
Publisher
Hindawi Publishing Corporation
Publication Date
2020-10-29
Country of Publication
Egypt
No. of Pages
9
Main Subjects
Abstract EN
Currently, multivariate time series anomaly detection has made great progress in many fields and occupied an important position.
The common limitation of many related studies is that there is only temporal pattern without capturing the relationship between variables and the loss of information leads to false warnings.
Our article proposes an unsupervised multivariate time series anomaly detection.
In the prediction part, multiscale convolution and graph attention network are mainly used to capture information in temporal pattern with feature pattern.
The threshold selection part uses the root mean square error between the predicted value and the actual value to perform extreme value analysis to obtain the threshold.
Finally, the model in this paper outperforms other latest models on actual datasets.
American Psychological Association (APA)
He, Q.& Zheng, Y. J.& Zhang, C.L.& Wang, H. Y.. 2020. MTAD-TF: Multivariate Time Series Anomaly Detection Using the Combination of Temporal Pattern and Feature Pattern. Complexity،Vol. 2020, no. 2020, pp.1-9.
https://search.emarefa.net/detail/BIM-1144881
Modern Language Association (MLA)
He, Q.…[et al.]. MTAD-TF: Multivariate Time Series Anomaly Detection Using the Combination of Temporal Pattern and Feature Pattern. Complexity No. 2020 (2020), pp.1-9.
https://search.emarefa.net/detail/BIM-1144881
American Medical Association (AMA)
He, Q.& Zheng, Y. J.& Zhang, C.L.& Wang, H. Y.. MTAD-TF: Multivariate Time Series Anomaly Detection Using the Combination of Temporal Pattern and Feature Pattern. Complexity. 2020. Vol. 2020, no. 2020, pp.1-9.
https://search.emarefa.net/detail/BIM-1144881
Data Type
Journal Articles
Language
English
Notes
Includes bibliographical references
Record ID
BIM-1144881