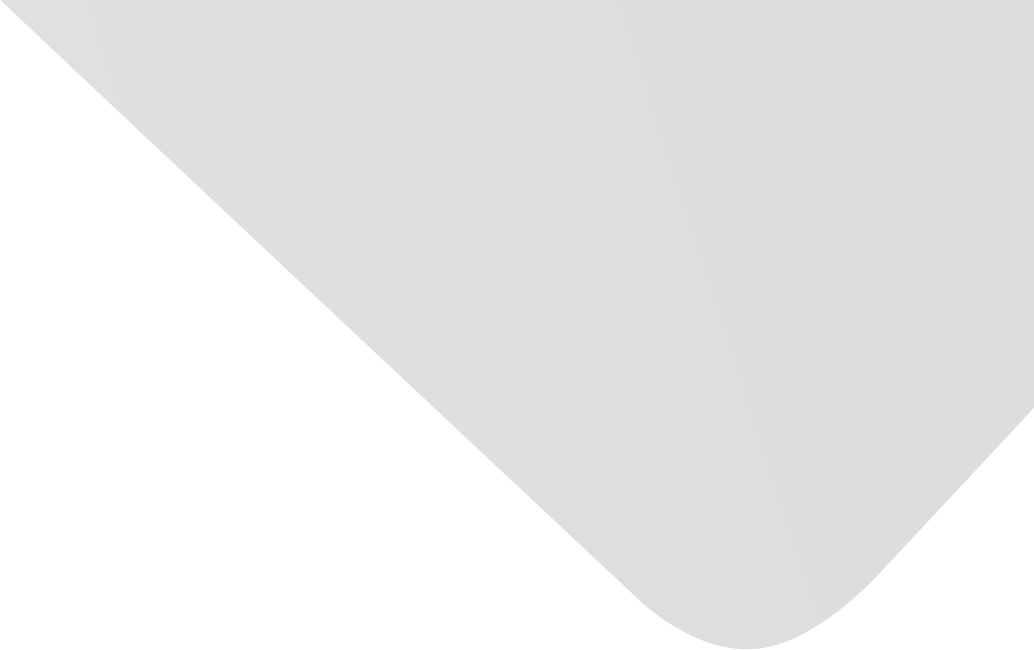
A Penalized h-Likelihood Variable Selection Algorithm for Generalized Linear Regression Models with Random Effects
Joint Authors
Yan, Ruixia
Xia, Zhijie
Xie, Yanxi
Li, Yuewen
Luan, Dongqing
Source
Issue
Vol. 2020, Issue 2020 (31 Dec. 2020), pp.1-13, 13 p.
Publisher
Hindawi Publishing Corporation
Publication Date
2020-09-15
Country of Publication
Egypt
No. of Pages
13
Main Subjects
Abstract EN
Reinforcement learning is one of the paradigms and methodologies of machine learning developed in the computational intelligence community.
Reinforcement learning algorithms present a major challenge in complex dynamics recently.
In the perspective of variable selection, we often come across situations where too many variables are included in the full model at the initial stage of modeling.
Due to a high-dimensional and intractable integral of longitudinal data, likelihood inference is computationally challenging.
It can be computationally difficult such as very slow convergence or even nonconvergence, for the computationally intensive methods.
Recently, hierarchical likelihood (h-likelihood) plays an important role in inferences for models having unobservable or unobserved random variables.
This paper focuses linear models with random effects in the mean structure and proposes a penalized h-likelihood algorithm which incorporates variable selection procedures in the setting of mean modeling via h-likelihood.
The penalized h-likelihood method avoids the messy integration for the random effects and is computationally efficient.
Furthermore, it demonstrates good performance in relevant-variable selection.
Throughout theoretical analysis and simulations, it is confirmed that the penalized h-likelihood algorithm produces good fixed effect estimation results and can identify zero regression coefficients in modeling the mean structure.
American Psychological Association (APA)
Xie, Yanxi& Li, Yuewen& Xia, Zhijie& Yan, Ruixia& Luan, Dongqing. 2020. A Penalized h-Likelihood Variable Selection Algorithm for Generalized Linear Regression Models with Random Effects. Complexity،Vol. 2020, no. 2020, pp.1-13.
https://search.emarefa.net/detail/BIM-1145286
Modern Language Association (MLA)
Xie, Yanxi…[et al.]. A Penalized h-Likelihood Variable Selection Algorithm for Generalized Linear Regression Models with Random Effects. Complexity No. 2020 (2020), pp.1-13.
https://search.emarefa.net/detail/BIM-1145286
American Medical Association (AMA)
Xie, Yanxi& Li, Yuewen& Xia, Zhijie& Yan, Ruixia& Luan, Dongqing. A Penalized h-Likelihood Variable Selection Algorithm for Generalized Linear Regression Models with Random Effects. Complexity. 2020. Vol. 2020, no. 2020, pp.1-13.
https://search.emarefa.net/detail/BIM-1145286
Data Type
Journal Articles
Language
English
Notes
Includes bibliographical references
Record ID
BIM-1145286