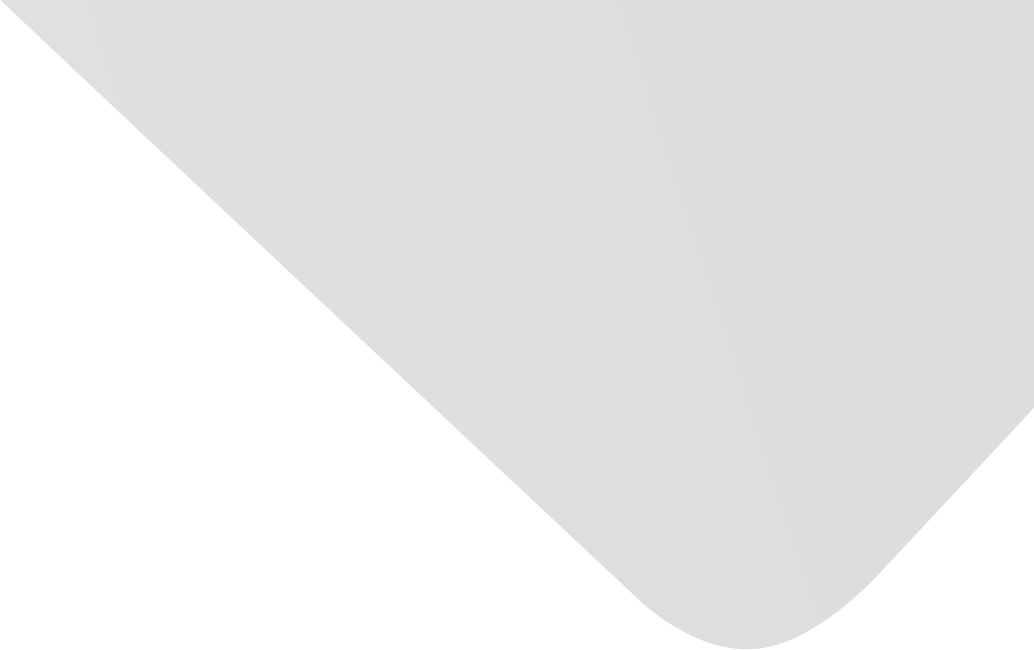
A Hybrid Approach Integrating Multiple ICEEMDANs, WOA, and RVFL Networks for Economic and Financial Time Series Forecasting
Joint Authors
Wu, Jiang
Zhou, Tengfei
Li, Taiyong
Source
Issue
Vol. 2020, Issue 2020 (31 Dec. 2020), pp.1-17, 17 p.
Publisher
Hindawi Publishing Corporation
Publication Date
2020-10-22
Country of Publication
Egypt
No. of Pages
17
Main Subjects
Abstract EN
The fluctuations of economic and financial time series are influenced by various kinds of factors and usually demonstrate strong nonstationary and high complexity.
Therefore, accurately forecasting economic and financial time series is always a challenging research topic.
In this study, a novel multidecomposition and self-optimizing hybrid approach integrating multiple improved complete ensemble empirical mode decompositions with adaptive noise (ICEEMDANs), whale optimization algorithm (WOA), and random vector functional link (RVFL) neural networks, namely, MICEEMDAN-WOA-RVFL, is developed to predict economic and financial time series.
First, we employ ICEEMDAN with random parameters to separate the original time series into a group of comparatively simple subseries multiple times.
Second, we construct RVFL networks to individually forecast each subseries.
Considering the complex parameter settings of RVFL networks, we utilize WOA to search the optimal parameters for RVFL networks simultaneously.
Then, we aggregate the prediction results of individual decomposed subseries as the prediction results of each decomposition, respectively, and finally integrate these prediction results of all the decompositions as the final ensemble prediction results.
The proposed MICEEMDAN-WOA-RVFL remarkably outperforms the compared single and ensemble benchmark models in terms of forecasting accuracy and stability, as demonstrated by the experiments conducted using various economic and financial time series, including West Texas Intermediate (WTI) crude oil prices, US dollar/Euro foreign exchange rate (USD/EUR), US industrial production (IP), and Shanghai stock exchange composite index (SSEC).
American Psychological Association (APA)
Wu, Jiang& Zhou, Tengfei& Li, Taiyong. 2020. A Hybrid Approach Integrating Multiple ICEEMDANs, WOA, and RVFL Networks for Economic and Financial Time Series Forecasting. Complexity،Vol. 2020, no. 2020, pp.1-17.
https://search.emarefa.net/detail/BIM-1145515
Modern Language Association (MLA)
Wu, Jiang…[et al.]. A Hybrid Approach Integrating Multiple ICEEMDANs, WOA, and RVFL Networks for Economic and Financial Time Series Forecasting. Complexity No. 2020 (2020), pp.1-17.
https://search.emarefa.net/detail/BIM-1145515
American Medical Association (AMA)
Wu, Jiang& Zhou, Tengfei& Li, Taiyong. A Hybrid Approach Integrating Multiple ICEEMDANs, WOA, and RVFL Networks for Economic and Financial Time Series Forecasting. Complexity. 2020. Vol. 2020, no. 2020, pp.1-17.
https://search.emarefa.net/detail/BIM-1145515
Data Type
Journal Articles
Language
English
Notes
Includes bibliographical references
Record ID
BIM-1145515