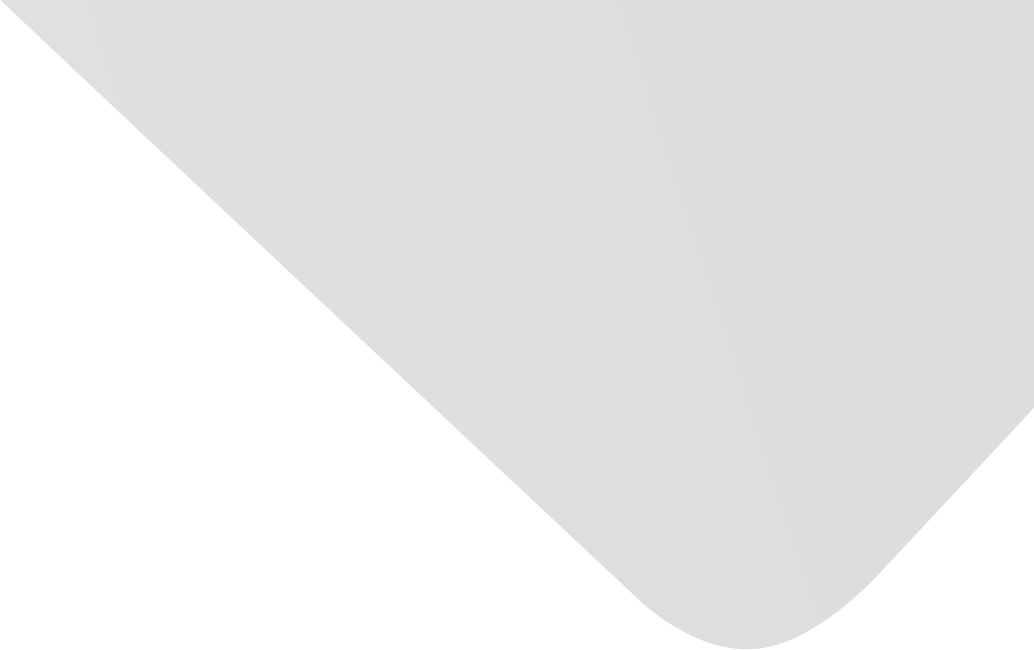
Forecasting Stock Market Volatility: A Combination Approach
Joint Authors
Dai, Zhifeng
Zhou, Huiting
Dong, Xiaodi
Kang, Jie
Source
Discrete Dynamics in Nature and Society
Issue
Vol. 2020, Issue 2020 (31 Dec. 2020), pp.1-9, 9 p.
Publisher
Hindawi Publishing Corporation
Publication Date
2020-06-05
Country of Publication
Egypt
No. of Pages
9
Main Subjects
Abstract EN
We find that combining two important predictors, stock market implied volatility and oil volatility, can improve the predictability of stock return volatility.
We also document that the stock market implied volatility provides far more significant predictability than the oil volatility and other nonoil macroeconomic and financial variables.
The empirical results show the “kitchen sink” combination approach that using two predictors jointly performs better than not only the univariate regression models which use oil volatility or stock market implied volatility separately but also convex combination of the individual forecasts.
This improvement of predictability is also remarkable when we consider the business cycle.
Furthermore, the robust test based on different lag lengths and different macroinformation shows that our forecasting strategy is efficient.
American Psychological Association (APA)
Dai, Zhifeng& Zhou, Huiting& Dong, Xiaodi& Kang, Jie. 2020. Forecasting Stock Market Volatility: A Combination Approach. Discrete Dynamics in Nature and Society،Vol. 2020, no. 2020, pp.1-9.
https://search.emarefa.net/detail/BIM-1152827
Modern Language Association (MLA)
Dai, Zhifeng…[et al.]. Forecasting Stock Market Volatility: A Combination Approach. Discrete Dynamics in Nature and Society No. 2020 (2020), pp.1-9.
https://search.emarefa.net/detail/BIM-1152827
American Medical Association (AMA)
Dai, Zhifeng& Zhou, Huiting& Dong, Xiaodi& Kang, Jie. Forecasting Stock Market Volatility: A Combination Approach. Discrete Dynamics in Nature and Society. 2020. Vol. 2020, no. 2020, pp.1-9.
https://search.emarefa.net/detail/BIM-1152827
Data Type
Journal Articles
Language
English
Notes
Includes bibliographical references
Record ID
BIM-1152827