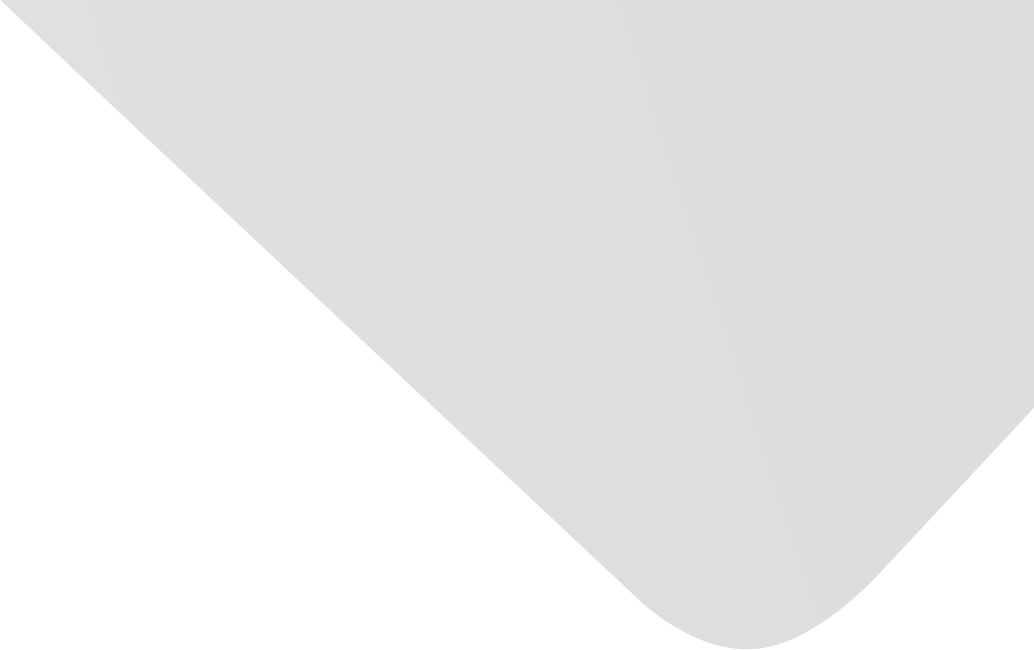
An Improved Spectral Clustering Community Detection Algorithm Based on Probability Matrix
Joint Authors
Ren, Shuxia
Zhang, Shubo
Wu, Tao
Source
Discrete Dynamics in Nature and Society
Issue
Vol. 2020, Issue 2020 (31 Dec. 2020), pp.1-6, 6 p.
Publisher
Hindawi Publishing Corporation
Publication Date
2020-06-04
Country of Publication
Egypt
No. of Pages
6
Main Subjects
Abstract EN
The similarity graphs of most spectral clustering algorithms carry lots of wrong community information.
In this paper, we propose a probability matrix and a novel improved spectral clustering algorithm based on the probability matrix for community detection.
First, the Markov chain is used to calculate the transition probability between nodes, and the probability matrix is constructed by the transition probability.
Then, the similarity graph is constructed with the mean probability matrix.
Finally, community detection is achieved by optimizing the NCut objective function.
The proposed algorithm is compared with SC, WT, FG, FluidC, and SCRW on artificial networks and real networks.
Experimental results show that the proposed algorithm can detect communities more accurately and has better clustering performance.
American Psychological Association (APA)
Ren, Shuxia& Zhang, Shubo& Wu, Tao. 2020. An Improved Spectral Clustering Community Detection Algorithm Based on Probability Matrix. Discrete Dynamics in Nature and Society،Vol. 2020, no. 2020, pp.1-6.
https://search.emarefa.net/detail/BIM-1153052
Modern Language Association (MLA)
Ren, Shuxia…[et al.]. An Improved Spectral Clustering Community Detection Algorithm Based on Probability Matrix. Discrete Dynamics in Nature and Society No. 2020 (2020), pp.1-6.
https://search.emarefa.net/detail/BIM-1153052
American Medical Association (AMA)
Ren, Shuxia& Zhang, Shubo& Wu, Tao. An Improved Spectral Clustering Community Detection Algorithm Based on Probability Matrix. Discrete Dynamics in Nature and Society. 2020. Vol. 2020, no. 2020, pp.1-6.
https://search.emarefa.net/detail/BIM-1153052
Data Type
Journal Articles
Language
English
Notes
Includes bibliographical references
Record ID
BIM-1153052