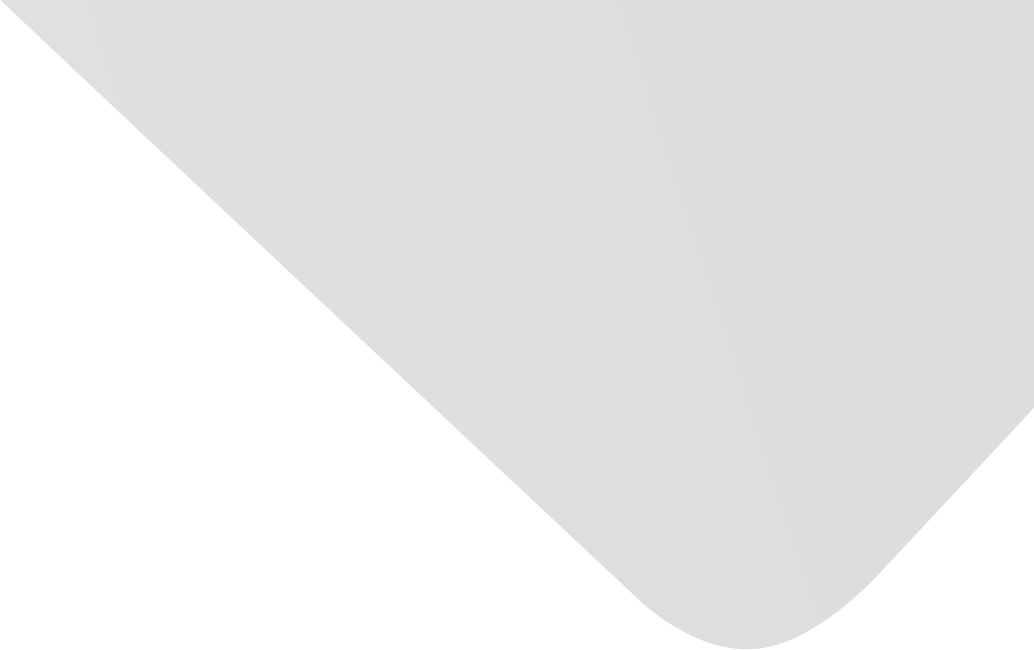
Improving Deep Learning for Forecasting Accuracy in Financial Data
Joint Authors
Source
Discrete Dynamics in Nature and Society
Issue
Vol. 2020, Issue 2020 (31 Dec. 2020), pp.1-12, 12 p.
Publisher
Hindawi Publishing Corporation
Publication Date
2020-03-26
Country of Publication
Egypt
No. of Pages
12
Main Subjects
Abstract EN
Financial forecasting is based on the use of past and present financial information to make the best prediction of the future financial situation, to avoid high-risk situations, and to increase benefits.
Such forecasts are of interest to anyone who wants to know the state of possible finances in the future, including investors and decision-makers.
However, the complex nature of financial data makes it difficult to get accurate forecasts.
Artificial intelligence, which has been shown to be suitable for analyzing very complex problems, can be applied to financial forecasting.
Financial data is both nonlinear and nonstationary, with broadband frequency features.
In other words, there is a large range of fluctuation, meaning that predictions made only using long short-term memory (LSTM) are not enough to ensure accuracy.
This study uses an LSTM model for analysis of financial data, followed by a comparison of the analytical results with the actual data to see which has a larger root-mean-square-error (RMSE).
The proposed method combines deep learning with empirical mode decomposition (EMD) to understand and predict financial trends from financial data.
The financial data for this study are from the Taiwan corporate social responsibility (CSR) index.
First, the EMD method is used to transform the CSR index data into a limited number of intrinsic mode functions (IMF).
The bandwidth of these IMFs becomes narrower, with regular cyclic, periodic, or seasonal components in the time domain.
In other words, the range of fluctuation is small.
LSTM is a good way to forecast cyclic or seasonal data.
The forecast result is obtained by adding all the IMFs together.
It has been verified in past studies that only the LSTM and LSTM combined with the EMD can be used.
The analytical results show that smaller RMSEs can be obtained using the LSTM combined with EMD compared to real data.
American Psychological Association (APA)
Lin, Shih-Lin& Huang, Hua-Wei. 2020. Improving Deep Learning for Forecasting Accuracy in Financial Data. Discrete Dynamics in Nature and Society،Vol. 2020, no. 2020, pp.1-12.
https://search.emarefa.net/detail/BIM-1153196
Modern Language Association (MLA)
Lin, Shih-Lin& Huang, Hua-Wei. Improving Deep Learning for Forecasting Accuracy in Financial Data. Discrete Dynamics in Nature and Society No. 2020 (2020), pp.1-12.
https://search.emarefa.net/detail/BIM-1153196
American Medical Association (AMA)
Lin, Shih-Lin& Huang, Hua-Wei. Improving Deep Learning for Forecasting Accuracy in Financial Data. Discrete Dynamics in Nature and Society. 2020. Vol. 2020, no. 2020, pp.1-12.
https://search.emarefa.net/detail/BIM-1153196
Data Type
Journal Articles
Language
English
Notes
Includes bibliographical references
Record ID
BIM-1153196