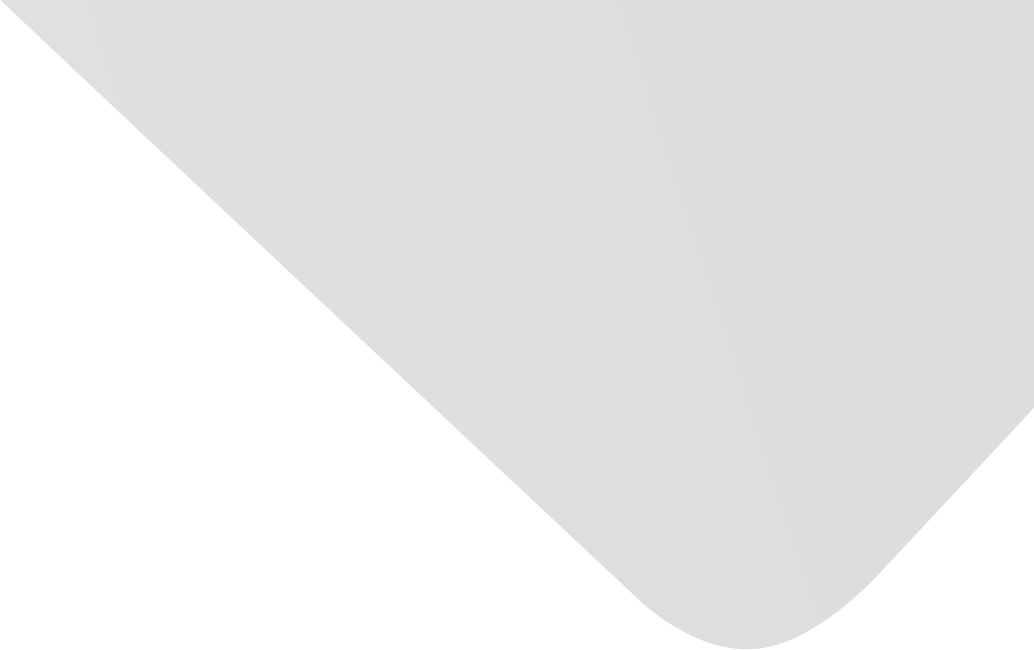
Text to Realistic Image Generation with Attentional Concatenation Generative Adversarial Networks
Joint Authors
Ren, Jinchang
Zhou, Tao
Sun, Yu
Li, Linyan
Hu, Fuyuan
Xi, Xuefeng
Source
Discrete Dynamics in Nature and Society
Issue
Vol. 2020, Issue 2020 (31 Dec. 2020), pp.1-10, 10 p.
Publisher
Hindawi Publishing Corporation
Publication Date
2020-10-28
Country of Publication
Egypt
No. of Pages
10
Main Subjects
Abstract EN
In this paper, we propose an Attentional Concatenation Generative Adversarial Network (ACGAN) aiming at generating 1024 × 1024 high-resolution images.
First, we propose a multilevel cascade structure, for text-to-image synthesis.
During training progress, we gradually add new layers and, at the same time, use the results and word vectors from the previous layer as inputs to the next layer to generate high-resolution images with photo-realistic details.
Second, the deep attentional multimodal similarity model is introduced into the network, and we match word vectors with images in a common semantic space to compute a fine-grained matching loss for training the generator.
In this way, we can pay attention to the fine-grained information of the word level in the semantics.
Finally, the measure of diversity is added to the discriminator, which enables the generator to obtain more diverse gradient directions and improve the diversity of generated samples.
The experimental results show that the inception scores of the proposed model on the CUB and Oxford-102 datasets have reached 4.48 and 4.16, improved by 2.75% and 6.42% compared to Attentional Generative Adversarial Networks (AttenGAN).
The ACGAN model has a better effect on text-generated images, and the resulting image is closer to the real image.
American Psychological Association (APA)
Li, Linyan& Sun, Yu& Hu, Fuyuan& Zhou, Tao& Xi, Xuefeng& Ren, Jinchang. 2020. Text to Realistic Image Generation with Attentional Concatenation Generative Adversarial Networks. Discrete Dynamics in Nature and Society،Vol. 2020, no. 2020, pp.1-10.
https://search.emarefa.net/detail/BIM-1153280
Modern Language Association (MLA)
Li, Linyan…[et al.]. Text to Realistic Image Generation with Attentional Concatenation Generative Adversarial Networks. Discrete Dynamics in Nature and Society No. 2020 (2020), pp.1-10.
https://search.emarefa.net/detail/BIM-1153280
American Medical Association (AMA)
Li, Linyan& Sun, Yu& Hu, Fuyuan& Zhou, Tao& Xi, Xuefeng& Ren, Jinchang. Text to Realistic Image Generation with Attentional Concatenation Generative Adversarial Networks. Discrete Dynamics in Nature and Society. 2020. Vol. 2020, no. 2020, pp.1-10.
https://search.emarefa.net/detail/BIM-1153280
Data Type
Journal Articles
Language
English
Notes
Includes bibliographical references
Record ID
BIM-1153280