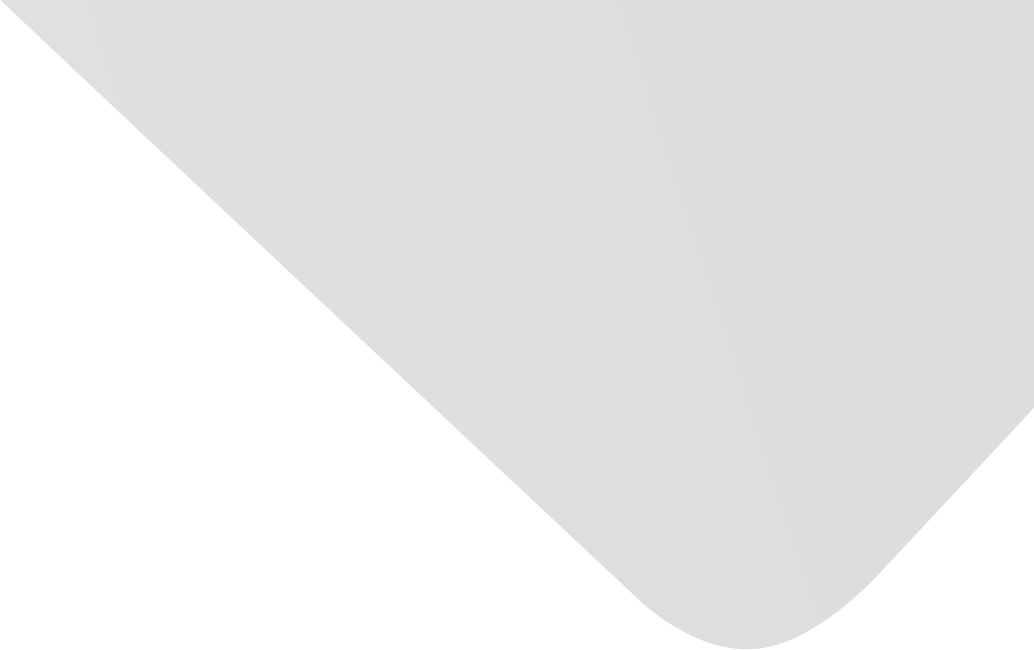
Random-Parameter Multivariate Negative Binomial Regression for Modeling Impacts of Contributing Factors on the Crash Frequency by Crash Types
Joint Authors
Xiao, Feng
Cheng, Jianchuan
Hou, Mingyu
Wang, Chenzhu
Zhang, Ping
Chen, Fei
Bo, Wu
Source
Discrete Dynamics in Nature and Society
Issue
Vol. 2020, Issue 2020 (31 Dec. 2020), pp.1-13, 13 p.
Publisher
Hindawi Publishing Corporation
Publication Date
2020-11-28
Country of Publication
Egypt
No. of Pages
13
Main Subjects
Abstract EN
Highways provide the basis for safe and efficient driving.
Road geometry plays a critical role in dynamic driving systems.
Contributing factors such as plane, longitudinal alignment, and traffic volume, as well as drivers’ sight characteristics, determine the safe operating speed of cars and trucks.
In turn, the operating speed influences the frequency and type of crashes on the highways.
Methods.
Independent negative binomial and Poisson models are considered as the base approaches to modeling in this study.
However, random-parameter models reduce unobserved heterogeneity and obtain higher dimensions.
Therefore, we propose the random-parameter multivariate negative binomial (RPMNB) model to analyze the influence of the traffic, speed, road geometry, and sight characteristics on the rear-end, bumping-guardrail, other, noncasualty, and casualty crashes.
Subsequently, we compute the goodness-of-fit and predictive measures to confirm the superiority of the proposed model.
Finally, we also calculate the elasticity effects to augment the comparison.
Results.
Among the significant variables, black spots, average annual daily traffic volume (AADT), operating speed of cars, speed difference of cars, and length of the present plane curve positively influence the crash risk, whereas the speed difference of trucks, length of the longitudinal slope corresponding to the minimum grade, and stopping sight distance negatively influence the crash risk.
Based on the results, several practical and efficient measures can be taken to promote safety during the road design and operating processes.
Moreover, the goodness-of-fit and predictive measures clearly highlight the greater performance of the RPMNB model compared to standard models.
The elasticity effects across all the models show comparable performance with the RPMNB model.
Thus, the RPMNB model reduces the unobserved heterogeneity and yields better performance in terms of precision, with more consistent explanatory power compared to the traditional models.
American Psychological Association (APA)
Wang, Chenzhu& Chen, Fei& Cheng, Jianchuan& Bo, Wu& Zhang, Ping& Hou, Mingyu…[et al.]. 2020. Random-Parameter Multivariate Negative Binomial Regression for Modeling Impacts of Contributing Factors on the Crash Frequency by Crash Types. Discrete Dynamics in Nature and Society،Vol. 2020, no. 2020, pp.1-13.
https://search.emarefa.net/detail/BIM-1153311
Modern Language Association (MLA)
Wang, Chenzhu…[et al.]. Random-Parameter Multivariate Negative Binomial Regression for Modeling Impacts of Contributing Factors on the Crash Frequency by Crash Types. Discrete Dynamics in Nature and Society No. 2020 (2020), pp.1-13.
https://search.emarefa.net/detail/BIM-1153311
American Medical Association (AMA)
Wang, Chenzhu& Chen, Fei& Cheng, Jianchuan& Bo, Wu& Zhang, Ping& Hou, Mingyu…[et al.]. Random-Parameter Multivariate Negative Binomial Regression for Modeling Impacts of Contributing Factors on the Crash Frequency by Crash Types. Discrete Dynamics in Nature and Society. 2020. Vol. 2020, no. 2020, pp.1-13.
https://search.emarefa.net/detail/BIM-1153311
Data Type
Journal Articles
Language
English
Notes
Includes bibliographical references
Record ID
BIM-1153311