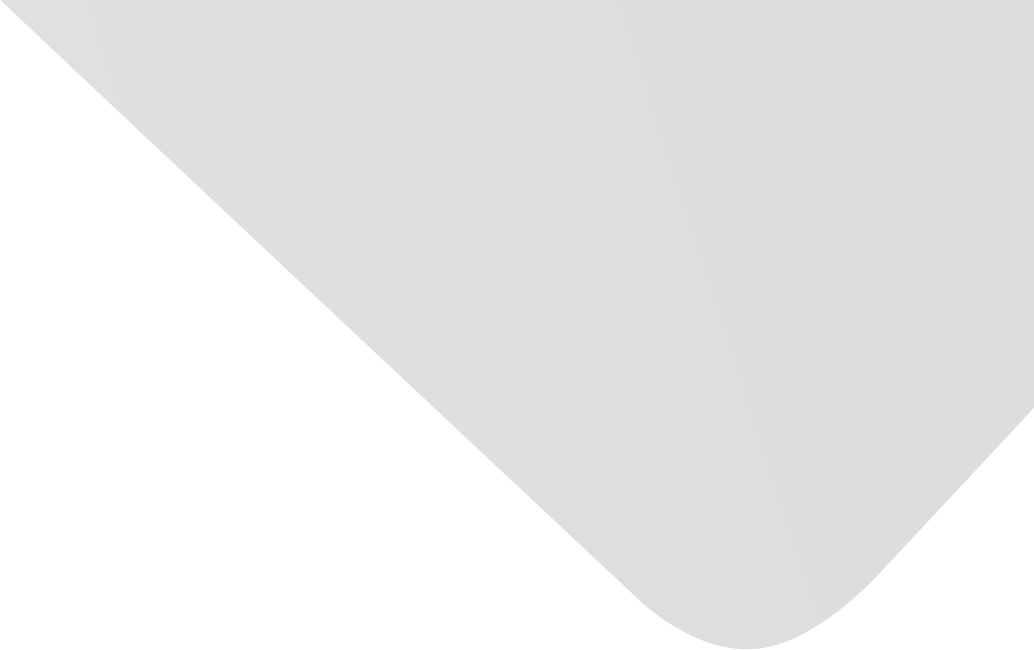
Development of a Three-Stage Hybrid Model by Utilizing a Two-Stage Signal Decomposition Methodology and Machine Learning Approach to Predict Monthly Runoff at Swat River Basin, Pakistan
Joint Authors
Sibtain, Muhammad
Li, Xianshan
Nabi, Ghulam
Azam, Muhammad Imran
Bashir, Hassan
Source
Discrete Dynamics in Nature and Society
Issue
Vol. 2020, Issue 2020 (31 Dec. 2020), pp.1-19, 19 p.
Publisher
Hindawi Publishing Corporation
Publication Date
2020-05-01
Country of Publication
Egypt
No. of Pages
19
Main Subjects
Abstract EN
Precise and reliable hydrological runoff prediction plays a significant role in the optimal management of hydropower resources.
Nevertheless, the hydrological runoff practically possesses a nonlinear dynamics, and constructing appropriate runoff prediction models to deal with the nonlinearity is a challenging task.
To overcome this difficulty, this paper proposes a three-stage novel hybrid model, namely, CVS (CEEMDAN-VMD-SVM), by coupling the support vector machine (SVM) with a two-stage signal decomposition methodology, combining complete ensemble empirical decomposition with additive noise (CEEMDAN) and variational mode decomposition (VMD), to obtain inclusive information of the runoff time series.
Hydrological runoff data of the Swat River, Pakistan, from 1961 to 2015 were taken for prediction.
CEEMDAN decomposes the runoff time series into subcomponents, and VMD performs further decomposition of the high-frequency component obtained after CEEMDAN decomposition to improve the prediction activity.
Afterward, the SVM algorithm was applied to the decomposed subcomponents for the prediction purpose.
Finally, four statistical indices are utilized to measure the performance of the CVS model compared with other hybrid models including CEEMDAN-VMD-MLP (multilayer perceptron), CEEMDAN-SVM, VMD-SVM, CEEMDAN-MLP, VMD-MLP, SVM, and MLP.
The CVS model performs better during the training period by reducing RMSE by 71.28% and 40.06% compared with MLP and CEEDMAD-VMD-SVM models, respectively.
However, during the testing period, the error reductions include RMSE by 68.37% and 35.33% compared with MLP and CEEDMAD-VMD-SVM models, respectively.
The results highlight that the CVS model outperforms other models in terms of accuracy and error reduction.
The research also highlights the superiority of other hybrid models over standalone in predicting the hydrological runoff.
Therefore, the proposed hybrid model is applicable for the nonlinear features of runoff time series with feasibility for future planning and management of water resources.
American Psychological Association (APA)
Sibtain, Muhammad& Li, Xianshan& Nabi, Ghulam& Azam, Muhammad Imran& Bashir, Hassan. 2020. Development of a Three-Stage Hybrid Model by Utilizing a Two-Stage Signal Decomposition Methodology and Machine Learning Approach to Predict Monthly Runoff at Swat River Basin, Pakistan. Discrete Dynamics in Nature and Society،Vol. 2020, no. 2020, pp.1-19.
https://search.emarefa.net/detail/BIM-1153390
Modern Language Association (MLA)
Sibtain, Muhammad…[et al.]. Development of a Three-Stage Hybrid Model by Utilizing a Two-Stage Signal Decomposition Methodology and Machine Learning Approach to Predict Monthly Runoff at Swat River Basin, Pakistan. Discrete Dynamics in Nature and Society No. 2020 (2020), pp.1-19.
https://search.emarefa.net/detail/BIM-1153390
American Medical Association (AMA)
Sibtain, Muhammad& Li, Xianshan& Nabi, Ghulam& Azam, Muhammad Imran& Bashir, Hassan. Development of a Three-Stage Hybrid Model by Utilizing a Two-Stage Signal Decomposition Methodology and Machine Learning Approach to Predict Monthly Runoff at Swat River Basin, Pakistan. Discrete Dynamics in Nature and Society. 2020. Vol. 2020, no. 2020, pp.1-19.
https://search.emarefa.net/detail/BIM-1153390
Data Type
Journal Articles
Language
English
Notes
Includes bibliographical references
Record ID
BIM-1153390