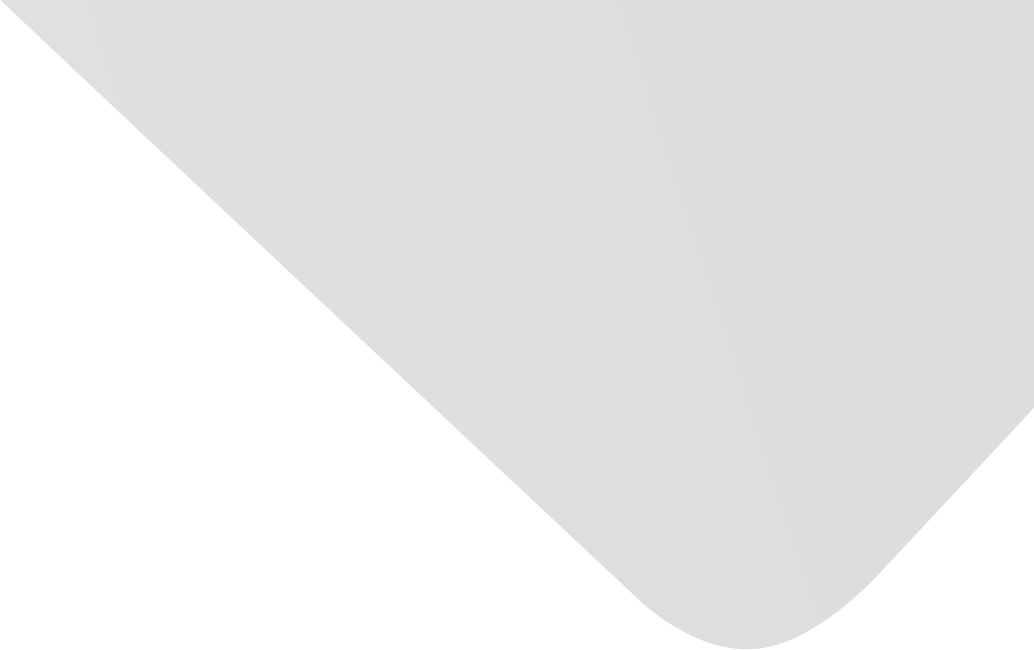
New Approach for Risk Estimation Algorithms of BRCA12 Negativeness Detection with Modelling Supervised Machine Learning Techniques
Joint Authors
Yazici, Hulya
Odemis, Demet Akdeniz
Aksu, Dogukan
Erdogan, Ozge Sukruoglu
Tuncer, Seref Bugra
Avsar, Mukaddes
Kilic, Seda
Turkcan, Gozde Kuru
Celik, Betul
Aydin, Muhammed Ali
Source
Issue
Vol. 2020, Issue 2020 (31 Dec. 2020), pp.1-7, 7 p.
Publisher
Hindawi Publishing Corporation
Publication Date
2020-12-09
Country of Publication
Egypt
No. of Pages
7
Main Subjects
Abstract EN
BRCA1/2 gene testing is a difficult, expensive, and time-consuming test which requires excessive work load.
The identification of the BRCA1/2 gene mutations is significantly important in the selection of treatment and the risk of secondary cancer.
We aimed to develop an algorithm considering all the clinical, demographic, and genetic features of patients for identifying the BRCA1/2 negativity in the present study.
An experimental dataset was created with the collection of the all clinical, demographic, and genetic features of breast cancer patients for 20 years.
This dataset consisted of 125 features of 2070 high-risk breast cancer patients.
All data were numeralized and normalized for detection of the BRCA1/2 negativity in the machine learning algorithm.
The performance of the algorithm was identified by studying the machine learning model with the test data.
k nearest neighbours (KNN) and decision tree (DT) accuracy rates of 9 features involving Dataset 2 were found to be the most effective.
The removal of the unnecessary data in the dataset by reducing the number of features was shown to increase the accuracy rate of algorithm compared with the DT.
BRCA1/2 negativity was identified without performing the BRCA1/2 gene test with 92.88% accuracy within minutes in high-risk breast cancer patients with this algorithm, and the test associated result waiting stress, time, and money loss were prevented.
That algorithm is suggested be useful in fast performing of the treatment plans of patients and accurately in addition to speeding up the clinical practice.
American Psychological Association (APA)
Yazici, Hulya& Odemis, Demet Akdeniz& Aksu, Dogukan& Erdogan, Ozge Sukruoglu& Tuncer, Seref Bugra& Avsar, Mukaddes…[et al.]. 2020. New Approach for Risk Estimation Algorithms of BRCA12 Negativeness Detection with Modelling Supervised Machine Learning Techniques. Disease Markers،Vol. 2020, no. 2020, pp.1-7.
https://search.emarefa.net/detail/BIM-1154045
Modern Language Association (MLA)
Yazici, Hulya…[et al.]. New Approach for Risk Estimation Algorithms of BRCA12 Negativeness Detection with Modelling Supervised Machine Learning Techniques. Disease Markers No. 2020 (2020), pp.1-7.
https://search.emarefa.net/detail/BIM-1154045
American Medical Association (AMA)
Yazici, Hulya& Odemis, Demet Akdeniz& Aksu, Dogukan& Erdogan, Ozge Sukruoglu& Tuncer, Seref Bugra& Avsar, Mukaddes…[et al.]. New Approach for Risk Estimation Algorithms of BRCA12 Negativeness Detection with Modelling Supervised Machine Learning Techniques. Disease Markers. 2020. Vol. 2020, no. 2020, pp.1-7.
https://search.emarefa.net/detail/BIM-1154045
Data Type
Journal Articles
Language
English
Notes
Includes bibliographical references
Record ID
BIM-1154045