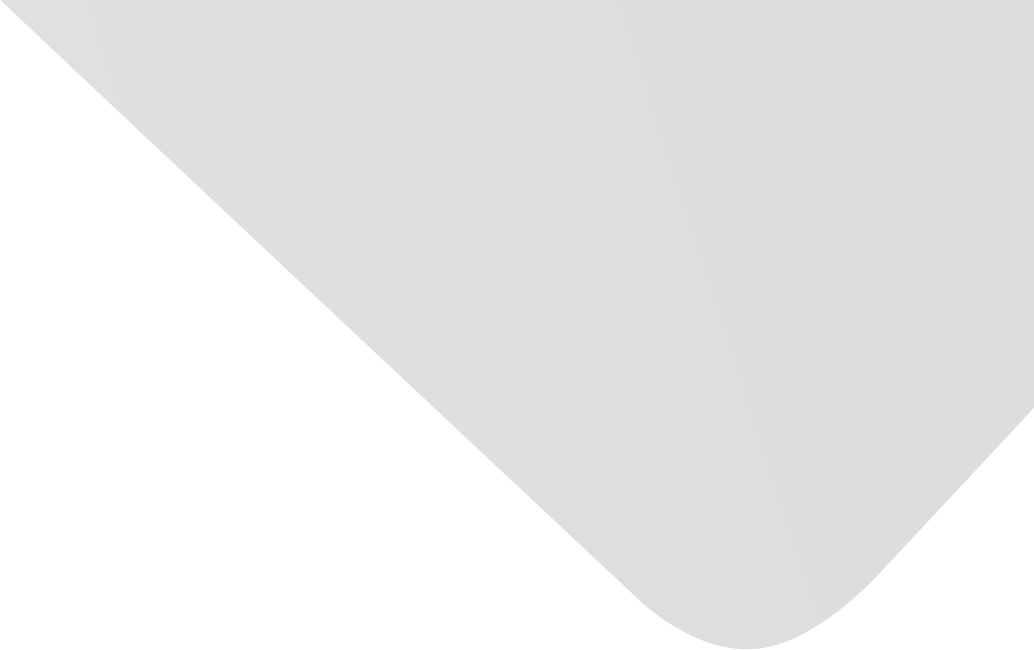
A Microcosmic Syndrome Differentiation Model for Metabolic Syndrome with Multilabel Learning
Joint Authors
Zhu, Long
Vong, Chi Teng
Zhang, Jia
Xia, Shujie
Gao, Bizhen
Li, Candong
Du, Guodong
Li, Shaozi
Yang, Zhaoyang
Xin, Jiliang
Source
Evidence-Based Complementary and Alternative Medicine
Issue
Vol. 2020, Issue 2020 (31 Dec. 2020), pp.1-10, 10 p.
Publisher
Hindawi Publishing Corporation
Publication Date
2020-11-26
Country of Publication
Egypt
No. of Pages
10
Main Subjects
Abstract EN
Background.
Metabolic syndrome (MS) is a complex multisystem disease.
Traditional Chinese medicine (TCM) is effective in preventing and treating MS.
Syndrome differentiation is the basis of TCM treatment, which is composed of location and/or nature syndrome elements.
At present, there are still some problems for objective and comprehensive syndrome differentiation in MS.
This study mainly proposes a solution to two problems.
Firstly, TCM syndromes are concurrent, that is, multiple TCM syndromes may develop in the same patient.
Secondly, there is a lack of holistic exploration of the relationship between microscopic indexes, and TCM syndromes.
In regard to these two problems, multilabel learning (MLL) method in machine learning can be used to solve them, and a microcosmic syndrome differentiation model can also be built innovatively, which can provide a foundation for the establishment of the next model of multidimensional syndrome differentiation in MS.
Methods.
The standardization scale of TCM four diagnostic information for MS was designed, which was used to obtain the results of TCM diagnosis.
The model of microcosmic syndrome differentiation was constructed based on 39 physicochemical indexes by MLL techniques, called ML-kNN.
Firstly, the multilabel learning method was compared with three commonly used single learning algorithms.
Then, the results from ML-kNN were compared between physicochemical indexes and TCM information.
Finally, the influence of the parameter k on the diagnostic model was investigated and the best k value was chosen for TCM diagnosis.
Results.
A total of 698 cases were collected for the modeling of the microcosmic diagnosis of MS.
The comprehensive performance of the ML-kNN model worked obviously better than the others, where the average precision of diagnosis was 71.4%.
The results from ML-kNN based on physicochemical indexes were similar to the results based on TCM information.
On the other hand, the k value had less influence on the prediction results from ML-kNN.
Conclusions.
In the present study, the microcosmic syndrome differentiation model of MS with MLL techniques was good at predicting syndrome elements and could be used to solve the diagnosis problems of multiple labels.
Besides, it was suggested that there was a complex correlation between TCM syndrome elements and physicochemical indexes, which worth future investigation to promote the development of objective differentiation of MS.
American Psychological Association (APA)
Xia, Shujie& Zhang, Jia& Du, Guodong& Li, Shaozi& Gao, Bizhen& Li, Candong…[et al.]. 2020. A Microcosmic Syndrome Differentiation Model for Metabolic Syndrome with Multilabel Learning. Evidence-Based Complementary and Alternative Medicine،Vol. 2020, no. 2020, pp.1-10.
https://search.emarefa.net/detail/BIM-1158337
Modern Language Association (MLA)
Gao, Bizhen…[et al.]. A Microcosmic Syndrome Differentiation Model for Metabolic Syndrome with Multilabel Learning. Evidence-Based Complementary and Alternative Medicine No. 2020 (2020), pp.1-10.
https://search.emarefa.net/detail/BIM-1158337
American Medical Association (AMA)
Xia, Shujie& Zhang, Jia& Du, Guodong& Li, Shaozi& Gao, Bizhen& Li, Candong…[et al.]. A Microcosmic Syndrome Differentiation Model for Metabolic Syndrome with Multilabel Learning. Evidence-Based Complementary and Alternative Medicine. 2020. Vol. 2020, no. 2020, pp.1-10.
https://search.emarefa.net/detail/BIM-1158337
Data Type
Journal Articles
Language
English
Notes
Includes bibliographical references
Record ID
BIM-1158337