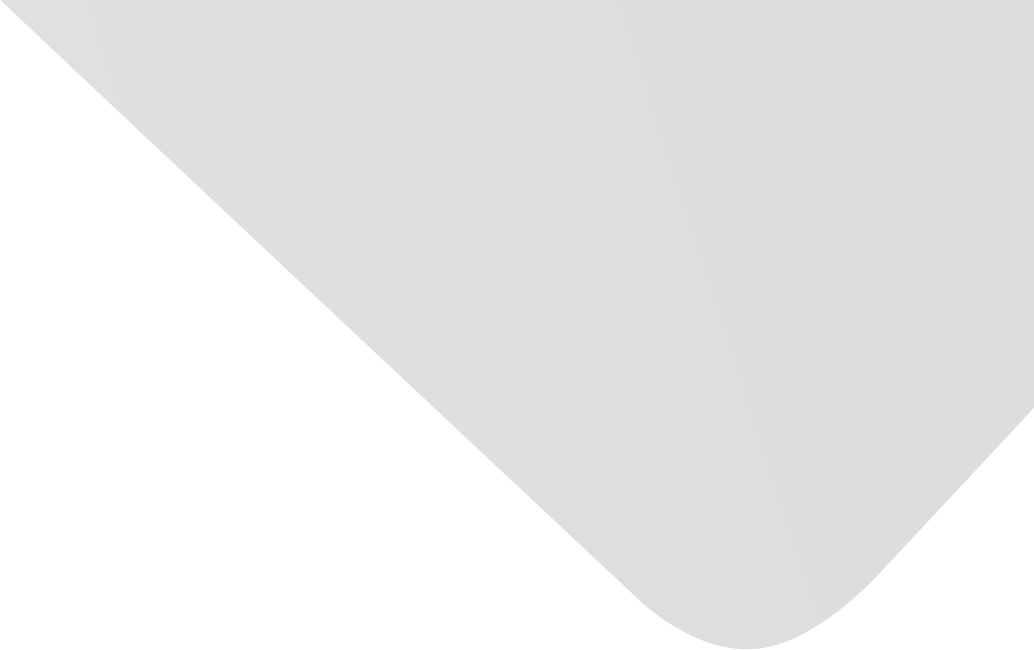
Generation of Synthetic Density Log Data Using Deep Learning Algorithm at the Golden Field in Alberta, Canada
Joint Authors
Kim, Sungil
Min, Baehyun
Lee, Kyungbook
Kim, Kwang Hyun
Lim, Jungtek
Source
Issue
Vol. 2020, Issue 2020 (31 Dec. 2020), pp.1-26, 26 p.
Publisher
Hindawi Publishing Corporation
Publication Date
2020-01-31
Country of Publication
Egypt
No. of Pages
26
Main Subjects
Abstract EN
This study proposes a deep neural network- (DNN-) based prediction model for creating synthetic log.
Unlike previous studies, it focuses on building a reliable prediction model based on two criteria: fit-for-purpose of a target field (the Golden field in Alberta) and compliance with domain knowledge.
First, in the target field, the density log has advantages over the sonic log for porosity analysis because of the carbonate depositional environment.
Considering the correlation between the density and sonic logs, we determine the sonic log as input and the density log as output for the DNN.
Although only five wells have a pair of training data in the field (i.e., sonic and density logs), we obtain, based on geological knowledge, 29 additional wells sharing the same depositional setting in the Slave Point Formation.
After securing the data, 5 wells among the 29 wells are excluded from dataset during preprocessing procedures (elimination of abnormal data and min–max normalisation) to improve the prediction model.
Two cases are designed according to usage of the well information at the target field.
Case 1 uses only 23 of the surrounding wells to train the prediction model, and another surrounding well is used for model testing.
In Case 1, the Levenberg–Marquardt algorithm shows a fast and reliable performance and the numbers of neurons in the two hidden layers are of 45 and 14, respectively.
In Case 2, the 24 surrounding wells and four wells from the target field are used to train the DNN with the optimised parameters from Case 1.
The synthetic density logs from Case 2 mitigate an underestimation problem in Case 1 and follow the overall trend of the true density logs.
The developed prediction model utilises the sonic log for generating the synthetic density log, and a reliable porosity model will be created by combining the given and the synthetic density logs.
American Psychological Association (APA)
Kim, Sungil& Kim, Kwang Hyun& Min, Baehyun& Lim, Jungtek& Lee, Kyungbook. 2020. Generation of Synthetic Density Log Data Using Deep Learning Algorithm at the Golden Field in Alberta, Canada. Geofluids،Vol. 2020, no. 2020, pp.1-26.
https://search.emarefa.net/detail/BIM-1159673
Modern Language Association (MLA)
Kim, Sungil…[et al.]. Generation of Synthetic Density Log Data Using Deep Learning Algorithm at the Golden Field in Alberta, Canada. Geofluids No. 2020 (2020), pp.1-26.
https://search.emarefa.net/detail/BIM-1159673
American Medical Association (AMA)
Kim, Sungil& Kim, Kwang Hyun& Min, Baehyun& Lim, Jungtek& Lee, Kyungbook. Generation of Synthetic Density Log Data Using Deep Learning Algorithm at the Golden Field in Alberta, Canada. Geofluids. 2020. Vol. 2020, no. 2020, pp.1-26.
https://search.emarefa.net/detail/BIM-1159673
Data Type
Journal Articles
Language
English
Notes
Includes bibliographical references
Record ID
BIM-1159673