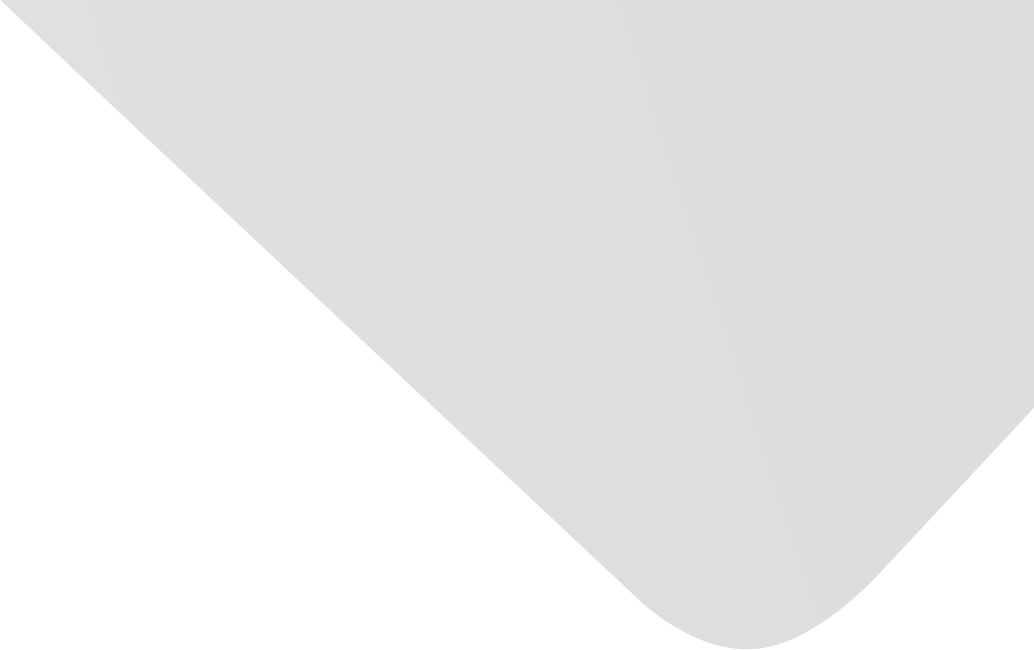
The Daily Container Volumes Prediction of Storage Yard in Port with Long Short-Term Memory Recurrent Neural Network
Joint Authors
Gao, Yinping
Chang, Daofang
Fang, Ting
Fan, Yiqun
Source
Journal of Advanced Transportation
Issue
Vol. 2019, Issue 2019 (31 Dec. 2019), pp.1-11, 11 p.
Publisher
Hindawi Publishing Corporation
Publication Date
2019-12-25
Country of Publication
Egypt
No. of Pages
11
Main Subjects
Abstract EN
The effective forecast of container volumes can provide decision support for port scheduling and operating.
In this work, by deep learning the historical dataset, the long short-term memory (LSTM) recurrent neural network (RNN) is used to predict daily volumes of containers which will enter the storage yard.
The raw dataset of daily container volumes in a certain port is chosen as the training set and preprocessed with box plot.
Then the LSTM model is established with Python and Tensorflow framework.
The comparison between LSTM and other prediction methods like ARIMA model and BP neural network is also provided in this study, and the prediction gap of LSTM is lower than other methods.
It is promising that the proposed LSTM is helpful to predict the daily volumes of containers.
American Psychological Association (APA)
Gao, Yinping& Chang, Daofang& Fang, Ting& Fan, Yiqun. 2019. The Daily Container Volumes Prediction of Storage Yard in Port with Long Short-Term Memory Recurrent Neural Network. Journal of Advanced Transportation،Vol. 2019, no. 2019, pp.1-11.
https://search.emarefa.net/detail/BIM-1169967
Modern Language Association (MLA)
Gao, Yinping…[et al.]. The Daily Container Volumes Prediction of Storage Yard in Port with Long Short-Term Memory Recurrent Neural Network. Journal of Advanced Transportation No. 2019 (2019), pp.1-11.
https://search.emarefa.net/detail/BIM-1169967
American Medical Association (AMA)
Gao, Yinping& Chang, Daofang& Fang, Ting& Fan, Yiqun. The Daily Container Volumes Prediction of Storage Yard in Port with Long Short-Term Memory Recurrent Neural Network. Journal of Advanced Transportation. 2019. Vol. 2019, no. 2019, pp.1-11.
https://search.emarefa.net/detail/BIM-1169967
Data Type
Journal Articles
Language
English
Notes
Includes bibliographical references
Record ID
BIM-1169967