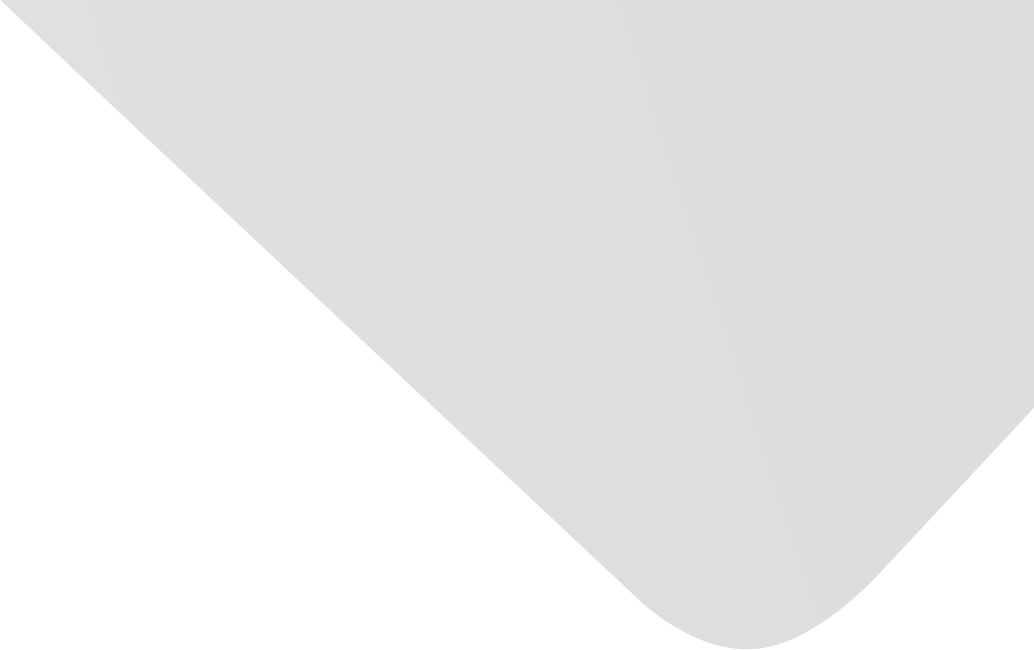
ST-LSTM: A Deep Learning Approach Combined Spatio-Temporal Features for Short-Term Forecast in Rail Transit
Joint Authors
Tang, Qicheng
Yang, Mengning
Yang, Ying
Source
Journal of Advanced Transportation
Issue
Vol. 2019, Issue 2019 (31 Dec. 2019), pp.1-8, 8 p.
Publisher
Hindawi Publishing Corporation
Publication Date
2019-02-06
Country of Publication
Egypt
No. of Pages
8
Main Subjects
Abstract EN
The short-term forecast of rail transit is one of the most essential issues in urban intelligent transportation system (ITS).
Accurate forecast result can provide support for the forewarning of flow outburst and enables passengers to make an appropriate travel plan.
Therefore, it is significant to develop a more accurate forecast model.
Long short-term memory (LSTM) network has been proved to be effective on data with temporal features.
However, it cannot process the correlation between time and space in rail transit.
As a result, a novel forecast model combining spatio-temporal features based on LSTM network (ST-LSTM) is proposed.
Different from other forecast methods, ST-LSTM network uses a new method to extract spatio-temporal features from the data and combines them together as the input.
Compared with other conventional models, ST-LSTM network can achieve a better performance in experiments.
American Psychological Association (APA)
Tang, Qicheng& Yang, Mengning& Yang, Ying. 2019. ST-LSTM: A Deep Learning Approach Combined Spatio-Temporal Features for Short-Term Forecast in Rail Transit. Journal of Advanced Transportation،Vol. 2019, no. 2019, pp.1-8.
https://search.emarefa.net/detail/BIM-1170162
Modern Language Association (MLA)
Tang, Qicheng…[et al.]. ST-LSTM: A Deep Learning Approach Combined Spatio-Temporal Features for Short-Term Forecast in Rail Transit. Journal of Advanced Transportation No. 2019 (2019), pp.1-8.
https://search.emarefa.net/detail/BIM-1170162
American Medical Association (AMA)
Tang, Qicheng& Yang, Mengning& Yang, Ying. ST-LSTM: A Deep Learning Approach Combined Spatio-Temporal Features for Short-Term Forecast in Rail Transit. Journal of Advanced Transportation. 2019. Vol. 2019, no. 2019, pp.1-8.
https://search.emarefa.net/detail/BIM-1170162
Data Type
Journal Articles
Language
English
Notes
Includes bibliographical references
Record ID
BIM-1170162