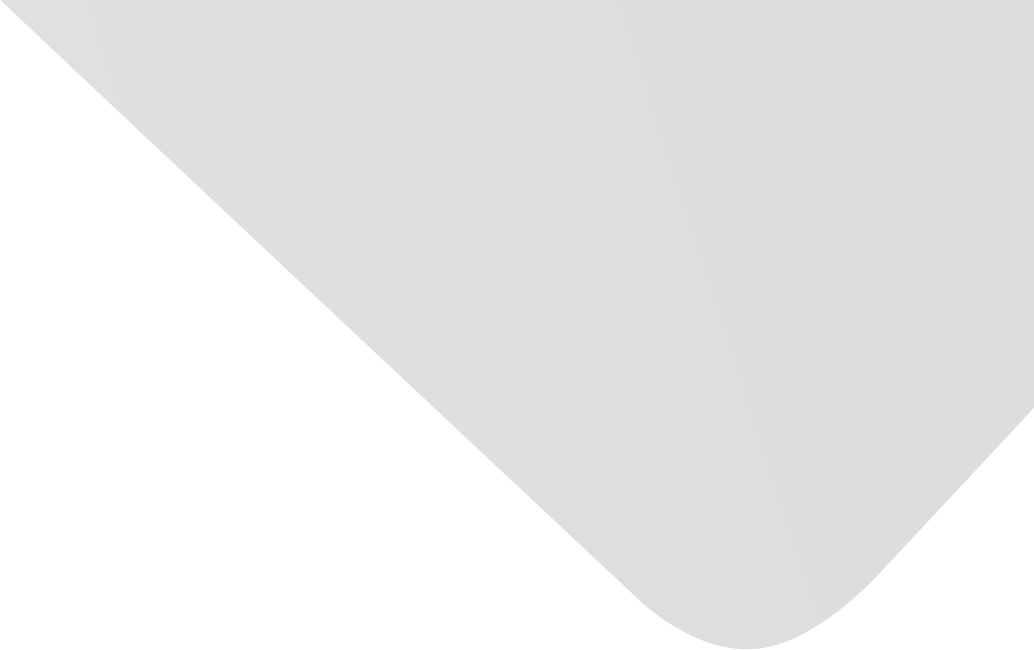
Extracting Vehicle Trajectories Using Unmanned Aerial Vehicles in Congested Traffic Conditions
Joint Authors
Kim, Eui-Jin
Park, Ho-Chul
Kho, Seung-Young
Kim, Dong-Kyu
Ham, Seung-Woo
Source
Journal of Advanced Transportation
Issue
Vol. 2019, Issue 2019 (31 Dec. 2019), pp.1-16, 16 p.
Publisher
Hindawi Publishing Corporation
Publication Date
2019-04-01
Country of Publication
Egypt
No. of Pages
16
Main Subjects
Abstract EN
Obtaining the trajectories of all vehicles in congested traffic is essential for analyzing traffic dynamics.
To conduct an effective analysis using trajectory data, a framework is needed to efficiently and accurately extract the data.
Unfortunately, obtaining accurate trajectories in congested traffic is challenging due to false detections and tracking errors caused by factors in the road environment, such as adjacent vehicles, shadows, road signs, and road facilities.
Unmanned aerial vehicles (UAVs), with incorporating machine learning and image processing, can mitigate these difficulties by their ability to hover above the traffic.
However, research is lacking regarding the extraction and evaluation of vehicle trajectories in congested traffic.
In this study, we propose and compare two learning-based frameworks for detecting vehicles: the aggregated channel feature (ACF), which is based on human-made features, and the faster region-based convolutional neural network (Faster R-CNN), which is based on data-driven features.
We extend the detection results to extract vehicle trajectories in congested traffic conditions from UAV images.
To remove the errors associated with tracking vehicles, we also develop a postprocessing method based on motion constraints.
Then, we conduct detailed performance analyses to confirm the feasibility of the proposed framework on a congested expressway in Korea.
The results show that Faster R-CNN outperforms the ACF in images with large objects and in those with small objects if sufficient data are provided.
This framework extracts the vehicle trajectories with high precision, making them available for analyzing traffic dynamics based on the training of just a small number of positive samples.
The results of this study provide a practical guideline for building a framework to extract vehicles trajectories based on given conditions.
American Psychological Association (APA)
Kim, Eui-Jin& Park, Ho-Chul& Ham, Seung-Woo& Kho, Seung-Young& Kim, Dong-Kyu. 2019. Extracting Vehicle Trajectories Using Unmanned Aerial Vehicles in Congested Traffic Conditions. Journal of Advanced Transportation،Vol. 2019, no. 2019, pp.1-16.
https://search.emarefa.net/detail/BIM-1170262
Modern Language Association (MLA)
Kim, Eui-Jin…[et al.]. Extracting Vehicle Trajectories Using Unmanned Aerial Vehicles in Congested Traffic Conditions. Journal of Advanced Transportation No. 2019 (2019), pp.1-16.
https://search.emarefa.net/detail/BIM-1170262
American Medical Association (AMA)
Kim, Eui-Jin& Park, Ho-Chul& Ham, Seung-Woo& Kho, Seung-Young& Kim, Dong-Kyu. Extracting Vehicle Trajectories Using Unmanned Aerial Vehicles in Congested Traffic Conditions. Journal of Advanced Transportation. 2019. Vol. 2019, no. 2019, pp.1-16.
https://search.emarefa.net/detail/BIM-1170262
Data Type
Journal Articles
Language
English
Notes
Includes bibliographical references
Record ID
BIM-1170262