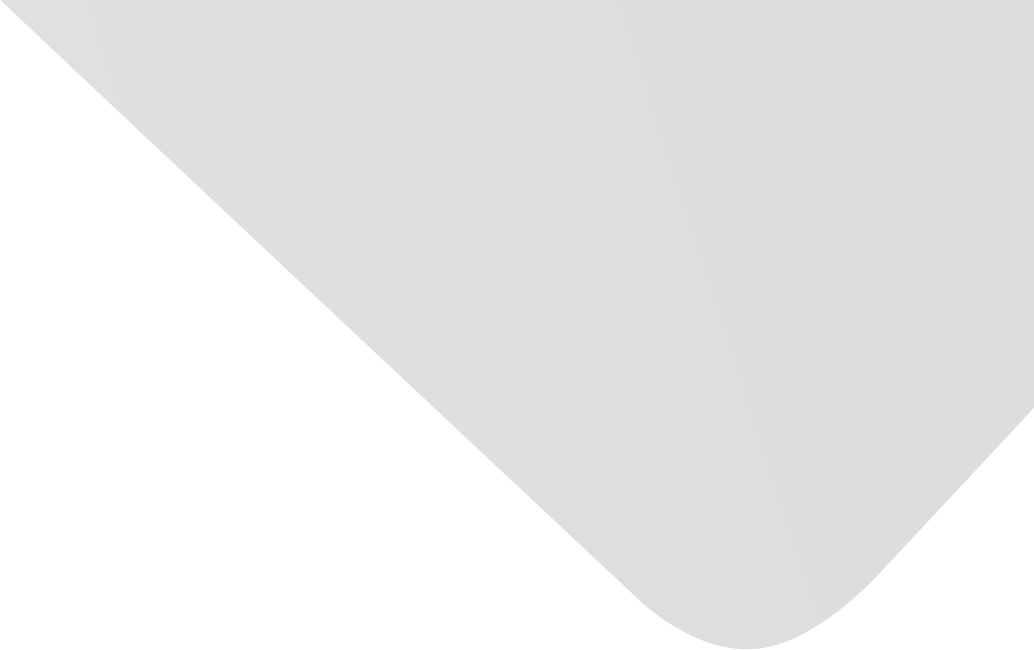
A Hybrid Vision-Map Method for Urban Road Detection
Joint Authors
Fernández, Carlos
Fernández-Llorca, David
Sotelo, Miguel A.
Source
Journal of Advanced Transportation
Issue
Vol. 2017, Issue 2017 (31 Dec. 2017), pp.1-21, 21 p.
Publisher
Hindawi Publishing Corporation
Publication Date
2017-10-30
Country of Publication
Egypt
No. of Pages
21
Main Subjects
Abstract EN
A hybrid vision-map system is presented to solve the road detection problem in urban scenarios.
The standardized use of machine learning techniques in classification problems has been merged with digital navigation map information to increase system robustness.
The objective of this paper is to create a new environment perception method to detect the road in urban environments, fusing stereo vision with digital maps by detecting road appearance and road limits such as lane markings or curbs.
Deep learning approaches make the system hard-coupled to the training set.
Even though our approach is based on machine learning techniques, the features are calculated from different sources (GPS, map, curbs, etc.), making our system less dependent on the training set.
American Psychological Association (APA)
Fernández, Carlos& Fernández-Llorca, David& Sotelo, Miguel A.. 2017. A Hybrid Vision-Map Method for Urban Road Detection. Journal of Advanced Transportation،Vol. 2017, no. 2017, pp.1-21.
https://search.emarefa.net/detail/BIM-1170919
Modern Language Association (MLA)
Fernández, Carlos…[et al.]. A Hybrid Vision-Map Method for Urban Road Detection. Journal of Advanced Transportation No. 2017 (2017), pp.1-21.
https://search.emarefa.net/detail/BIM-1170919
American Medical Association (AMA)
Fernández, Carlos& Fernández-Llorca, David& Sotelo, Miguel A.. A Hybrid Vision-Map Method for Urban Road Detection. Journal of Advanced Transportation. 2017. Vol. 2017, no. 2017, pp.1-21.
https://search.emarefa.net/detail/BIM-1170919
Data Type
Journal Articles
Language
English
Notes
Includes bibliographical references
Record ID
BIM-1170919