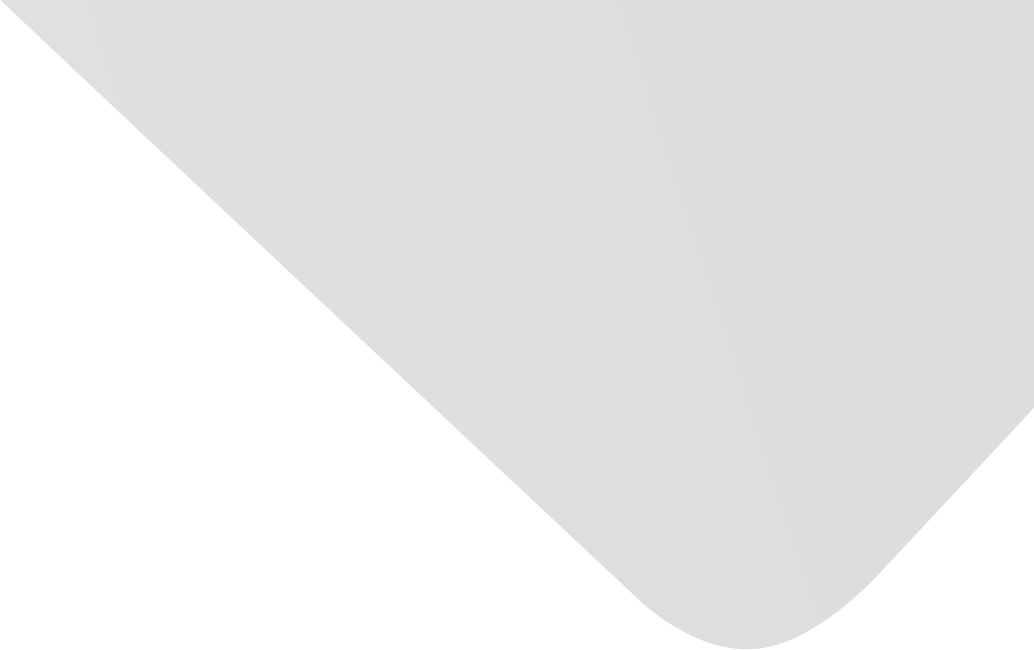
Assessment of Electrocardiogram Rhythms by GoogLeNet Deep Neural Network Architecture
Joint Authors
Kim, Jeong-Hwan
Seo, Seung-Yeon
Song, Chul-Gyu
Kim, Kyeong-Seop
Source
Journal of Healthcare Engineering
Issue
Vol. 2019, Issue 2019 (31 Dec. 2019), pp.1-10, 10 p.
Publisher
Hindawi Publishing Corporation
Publication Date
2019-04-28
Country of Publication
Egypt
No. of Pages
10
Main Subjects
Abstract EN
The aim of this study is to design GoogLeNet deep neural network architecture by expanding the kernel size of the inception layer and combining the convolution layers to classify the electrocardiogram (ECG) beats into a normal sinus rhythm, premature ventricular contraction, atrial premature contraction, and right/left bundle branch block arrhythmia.
Based on testing MIT-BIH arrhythmia benchmark databases, the scope of training/test ECG data was configured by covering at least three and seven R-peak features, and the proposed extended-GoogLeNet architecture can classify five distinct heartbeats; normal sinus rhythm (NSR), premature ventricular contraction (PVC), atrial premature contraction (APC), right bundle branch block (RBBB), and left bundle brunch block(LBBB), with an accuracy of 95.94%, an error rate of 4.06%, a maximum sensitivity of 96.9%, and a maximum positive predictive value of 95.7% for judging a normal or an abnormal beat with considering three ECG segments; an accuracy of 98.31%, a sensitivity of 88.75%, a specificity of 99.4%, and a positive predictive value of 94.4% for classifying APC from NSR, PVC, APC beats, whereas the error rate for misclassifying APC beat was relative low at 6.32%, compared with previous research efforts.
American Psychological Association (APA)
Kim, Jeong-Hwan& Seo, Seung-Yeon& Song, Chul-Gyu& Kim, Kyeong-Seop. 2019. Assessment of Electrocardiogram Rhythms by GoogLeNet Deep Neural Network Architecture. Journal of Healthcare Engineering،Vol. 2019, no. 2019, pp.1-10.
https://search.emarefa.net/detail/BIM-1175106
Modern Language Association (MLA)
Kim, Jeong-Hwan…[et al.]. Assessment of Electrocardiogram Rhythms by GoogLeNet Deep Neural Network Architecture. Journal of Healthcare Engineering No. 2019 (2019), pp.1-10.
https://search.emarefa.net/detail/BIM-1175106
American Medical Association (AMA)
Kim, Jeong-Hwan& Seo, Seung-Yeon& Song, Chul-Gyu& Kim, Kyeong-Seop. Assessment of Electrocardiogram Rhythms by GoogLeNet Deep Neural Network Architecture. Journal of Healthcare Engineering. 2019. Vol. 2019, no. 2019, pp.1-10.
https://search.emarefa.net/detail/BIM-1175106
Data Type
Journal Articles
Language
English
Notes
Includes bibliographical references
Record ID
BIM-1175106