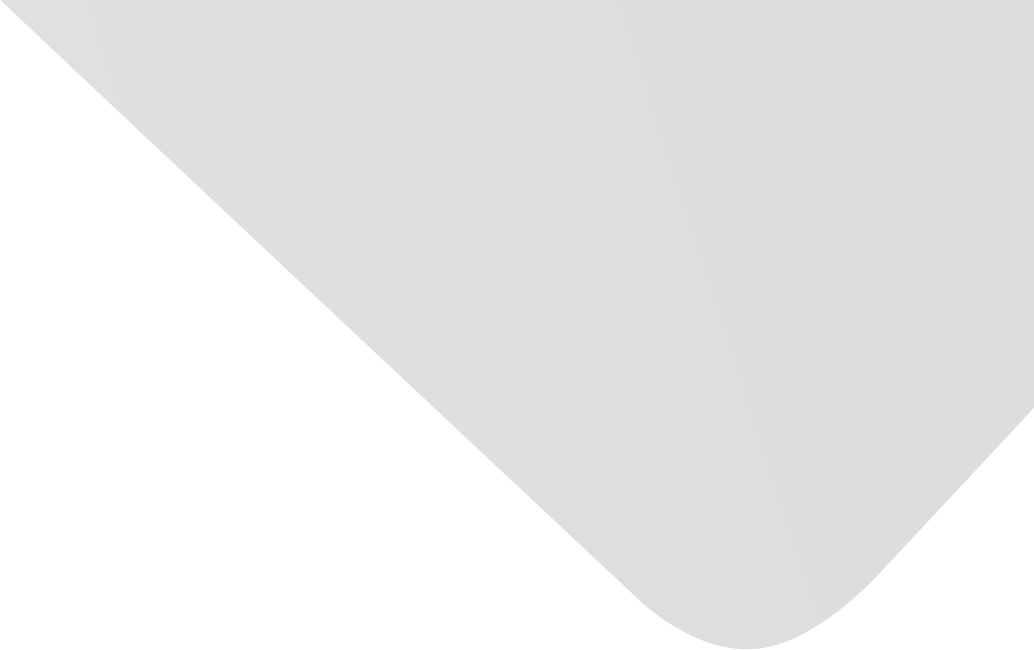
Discovering Insightful Rules among Truck Crash Characteristics using Apriori Algorithm
Joint Authors
Park, Dongjoo
Hong, Jungyeol
Tamakloe, Reuben
Source
Journal of Advanced Transportation
Issue
Vol. 2020, Issue 2020 (31 Dec. 2020), pp.1-16, 16 p.
Publisher
Hindawi Publishing Corporation
Publication Date
2020-01-16
Country of Publication
Egypt
No. of Pages
16
Main Subjects
Abstract EN
This study aims to discover hidden patterns and potential relationships in risk factors in freight truck crash data.
Existing studies mainly used parametric models to analyze the causes of freight vehicle crashes.
However, predetermined assumptions and underlying relationships between independent and dependent variables have been cited as its limitations.
To overcome these limitations and provide a better understanding of factors that lead to truck crashes on the expressways, we applied the Association Rules Mining (ARM) technique, which is a nonparametric method.
ARM quantifies the interrelationships between the antecedents and consequents of truck-involved crashes and provides researchers with the most influential set of factors that leads to crashes.
We utilized a freight vehicle-involved crash data consisting of 19,038 crashes that occurred on the Korean expressways from 2008 to 2017 for this investigation.
From the data, 90,951 association rules were generated through ARM employing the Apriori algorithm.
The lift values estimated by the Apriori algorithm showed the strength of association between risk factors, and based on the estimated lift values, we identified key crash contributory factors that lead to truck-involved crashes at various segment types, under different weather conditions, considering the driver’s age, crash type, driver’s faults, vehicle size, and roadway geometry type.
From the generated rules, we demonstrated that overspeeding with medium-weight trucks was highly associated with crashes during the rainy weather, whereas drowsy driving during the evening was correlated with crashes during fine weather.
Segment-related crashes were mainly associated with driver’s faults and roadway geometry.
Our results present useful insights and suggestions that can be used by transport stakeholders, including policymakers and researchers, to create relevant policies that will help reduce freight truck crashes on the expressways.
American Psychological Association (APA)
Hong, Jungyeol& Tamakloe, Reuben& Park, Dongjoo. 2020. Discovering Insightful Rules among Truck Crash Characteristics using Apriori Algorithm. Journal of Advanced Transportation،Vol. 2020, no. 2020, pp.1-16.
https://search.emarefa.net/detail/BIM-1175630
Modern Language Association (MLA)
Hong, Jungyeol…[et al.]. Discovering Insightful Rules among Truck Crash Characteristics using Apriori Algorithm. Journal of Advanced Transportation No. 2020 (2020), pp.1-16.
https://search.emarefa.net/detail/BIM-1175630
American Medical Association (AMA)
Hong, Jungyeol& Tamakloe, Reuben& Park, Dongjoo. Discovering Insightful Rules among Truck Crash Characteristics using Apriori Algorithm. Journal of Advanced Transportation. 2020. Vol. 2020, no. 2020, pp.1-16.
https://search.emarefa.net/detail/BIM-1175630
Data Type
Journal Articles
Language
English
Notes
Includes bibliographical references
Record ID
BIM-1175630