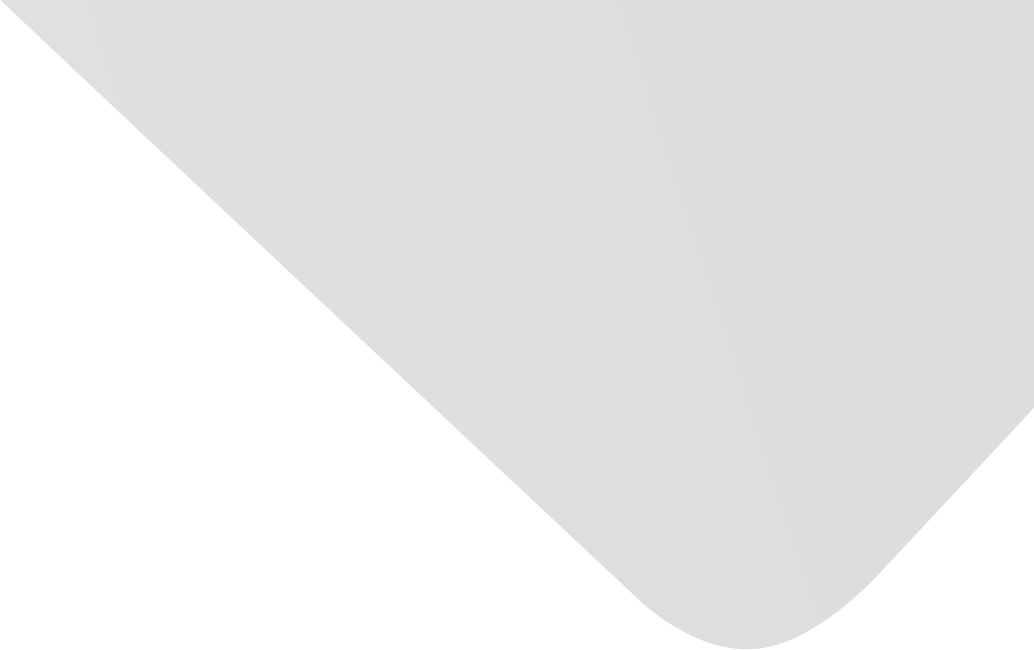
Automated Pavement Crack Damage Detection Using Deep Multiscale Convolutional Features
Joint Authors
Song, Weidong
Jia, Guohui
Zhu, Hong
Jia, Di
Gao, Lin
Source
Journal of Advanced Transportation
Issue
Vol. 2020, Issue 2020 (31 Dec. 2020), pp.1-11, 11 p.
Publisher
Hindawi Publishing Corporation
Publication Date
2020-01-08
Country of Publication
Egypt
No. of Pages
11
Main Subjects
Abstract EN
Road pavement cracks automated detection is one of the key factors to evaluate the road distress quality, and it is a difficult issue for the construction of intelligent maintenance systems.
However, pavement cracks automated detection has been a challenging task, including strong nonuniformity, complex topology, and strong noise-like problems in the crack images, and so on.
To address these challenges, we propose the CrackSeg—an end-to-end trainable deep convolutional neural network for pavement crack detection, which is effective in achieving pixel-level, and automated detection via high-level features.
In this work, we introduce a novel multiscale dilated convolutional module that can learn rich deep convolutional features, making the crack features acquired under a complex background more discriminant.
Moreover, in the upsampling module process, the high spatial resolution features of the shallow network are fused to obtain more refined pixel-level pavement crack detection results.
We train and evaluate the CrackSeg net on our CrackDataset, the experimental results prove that the CrackSeg achieves high performance with a precision of 98.00%, recall of 97.85%, F-score of 97.92%, and a mIoU of 73.53%.
Compared with other state-of-the-art methods, the CrackSeg performs more efficiently, and robustly for automated pavement crack detection.
American Psychological Association (APA)
Song, Weidong& Jia, Guohui& Zhu, Hong& Jia, Di& Gao, Lin. 2020. Automated Pavement Crack Damage Detection Using Deep Multiscale Convolutional Features. Journal of Advanced Transportation،Vol. 2020, no. 2020, pp.1-11.
https://search.emarefa.net/detail/BIM-1175872
Modern Language Association (MLA)
Song, Weidong…[et al.]. Automated Pavement Crack Damage Detection Using Deep Multiscale Convolutional Features. Journal of Advanced Transportation No. 2020 (2020), pp.1-11.
https://search.emarefa.net/detail/BIM-1175872
American Medical Association (AMA)
Song, Weidong& Jia, Guohui& Zhu, Hong& Jia, Di& Gao, Lin. Automated Pavement Crack Damage Detection Using Deep Multiscale Convolutional Features. Journal of Advanced Transportation. 2020. Vol. 2020, no. 2020, pp.1-11.
https://search.emarefa.net/detail/BIM-1175872
Data Type
Journal Articles
Language
English
Notes
Includes bibliographical references
Record ID
BIM-1175872