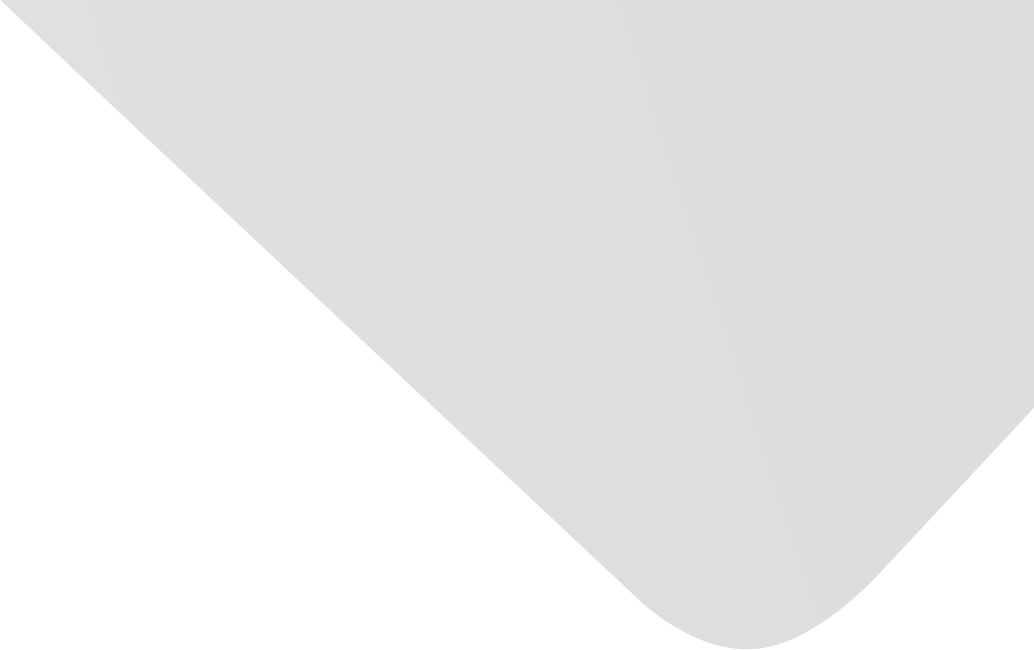
Quality Control of Olive Oils Using Machine Learning and Electronic Nose
Joint Authors
Source
Issue
Vol. 2017, Issue 2017 (31 Dec. 2017), pp.1-7, 7 p.
Publisher
Hindawi Publishing Corporation
Publication Date
2017-10-17
Country of Publication
Egypt
No. of Pages
7
Abstract EN
The adulteration of olive oils can be detected with chemical test.
This is very expensive and takes very long time.
Thus, this study is focused on reducing both time and cost.
For this purpose, the raw data has been collected from olive oils by using an e-nose from different regions in Balikesir in Turkey.
This study presents two methods to analyze quality control of olive oils.
In the first method, 32 inputs are applied to the classifiers directly.
In the second, 32-input collected data are reduced to 8 inputs by Principal Component Analysis.
These reduced data as 8 inputs are applied to the classifiers.
Different machine learning classifiers such as Naïve Bayesian, K-Nearest Neighbors (k-NN), Linear Discriminate Analysis (LDA), Decision Tree, Artificial Neural Networks (ANN), and Support Vector Machine (SVM) were used.
Then performances of these classifiers were compared according to their accuracies.
American Psychological Association (APA)
Ordukaya, Emre& Karlik, Bekir. 2017. Quality Control of Olive Oils Using Machine Learning and Electronic Nose. Journal of Food Quality،Vol. 2017, no. 2017, pp.1-7.
https://search.emarefa.net/detail/BIM-1176177
Modern Language Association (MLA)
Ordukaya, Emre& Karlik, Bekir. Quality Control of Olive Oils Using Machine Learning and Electronic Nose. Journal of Food Quality No. 2017 (2017), pp.1-7.
https://search.emarefa.net/detail/BIM-1176177
American Medical Association (AMA)
Ordukaya, Emre& Karlik, Bekir. Quality Control of Olive Oils Using Machine Learning and Electronic Nose. Journal of Food Quality. 2017. Vol. 2017, no. 2017, pp.1-7.
https://search.emarefa.net/detail/BIM-1176177
Data Type
Journal Articles
Language
English
Notes
Includes bibliographical references
Record ID
BIM-1176177