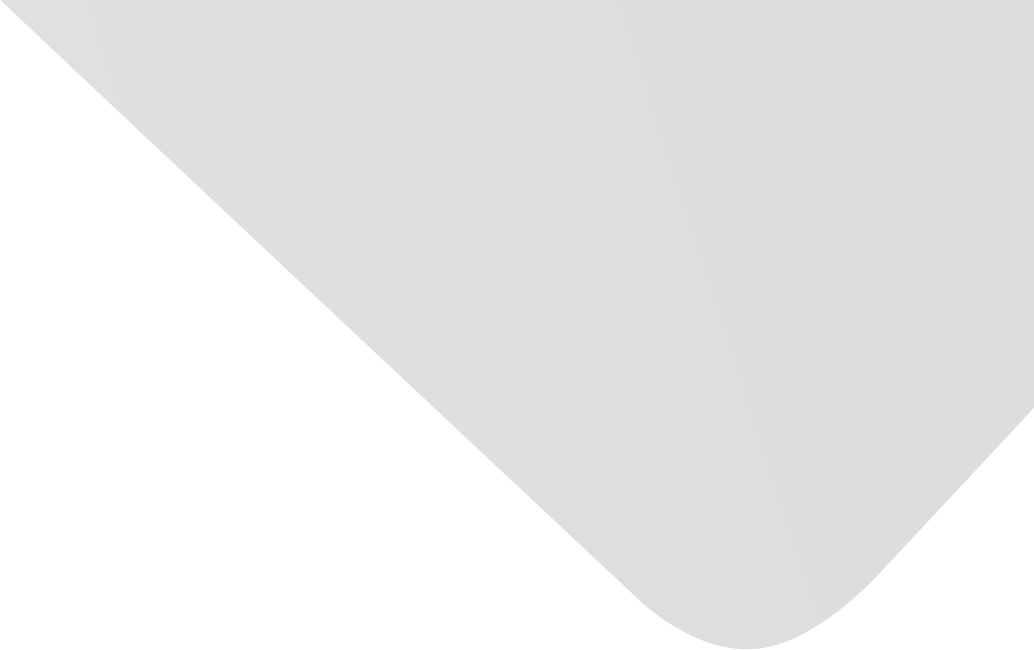
Predicting Critical Bicycle-Vehicle Conflicts at Signalized Intersections
Joint Authors
Darzian Rostami, Alireza
Katthe, Anagha
Sohrabi, Aryan
Jahangiri, Arash
Source
Journal of Advanced Transportation
Issue
Vol. 2020, Issue 2020 (31 Dec. 2020), pp.1-16, 16 p.
Publisher
Hindawi Publishing Corporation
Publication Date
2020-12-03
Country of Publication
Egypt
No. of Pages
16
Main Subjects
Abstract EN
Continuous development of urban infrastructure with a focus on sustainable transportation has led to a proliferation of vulnerable road users (VRUs), such as bicyclists and pedestrians, at intersections.
Intersection safety evaluation has primarily relied on historical crash data.
However, due to several limitations, including rarity, unpredictability, and irregularity of crash occurrences, quantitative and qualitative analyses of crashes may not be accurate.
To transcend these limitations, intersection safety can be proactively evaluated by quantifying near-crashes using alternative measures known as surrogate safety measures (SSMs).
This study focuses on developing models to predict critical near-crashes between vehicles and bicycles at intersections based on SSMs and kinematic data.
Video data from ten signalized intersections in the city of San Diego were employed to train logistic regression (LR), support vector machine (SVM), and random forest (RF) models.
A variation of time-to-collision called T2 and postencroachment time (PET) were used to specify monitoring periods and to identify critical near-crashes, respectively.
Four scenarios were created using two thresholds of 5 and 3 s for both PET and T2.
In each scenario, five monitoring period lengths were examined.
The RF model was superior compared to other models in all different scenarios and across different monitoring period lengths.
The results also showed a small trade-off between model performance and monitoring period length, identifying models with monitoring period lengths of 10 and 20 frames performed slightly better than those with lower or higher lengths.
Sequential backward and forward feature selection methods were also applied that enhanced model performance.
The best RF model had recall values of 85% or higher across all scenarios.
Also, RF prediction models performed better when considering just the rear-end near-crashes with recalls of above 90%.
American Psychological Association (APA)
Darzian Rostami, Alireza& Katthe, Anagha& Sohrabi, Aryan& Jahangiri, Arash. 2020. Predicting Critical Bicycle-Vehicle Conflicts at Signalized Intersections. Journal of Advanced Transportation،Vol. 2020, no. 2020, pp.1-16.
https://search.emarefa.net/detail/BIM-1176197
Modern Language Association (MLA)
Darzian Rostami, Alireza…[et al.]. Predicting Critical Bicycle-Vehicle Conflicts at Signalized Intersections. Journal of Advanced Transportation No. 2020 (2020), pp.1-16.
https://search.emarefa.net/detail/BIM-1176197
American Medical Association (AMA)
Darzian Rostami, Alireza& Katthe, Anagha& Sohrabi, Aryan& Jahangiri, Arash. Predicting Critical Bicycle-Vehicle Conflicts at Signalized Intersections. Journal of Advanced Transportation. 2020. Vol. 2020, no. 2020, pp.1-16.
https://search.emarefa.net/detail/BIM-1176197
Data Type
Journal Articles
Language
English
Notes
Includes bibliographical references
Record ID
BIM-1176197