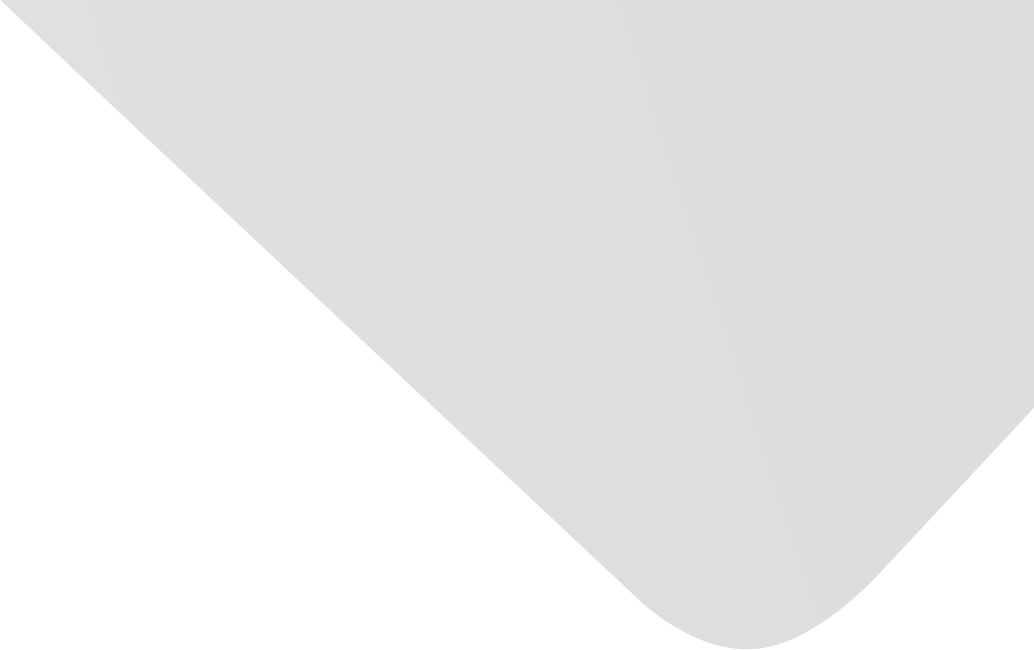
Machine Learning Approach to Quantity Management for Long-Term Sustainable Development of Dockless Public Bike: Case of Shenzhen in China
Joint Authors
Zhou, Qingfeng
Wong, Chun Janice
Su, Xian
Source
Journal of Advanced Transportation
Issue
Vol. 2020, Issue 2020 (31 Dec. 2020), pp.1-13, 13 p.
Publisher
Hindawi Publishing Corporation
Publication Date
2020-11-28
Country of Publication
Egypt
No. of Pages
13
Main Subjects
Abstract EN
Since the number of bicycles is critical to the sustainable development of dockless PBS, this research practiced the introduction of a machine learning approach to quantity management using OFO bike operation data in Shenzhen.
First, two clustering algorithms were used to identify the bicycle gathering area, and the available bike number and coefficient of available bike number variation were analyzed in each bicycle gathering area’s type.
Second, five classification algorithms were compared in the accuracy of distinguishing the type of bicycle gathering areas using 25 impact factors.
Finally, the application of the knowledge gained from the existing dockless bicycle operation data to guide the number planning and management of public bicycles was explored.
We found the following.
(1) There were 492 OFO bicycle gathering areas that can be divided into four types: high inefficient, normal inefficient, high efficient, and normal efficient.
The high inefficient and normal inefficient areas gathered about 110,000 bicycles with low usage.
(2) More types of bicycle gathering area will affect the accuracy of the classification algorithm.
The random forest classification had the best performance in identifying bicycle gathering area types in five classification algorithms with an accuracy of more than 75%.
(3) There were obvious differences in the characteristics of 25 impact factors in four types of bicycle gathering areas.
It is feasible to use these factors to predict area type to optimize the number of available bicycles, reduce operating costs, and improve utilization efficiency.
This work helps operators and government understand the characteristics of dockless PBS and contributes to promoting long-term sustainable development of the system through a machine learning approach.
American Psychological Association (APA)
Zhou, Qingfeng& Wong, Chun Janice& Su, Xian. 2020. Machine Learning Approach to Quantity Management for Long-Term Sustainable Development of Dockless Public Bike: Case of Shenzhen in China. Journal of Advanced Transportation،Vol. 2020, no. 2020, pp.1-13.
https://search.emarefa.net/detail/BIM-1176552
Modern Language Association (MLA)
Zhou, Qingfeng…[et al.]. Machine Learning Approach to Quantity Management for Long-Term Sustainable Development of Dockless Public Bike: Case of Shenzhen in China. Journal of Advanced Transportation No. 2020 (2020), pp.1-13.
https://search.emarefa.net/detail/BIM-1176552
American Medical Association (AMA)
Zhou, Qingfeng& Wong, Chun Janice& Su, Xian. Machine Learning Approach to Quantity Management for Long-Term Sustainable Development of Dockless Public Bike: Case of Shenzhen in China. Journal of Advanced Transportation. 2020. Vol. 2020, no. 2020, pp.1-13.
https://search.emarefa.net/detail/BIM-1176552
Data Type
Journal Articles
Language
English
Notes
Includes bibliographical references
Record ID
BIM-1176552