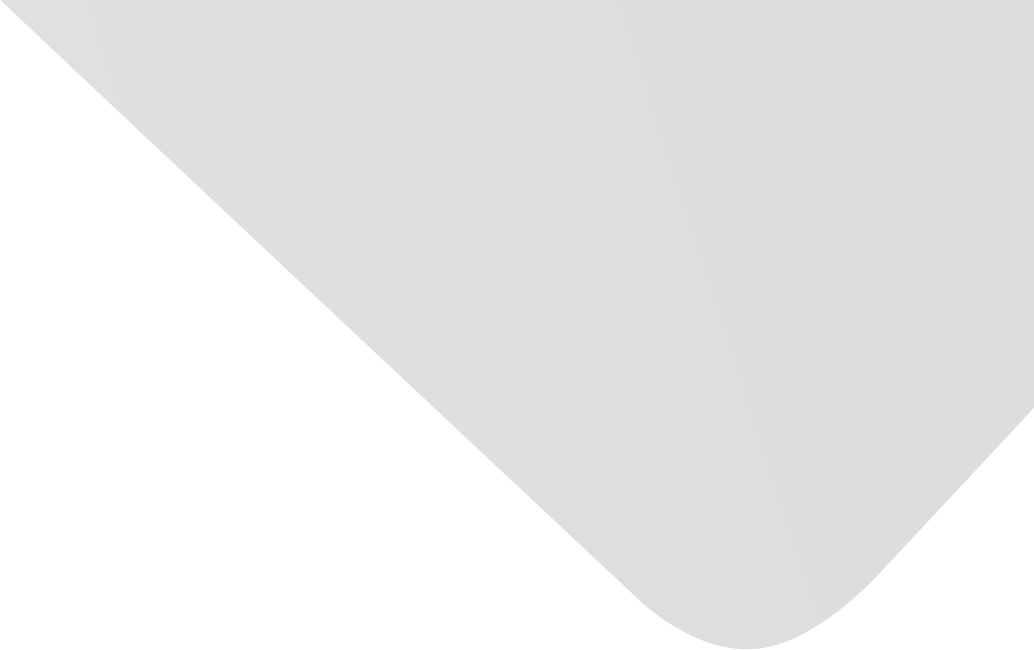
Urban Traffic Flow Forecast Based on FastGCRNN
Joint Authors
Li, Haifeng
Zhang, Ya
Lu, Mingming
Source
Journal of Advanced Transportation
Issue
Vol. 2020, Issue 2020 (31 Dec. 2020), pp.1-9, 9 p.
Publisher
Hindawi Publishing Corporation
Publication Date
2020-09-27
Country of Publication
Egypt
No. of Pages
9
Main Subjects
Abstract EN
Traffic forecasting is an important prerequisite for the application of intelligent transportation systems in urban traffic networks.
The existing works adopted RNN and CNN/GCN, among which GCRN is the state-of-the-art work, to characterize the temporal and spatial correlation of traffic flows.
However, it is hard to apply GCRN to the large-scale road networks due to high computational complexity.
To address this problem, we propose abstracting the road network into a geometric graph and building a Fast Graph Convolution Recurrent Neural Network (FastGCRNN) to model the spatial-temporal dependencies of traffic flow.
Specifically, we use FastGCN unit to efficiently capture the topological relationship between the roads and the surrounding roads in the graph with reducing the computational complexity through importance sampling, combine GRU unit to capture the temporal dependency of traffic flow, and embed the spatiotemporal features into Seq2Seq based on the Encoder-Decoder framework.
Experiments on large-scale traffic data sets illustrate that the proposed method can greatly reduce computational complexity and memory consumption while maintaining relatively high accuracy.
American Psychological Association (APA)
Zhang, Ya& Lu, Mingming& Li, Haifeng. 2020. Urban Traffic Flow Forecast Based on FastGCRNN. Journal of Advanced Transportation،Vol. 2020, no. 2020, pp.1-9.
https://search.emarefa.net/detail/BIM-1180720
Modern Language Association (MLA)
Zhang, Ya…[et al.]. Urban Traffic Flow Forecast Based on FastGCRNN. Journal of Advanced Transportation No. 2020 (2020), pp.1-9.
https://search.emarefa.net/detail/BIM-1180720
American Medical Association (AMA)
Zhang, Ya& Lu, Mingming& Li, Haifeng. Urban Traffic Flow Forecast Based on FastGCRNN. Journal of Advanced Transportation. 2020. Vol. 2020, no. 2020, pp.1-9.
https://search.emarefa.net/detail/BIM-1180720
Data Type
Journal Articles
Language
English
Notes
Includes bibliographical references
Record ID
BIM-1180720