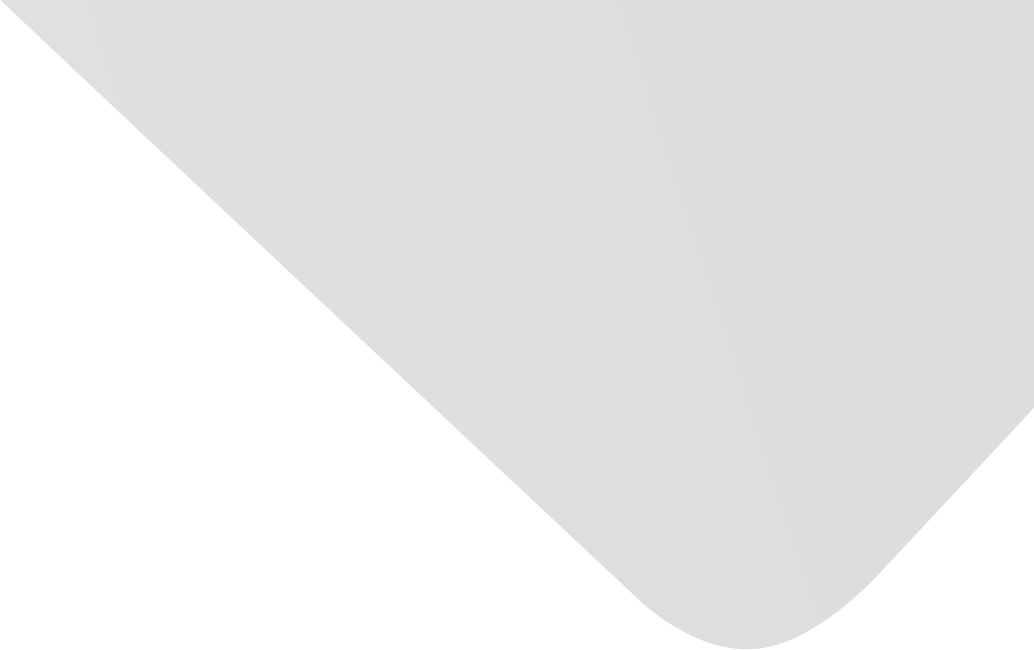
Cell Detection Using Extremal Regions in a Semisupervised Learning Framework
Joint Authors
Ramesh, Nisha
Liu, Ting
Tasdizen, Tolga
Source
Journal of Healthcare Engineering
Issue
Vol. 2017, Issue 2017 (31 Dec. 2017), pp.1-13, 13 p.
Publisher
Hindawi Publishing Corporation
Publication Date
2017-06-14
Country of Publication
Egypt
No. of Pages
13
Main Subjects
Abstract EN
This paper discusses an algorithm to build a semisupervised learning framework for detecting cells.
The cell candidates are represented as extremal regions drawn from a hierarchical image representation.
Training a classifier for cell detection using supervised approaches relies on a large amount of training data, which requires a lot of effort and time.
We propose a semisupervised approach to reduce this burden.
The set of extremal regions is generated using a maximally stable extremal region (MSER) detector.
A subset of nonoverlapping regions with high similarity to the cells of interest is selected.
Using the tree built from the MSER detector, we develop a novel differentiable unsupervised loss term that enforces the nonoverlapping constraint with the learned function.
Our algorithm requires very few examples of cells with simple dot annotations for training.
The supervised and unsupervised losses are embedded in a Bayesian framework for probabilistic learning.
American Psychological Association (APA)
Ramesh, Nisha& Liu, Ting& Tasdizen, Tolga. 2017. Cell Detection Using Extremal Regions in a Semisupervised Learning Framework. Journal of Healthcare Engineering،Vol. 2017, no. 2017, pp.1-13.
https://search.emarefa.net/detail/BIM-1180947
Modern Language Association (MLA)
Ramesh, Nisha…[et al.]. Cell Detection Using Extremal Regions in a Semisupervised Learning Framework. Journal of Healthcare Engineering No. 2017 (2017), pp.1-13.
https://search.emarefa.net/detail/BIM-1180947
American Medical Association (AMA)
Ramesh, Nisha& Liu, Ting& Tasdizen, Tolga. Cell Detection Using Extremal Regions in a Semisupervised Learning Framework. Journal of Healthcare Engineering. 2017. Vol. 2017, no. 2017, pp.1-13.
https://search.emarefa.net/detail/BIM-1180947
Data Type
Journal Articles
Language
English
Notes
Includes bibliographical references
Record ID
BIM-1180947