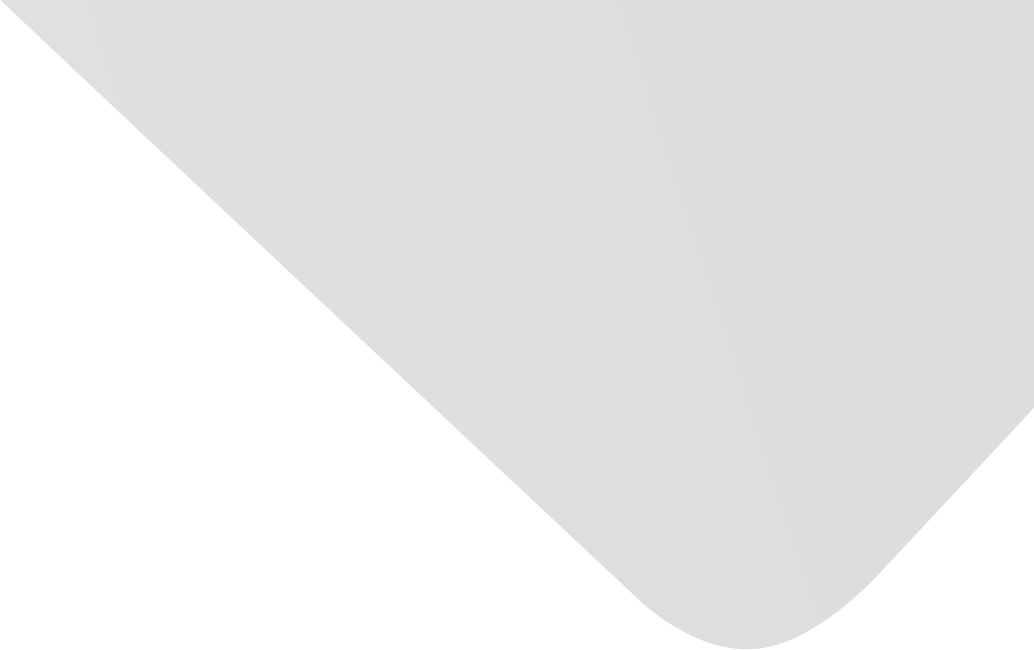
Forecasting of Short-Term Metro Ridership with Support Vector Machine Online Model
Joint Authors
Zhang, Yunlong
Wang, Xuemei
Zhang, Ning
Shi, Zhuangbin
Source
Journal of Advanced Transportation
Issue
Vol. 2018, Issue 2018 (31 Dec. 2018), pp.1-13, 13 p.
Publisher
Hindawi Publishing Corporation
Publication Date
2018-06-27
Country of Publication
Egypt
No. of Pages
13
Main Subjects
Abstract EN
Forecasting for short-term ridership is the foundation of metro operation and management.
A prediction model is necessary to seize the weekly periodicity and nonlinearity characteristics of short-term ridership in real-time.
First, this research captures the inherent periodicity of ridership via seasonal autoregressive integrated moving average model (SARIMA) and proposes a support vector machine overall online model (SVMOOL) which insets the weekly periodic characteristics and trains the updated data day by day.
Then, this research captures the nonlinear characteristics of the ridership via successive ridership value inputs and proposes a support vector machine partial online model (SVMPOL) which insets the nonlinear characteristics and trains the updated data of the predicted day by time interval (such as 5-min).
Afterwards, to avoid the drawbacks and to take advantages of the strengths of the two individual online models, this research takes the average predicted values of two models as the final predicted values, which are called support vector machine combined online model (SVMCOL).
Finally, this research uses the 5-min ridership at Zhujianglu and Sanshanjie Stations of Nanjing Metro to compare the SVMCOL model with three well-known prediction models including SARIMA, back-propagation neural network (BPNN), and SVM models.
The resultant performance comparisons suggest that SARIMA is superior for the stable weekday ridership to other models.
Yet the SVMCOL model is the best performer for the unstable weekend ridership and holiday ridership.
It shows that for metro operation manager that gear toward timely response to real-world unstable and abnormal situations, the SVMCOL may be a better tool than the three well-known models.
American Psychological Association (APA)
Wang, Xuemei& Zhang, Ning& Zhang, Yunlong& Shi, Zhuangbin. 2018. Forecasting of Short-Term Metro Ridership with Support Vector Machine Online Model. Journal of Advanced Transportation،Vol. 2018, no. 2018, pp.1-13.
https://search.emarefa.net/detail/BIM-1181129
Modern Language Association (MLA)
Wang, Xuemei…[et al.]. Forecasting of Short-Term Metro Ridership with Support Vector Machine Online Model. Journal of Advanced Transportation No. 2018 (2018), pp.1-13.
https://search.emarefa.net/detail/BIM-1181129
American Medical Association (AMA)
Wang, Xuemei& Zhang, Ning& Zhang, Yunlong& Shi, Zhuangbin. Forecasting of Short-Term Metro Ridership with Support Vector Machine Online Model. Journal of Advanced Transportation. 2018. Vol. 2018, no. 2018, pp.1-13.
https://search.emarefa.net/detail/BIM-1181129
Data Type
Journal Articles
Language
English
Notes
Includes bibliographical references
Record ID
BIM-1181129