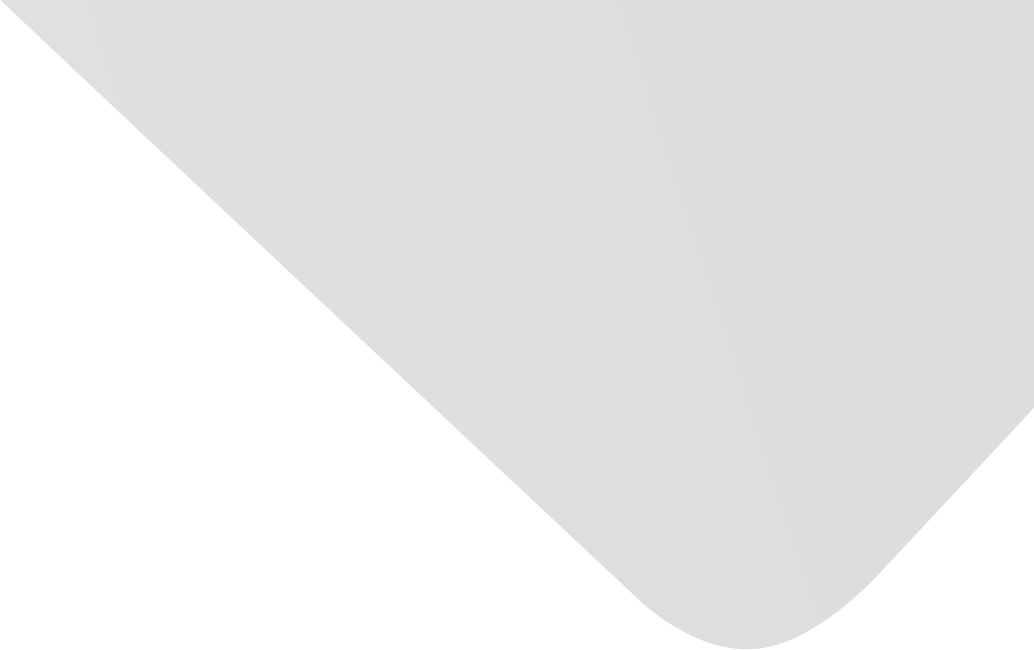
An Improved Deep Learning Model for Traffic Crash Prediction
Joint Authors
Dong, Chunjiao
Shao, Chunfu
Li, Juan
Xiong, Zhihua
Source
Journal of Advanced Transportation
Issue
Vol. 2018, Issue 2018 (31 Dec. 2018), pp.1-13, 13 p.
Publisher
Hindawi Publishing Corporation
Publication Date
2018-12-10
Country of Publication
Egypt
No. of Pages
13
Main Subjects
Abstract EN
Machine-learning technology powers many aspects of modern society.
Compared to the conventional machine learning techniques that were limited in processing natural data in the raw form, deep learning allows computational models to learn representations of data with multiple levels of abstraction.
In this study, an improved deep learning model is proposed to explore the complex interactions among roadways, traffic, environmental elements, and traffic crashes.
The proposed model includes two modules, an unsupervised feature learning module to identify functional network between the explanatory variables and the feature representations and a supervised fine tuning module to perform traffic crash prediction.
To address the unobserved heterogeneity issues in the traffic crash prediction, a multivariate negative binomial (MVNB) model is embedding into the supervised fine tuning module as a regression layer.
The proposed model was applied to the dataset that was collected from Knox County in Tennessee to validate the performances.
The results indicate that the feature learning module identifies relational information between the explanatory variables and the feature representations, which reduces the dimensionality of the input and preserves the original information.
The proposed model that includes the MVNB regression layer in the supervised fine tuning module can better account for differential distribution patterns in traffic crashes across injury severities and provides superior traffic crash predictions.
The findings suggest that the proposed model is a superior alternative for traffic crash predictions and the average accuracy of the prediction that was measured by RMSD can be improved by 84.58% and 158.27% compared to the deep learning model without the regression layer and the SVM model, respectively.
American Psychological Association (APA)
Dong, Chunjiao& Shao, Chunfu& Li, Juan& Xiong, Zhihua. 2018. An Improved Deep Learning Model for Traffic Crash Prediction. Journal of Advanced Transportation،Vol. 2018, no. 2018, pp.1-13.
https://search.emarefa.net/detail/BIM-1181199
Modern Language Association (MLA)
Dong, Chunjiao…[et al.]. An Improved Deep Learning Model for Traffic Crash Prediction. Journal of Advanced Transportation No. 2018 (2018), pp.1-13.
https://search.emarefa.net/detail/BIM-1181199
American Medical Association (AMA)
Dong, Chunjiao& Shao, Chunfu& Li, Juan& Xiong, Zhihua. An Improved Deep Learning Model for Traffic Crash Prediction. Journal of Advanced Transportation. 2018. Vol. 2018, no. 2018, pp.1-13.
https://search.emarefa.net/detail/BIM-1181199
Data Type
Journal Articles
Language
English
Notes
Includes bibliographical references
Record ID
BIM-1181199